YouTube-VOS: Sequence-to-Sequence Video Object Segmentation
COMPUTER VISION - ECCV 2018, PT V(2018)
摘要
Learning long-term spatial-temporal features are critical for many video analysis tasks. However, existing video segmentation methods predominantly rely on static image segmentation techniques, and methods capturing temporal dependency for segmentation have to depend on pretrained optical flow models, leading to suboptimal solutions for the problem. End-to-end sequential learning to explore spatial-temporal features for video segmentation is largely limited by the scale of available video segmentation datasets, i.e., even the largest video segmentation dataset only contains 90 short video clips. To solve this problem, we build a new large-scale video object segmentation dataset called YouTube Video Object Segmentation dataset (YouTube-VOS). Our dataset contains 3,252 YouTube video clips and 78 categories including common objects and human activities. This is by far the largest video object segmentation dataset to our knowledge and we have released it at https://youtube-vos.org. Based on this dataset, we propose a novel sequence-to-sequence network to fully exploit long-term spatial-temporal information in videos for segmentation. We demonstrate that our method is able to achieve the best results on our YouTube-VOS test set and comparable results on DAVIS 2016 compared to the current state-of-the-art methods. Experiments show that the large scale dataset is indeed a key factor to the success of our model.
更多查看译文
关键词
Video object segmentation, Large-scale dataset, Spatial-temporal information
AI 理解论文
溯源树
样例
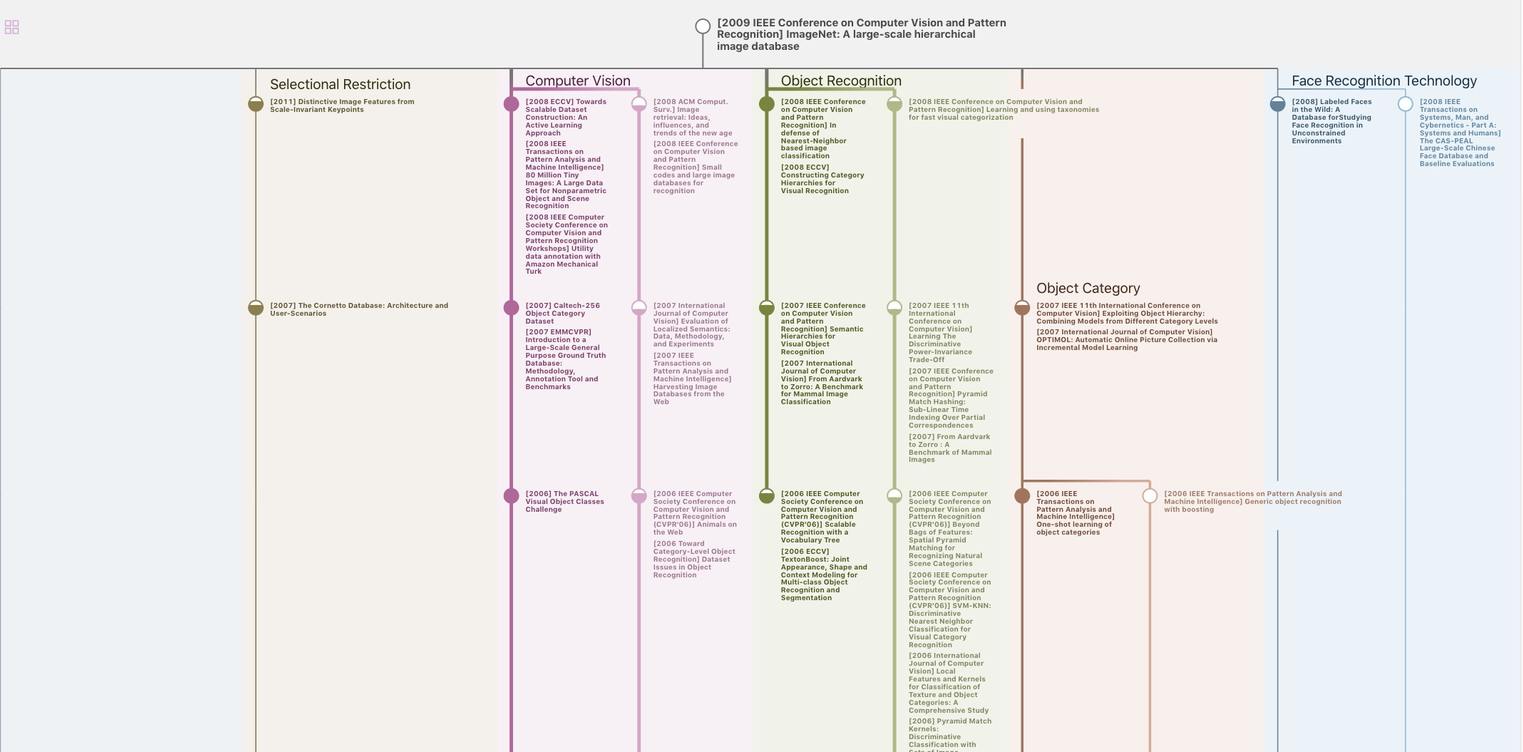
生成溯源树,研究论文发展脉络
Chat Paper
正在生成论文摘要