Dual Fixed-Size Ordinally Forgetting Encoding (FOFE) for Competitive Neural Language Models.
EMNLP(2018)
摘要
In this paper, we propose a new approach to employ the fixed-size ordinally-forgetting encoding (FOFE) (Zhang et al., 2015b) in neural languages modelling, called dual-FOFE. The main idea behind dual-FOFE is that it allows to use two different forgetting factors so that it can avoid the trade-off in choosing either small or large values for the single forgetting factor in the original FOFE. In our experiments, we have compared the dual-FOFE based neural network language models (NNLM) against the original FOFE counterparts and various traditional NNLMs. Our results on the challenging Google Billion Words corpus show that both FOFE and dual FOFE yield very strong performance while significantly reducing the computational complexity over other NNLMs. Furthermore, the proposed dual-FOFE method further gives over 10% relative improvement in perplexity over the original FOFE model.
更多查看译文
AI 理解论文
溯源树
样例
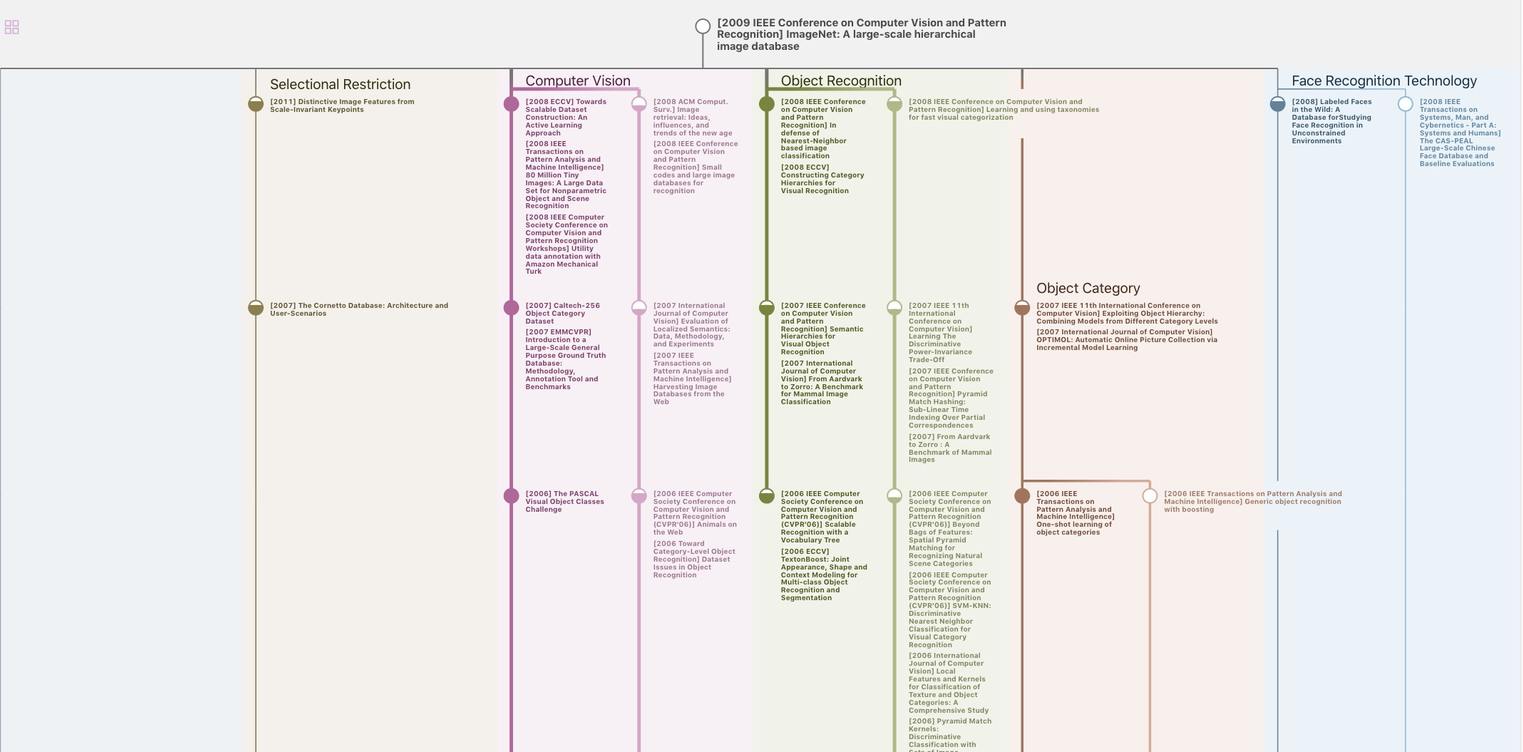
生成溯源树,研究论文发展脉络
Chat Paper
正在生成论文摘要