Estimating the Success of Unsupervised Image to Image Translation
COMPUTER VISION - ECCV 2018, PT V(2018)
摘要
While in supervised learning, the validation error is an unbiased estimator of the generalization (test) error and complexity-based generalization bounds are abundant, no such bounds exist for learning a mapping in an unsupervised way. As a result, when training GANs and specifically when using GANs for learning to map between domains in a completely unsupervised way, one is forced to select the hyperparameters and the stopping epoch by subjectively examining multiple options. We propose a novel bound for predicting the success of unsupervised cross domain mapping methods, which is motivated by the recently proposed Simplicity Principle. The bound can be applied both in expectation, for comparing hyperparameters and for selecting a stopping criterion, or per sample, in order to predict the success of a specific cross-domain translation. The utility of the bound is demonstrated in an extensive set of experiments employing multiple recent algorithms. Our code is available at https://github.com/sagiebenaim/gan_bound .
更多查看译文
关键词
Unsupervised learning, Generalization bounds, Image to image translation, GANs
AI 理解论文
溯源树
样例
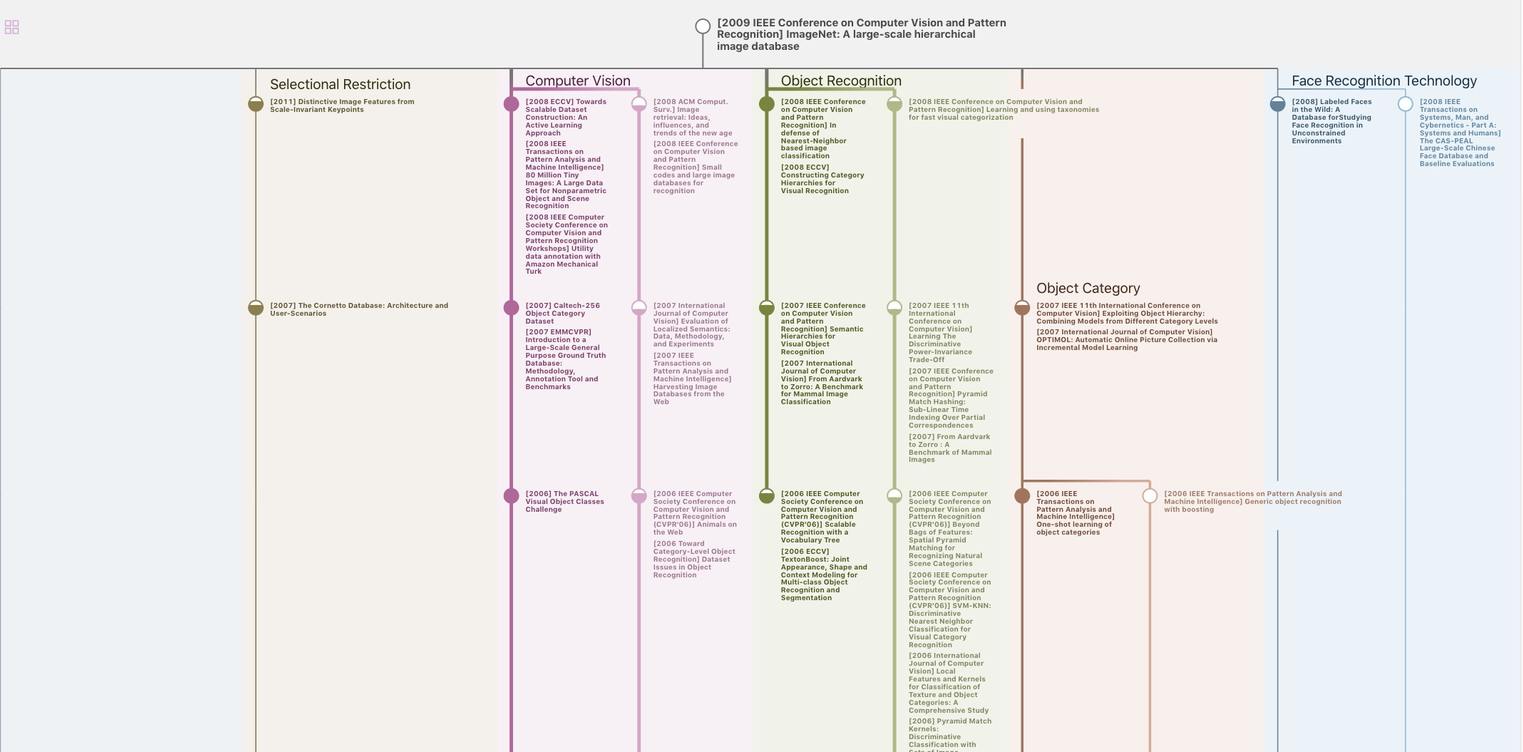
生成溯源树,研究论文发展脉络
Chat Paper
正在生成论文摘要