Nowcasting Air Quality by Fusing Insights from Meteorological Data, Satellite Imagery and Social Media Images Using Deep Learning
2018 IEEE/ACM INTERNATIONAL CONFERENCE ON ADVANCES IN SOCIAL NETWORKS ANALYSIS AND MINING (ASONAM)(2018)
摘要
Peatland fire and haze events in Southeast Asia are disasters with trans-boundary implications, having increased in recent years along with rapid deforestation, land clearing and severe dry seasons. Aerosols are emitted in high concentrations from the fires, which degrade air quality and reduce visibility, in turn causing economic, social, health, and environmental problems. During haze events, it is critical for public authorities to have timely information about affected populations. Currently, Indonesian disaster management authorities manage forest and peatland fire and haze events based on satellite data and sensors. They are looking for more real-time information in order to better protect vulnerable populations and environment. This paper explores information on visibility extracted from photos shared on social media to improve forecasting performance for haze severity. Our results show that visibility information can improve forecast accuracy over a baseline approach with common features, namely data from satellites and ground air quality sensors. Furthermore, by using social media photos, our model adds a near real-time property to the forecast model, with potential to improve disaster management and mitigation.
更多查看译文
关键词
image mining,deep learning,air quality forecast,haze severity inference
AI 理解论文
溯源树
样例
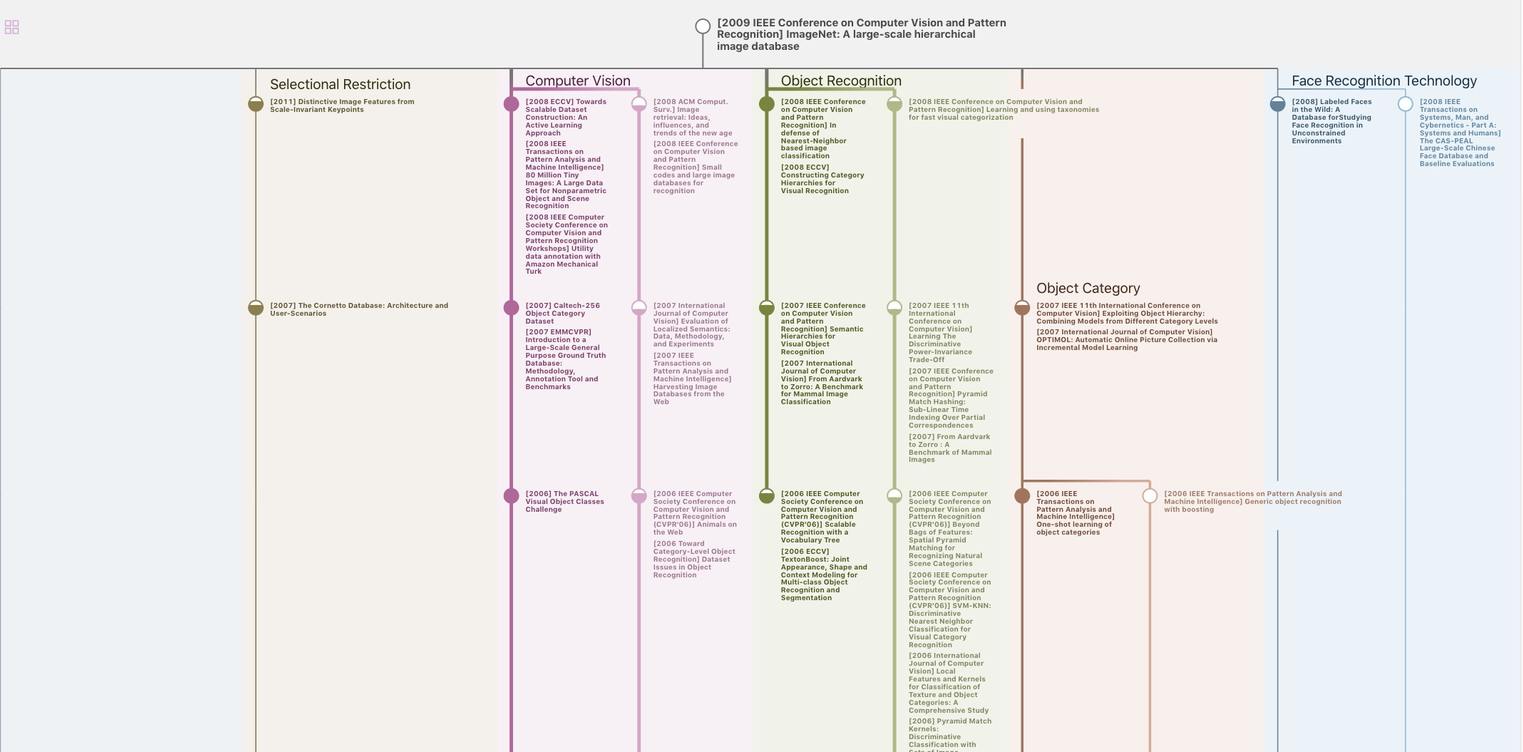
生成溯源树,研究论文发展脉络
Chat Paper
正在生成论文摘要