Learning a Hierarchical Latent-Variable Model of 3D Shapes
2018 International Conference on 3D Vision (3DV)(2018)
摘要
We propose the Variational Shape Learner (VSL), a generative model that learns the underlying structure of voxelized 3D shapes in an unsupervised fashion. Through the use of skip-connections, our model can successfully learn and infer a latent, hierarchical representation of objects. Furthermore, realistic 3D objects can be easily generated by sampling the VSL's latent probabilistic manifold. We show that our generative model can be trained end-to-end from 2D images to perform single image 3D model retrieval. Experiments show, both quantitatively and qualitatively, the improved generalization of our proposed model over a range of tasks, performing better or comparable to various state-of-the-art alternatives.
更多查看译文
关键词
3D shape learning,variational inference,generative models,image reconstruction
AI 理解论文
溯源树
样例
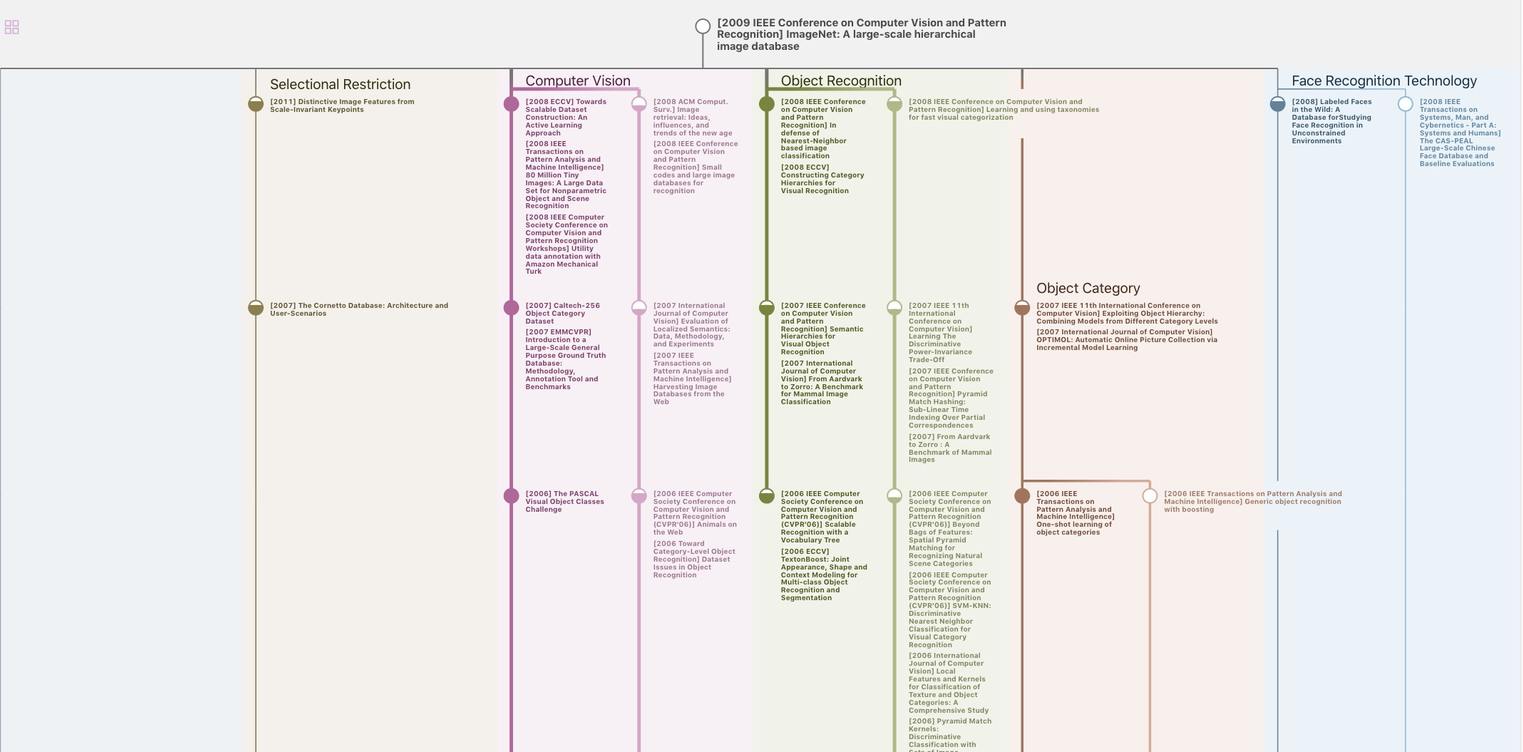
生成溯源树,研究论文发展脉络
Chat Paper
正在生成论文摘要