Entity Linking Facing Incomplete Knowledge Base.
WISE(2018)
摘要
Entity linking, bridging text and knowledge base, is a fundamental task in the field of information extraction. Most existing approaches highly depend on the structural features and statistics in the target knowledge base. Compared with raw text, they provide more discriminative information and make the task easier. However, in many closed domains, structural features and statistics are rarely available and the target knowledge base may be as simple and sparse as a series of separate entity records only with description. Therefore, few algorithms could work well on the incomplete knowledge base. In this paper, we propose a novel neural approach which only requires minimal text information from the knowledge base. To extract features from text effectively, we employ the co-attention mechanism to emphasize discriminative words and weaken noise. Compared with existing “black box” neural approaches, co-attention mechanism also brings better interpretability to our model. We conduct experiments on the AIDA-CoNLL benchmark and evaluate the performance with accuracy. Results show that our model achieves 82.3% in accuracy and outperforms the baseline by 1.1%.
更多查看译文
关键词
Entity linking, Co-attention mechanism, Neural network
AI 理解论文
溯源树
样例
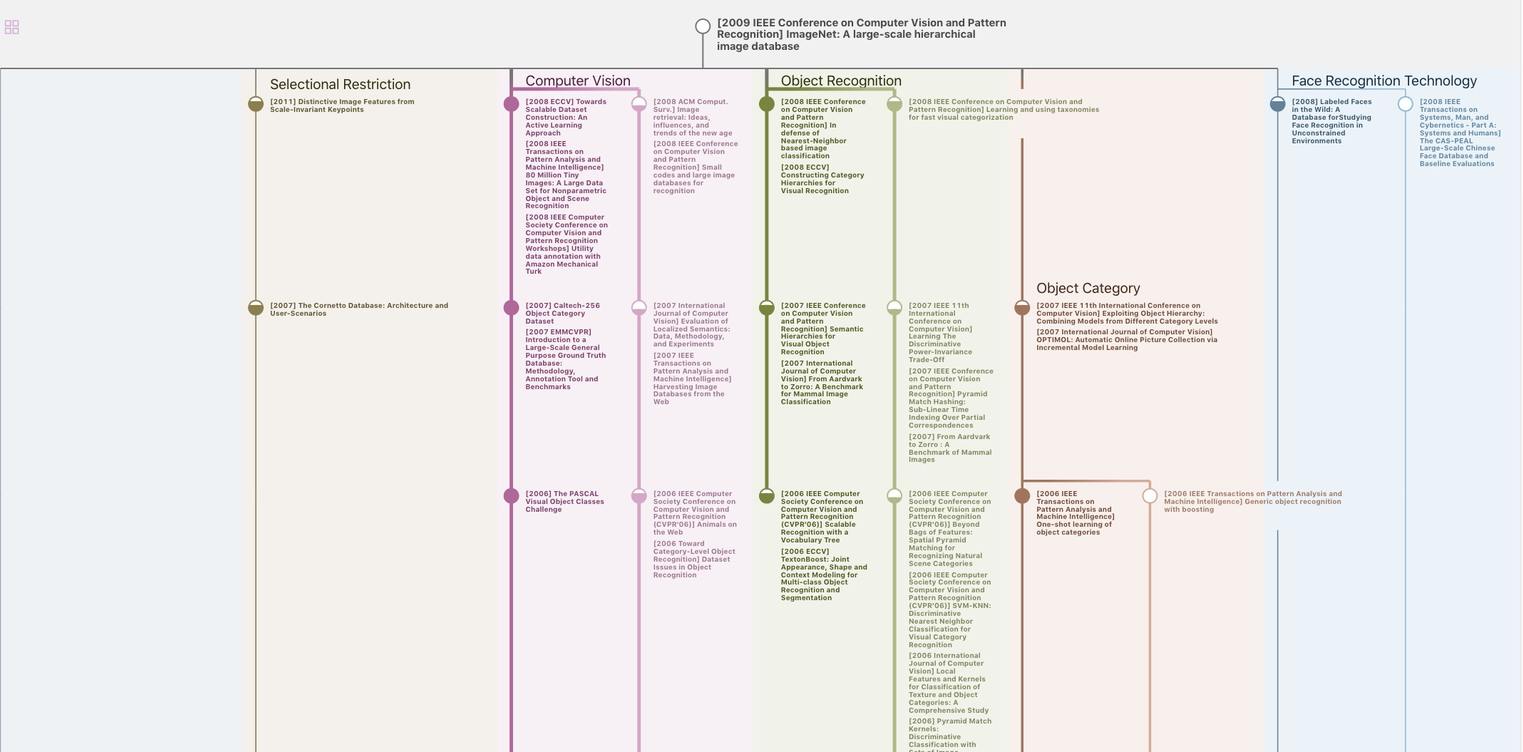
生成溯源树,研究论文发展脉络
Chat Paper
正在生成论文摘要