End-to-End Compromised Account Detection.
ASONAM '18: International Conference on Advances in Social Networks Analysis and Mining Barcelona Spain August, 2018(2018)
摘要
Social media, e.g. Twitter, has become a widely used medium for the exchange of information, but it has also become a valuable tool for hackers to spread misinformation through compromised accounts. Hence, detecting compromised accounts is a necessary step toward a safe and secure social media environment. Nevertheless, detecting compromised accounts faces several challenges. First, social media activities of users are temporally correlated which plays an important role in compromised account detection. Second, data associated with social media accounts is inherently sparse. Finally, social contagions where multiple accounts become compromised, take advantage of the user connectivity to propagate their attack. Thus how to represent each user's network features for compromised account detection is an additional challenge. To address these challenges, we propose an End-to-End Compromised Account Detection framework (E2ECAD). E2ECAD effectively captures temporal correlations via an LSTM (Long Short-Term Memory) network. Further, it addresses the sparsity problem by defining and employing a user context representation. Meanwhile, informative network-related features are modeled efficiently. To verify the working of the framework, we construct a real-world dataset of compromised accounts on Twitter and conduct extensive experiments. The results of experiments show that E2ECAD outperforms the state of the art compromised account detection algorithms.
更多查看译文
关键词
safe media environment,secure social media environment,social media accounts,compromised account detection algorithms,end-to-end compromised account detection
AI 理解论文
溯源树
样例
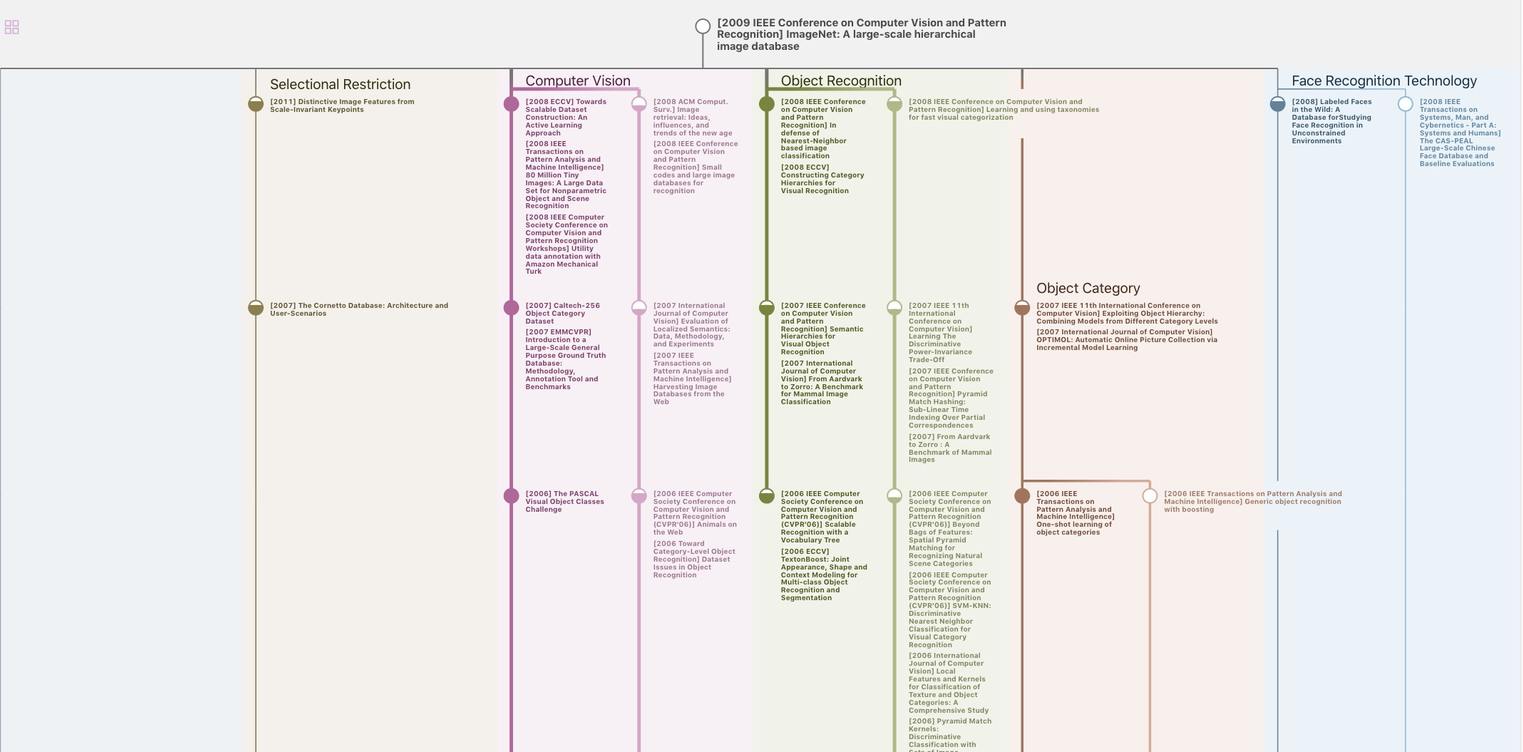
生成溯源树,研究论文发展脉络
Chat Paper
正在生成论文摘要