An efficient encoder-decoder CNN architecture for reliable multilane detection in real time.
Intelligent Vehicles Symposium(2018)
摘要
Multilane detection system is a vital prerequisite for realizing higher ADAS functionality of autonomous navigation. In this work, we present an efficient convolutional neural network (CNN) architecture for real time detection of multiple lane boundaries using a camera sensor. Our network has a simple encoder-decoder architecture and is a special two class semantic segmentation network designed to segment lane boundaries. Efficacy of our network stems from two key insights which are at the foundation of all our design decisions. Firstly, we term a lane boundary as a weak class object in the context of semantic segmentation. We show that the weak class objects which occupy relatively few pixels in the scene, also have a relatively low detection accuracy among the know segmentation methods. We present novel design choices and intuitions to improve the segmentation accuracy of weak class objects, which in turn reduces computation time. Our second insight lies in the manner we depict the ground truth information in our derived dataset. Instead of annotating just the visible lane markers, we accurately delineate the lane boundaries in the ground truth for challenging scenarios like occlusions, low light and degraded lane markings. We then leverage the CNNu0027s ability to concisely summarize the global and local context in an image, for accurately inferring lane boundaries in these challenging cases. We evaluate our network against ENet and FCN-8, and found it performing notably better in terms of speed and accuracy. Our network achieves an encouraging 46 FPS performance on NVIDIA Drive PX2 platform and it has been validated on our test vehicle in highway driving conditions.
更多查看译文
关键词
multilane detection system,autonomous navigation,time detection,multiple lane boundaries,segment lane boundaries,key insights,lane boundary,segmentation methods,segmentation accuracy,lane markings,encoder-decoder architecture,CNN,encoder-decoder CNN architecture,multilane detection,convolutional neural network architecture,ADAS functionality,two class semantic segmentation network,ENet
AI 理解论文
溯源树
样例
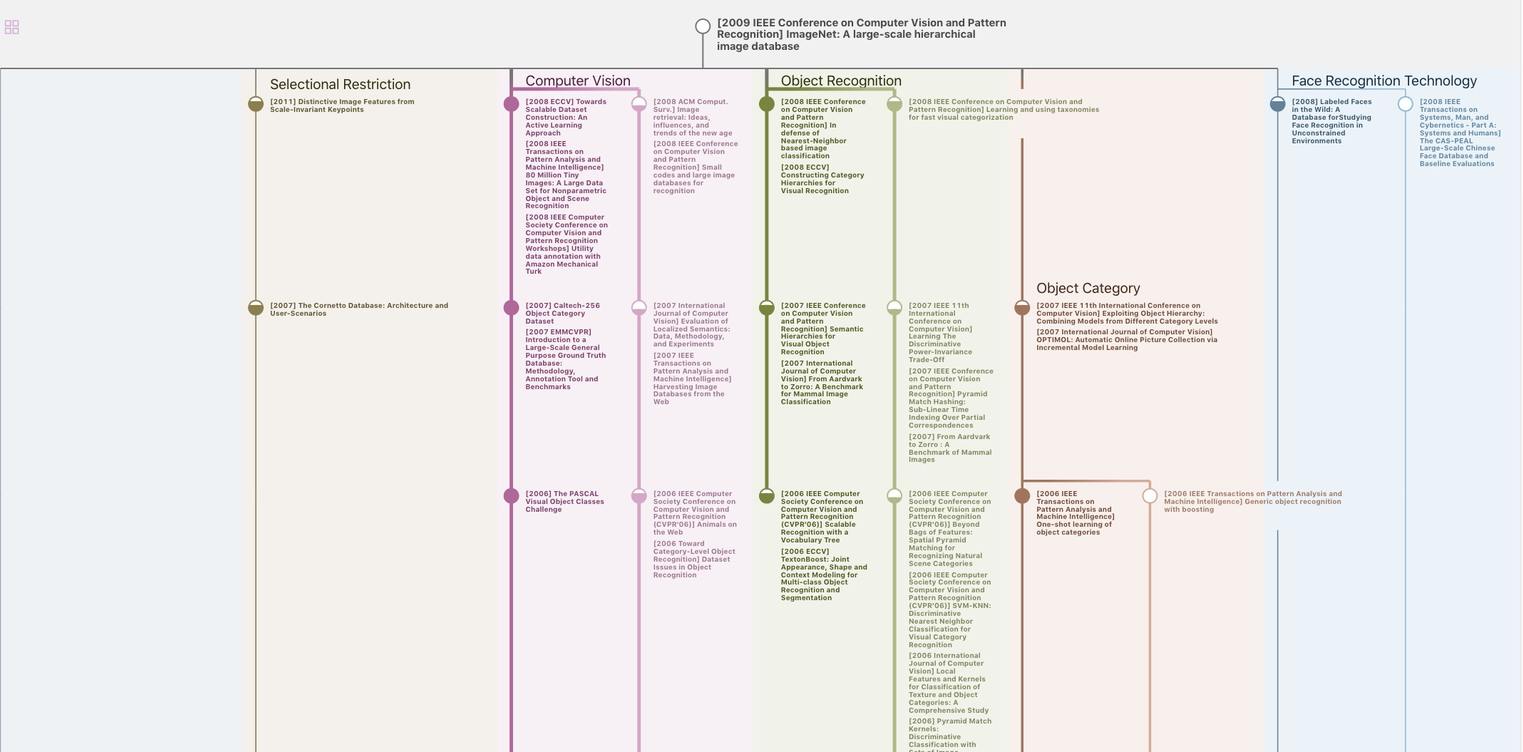
生成溯源树,研究论文发展脉络
Chat Paper
正在生成论文摘要