Learning 3d Keypoint Descriptors For Non-Rigid Shape Matching
COMPUTER VISION - ECCV 2018, PT VIII(2018)
摘要
In this paper, we present a novel deep learning framework that derives discriminative local descriptors for 3D surface shapes. In contrast to previous convolutional neural networks (CNNs) that rely on rendering multi-view images or extracting intrinsic shape properties, we parameterize the multi-scale localized neighborhoods of a keypoint into regular 2D grids, which are termed as 'geometry images'. The benefits of such geometry images include retaining sufficient geometric information, as well as allowing the usage of standard CNNs. Specifically, we leverage a triplet network to perform deep metric learning, which takes a set of triplets as input, and a newly designed triplet loss function is minimized to distinguish between similar and dissimilar pairs of keypoints. At the testing stage, given a geometry image of a point of interest, our network outputs a discriminative local descriptor for it. Experimental results for non-rigid shape matching on several benchmarks demonstrate the superior performance of our learned descriptors over traditional descriptors and the state-of-the-art learning-based alternatives.
更多查看译文
关键词
Local feature descriptor, Triplet CNNs, Non-rigid shapes
AI 理解论文
溯源树
样例
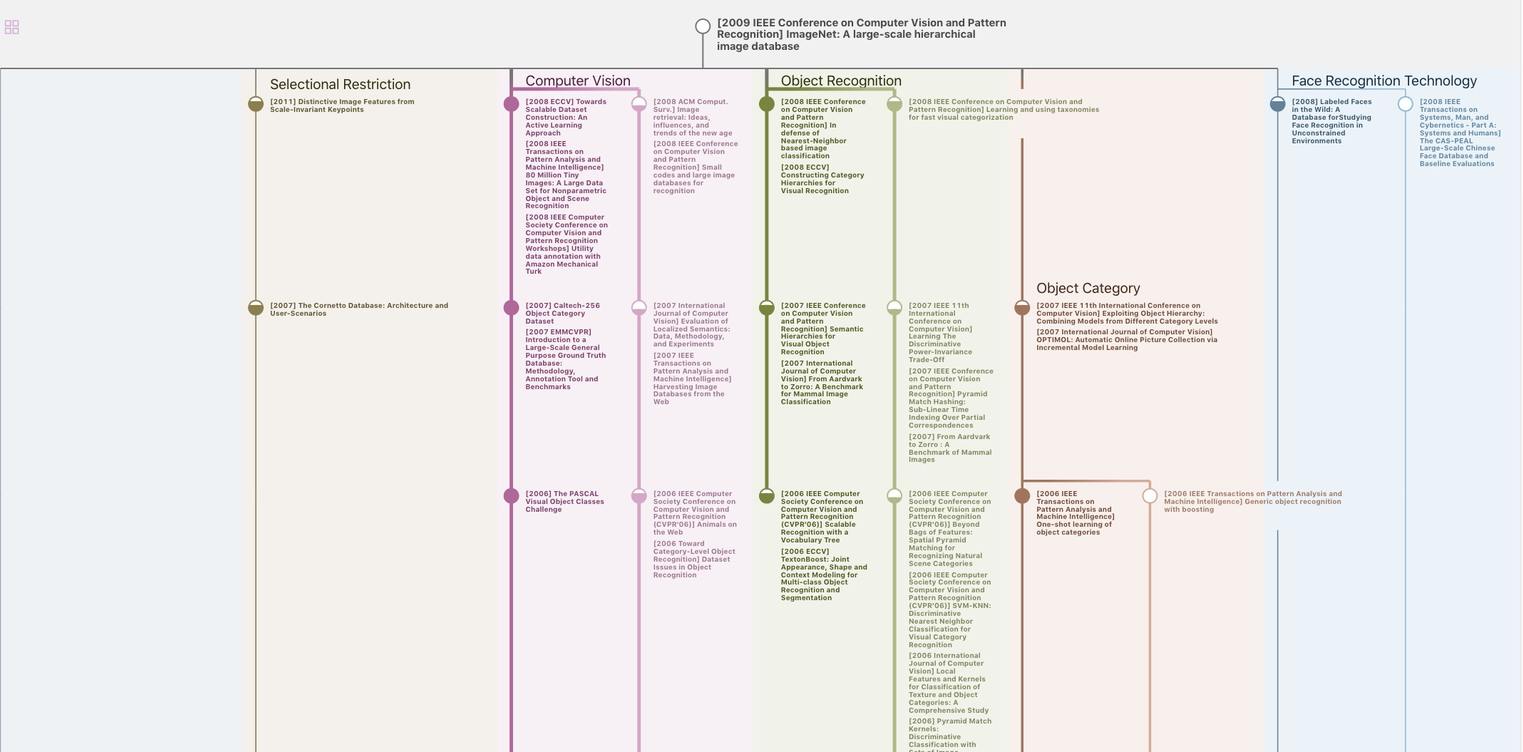
生成溯源树,研究论文发展脉络
Chat Paper
正在生成论文摘要