Calibration: A Simple Way to Improve Click Models.
CIKM(2018)
摘要
We show that click models trained with suboptimal hyperparameters suffer from the issue of bad calibration. This means that their predicted click probabilities do not agree with the observed proportions of clicks in the held-out data. To repair this discrepancy, we adapt a non-parametric calibration method called isotonic regression. Our experimental results show that isotonic regression significantly improves click models trained with suboptimal hyperparameters in terms of perplexity, and that it makes click models less sensitive to the choice of hyperparameters. Interestingly, the relative ranking of existing click models in terms of their predictive performance changes depending on whether or not their predictions are calibrated. Therefore, we advocate that calibration becomes a mandatory part of the click model evaluation protocol.
更多查看译文
关键词
Click models, Calibration
AI 理解论文
溯源树
样例
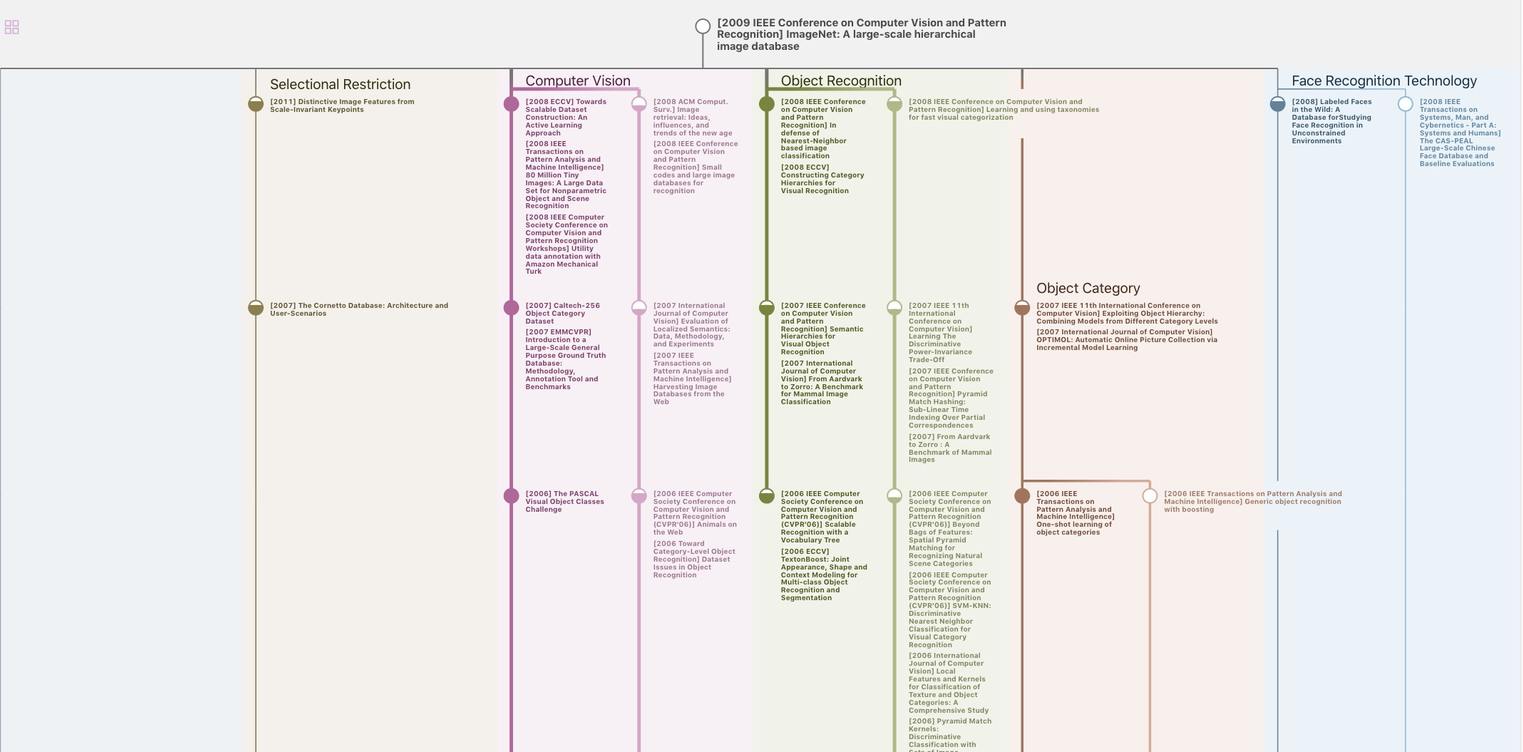
生成溯源树,研究论文发展脉络
Chat Paper
正在生成论文摘要