REGAL: Representation Learning-based Graph Alignment.
CIKM(2018)
摘要
Problems involving multiple networks are prevalent in many scientific and other domains. In particular, network alignment, or the task of identifying corresponding nodes in different networks, has applications across the social and natural sciences. Motivated by recent advancements in node representation learning for single-graph tasks, we propose REGAL (REpresentation learning-based Graph ALignment), a framework that leverages the power of automatically-learned node representations to match nodes across different graphs. Within REGAL we devise xNetMF, an elegant and principled node embedding formulation that uniquely generalizes to multi-network problems. Our results demonstrate the utility and promise of unsupervised representation learning-based network alignment in terms of both speed and accuracy. REGAL runs up to 30x faster in the representation learning stage than comparable methods, outperforms existing network alignment methods by 20 to 30% accuracy on average, and scales to networks with millions of nodes each.
更多查看译文
关键词
graph mining, network alignment, graph matching, node representation learning, node embedding
AI 理解论文
溯源树
样例
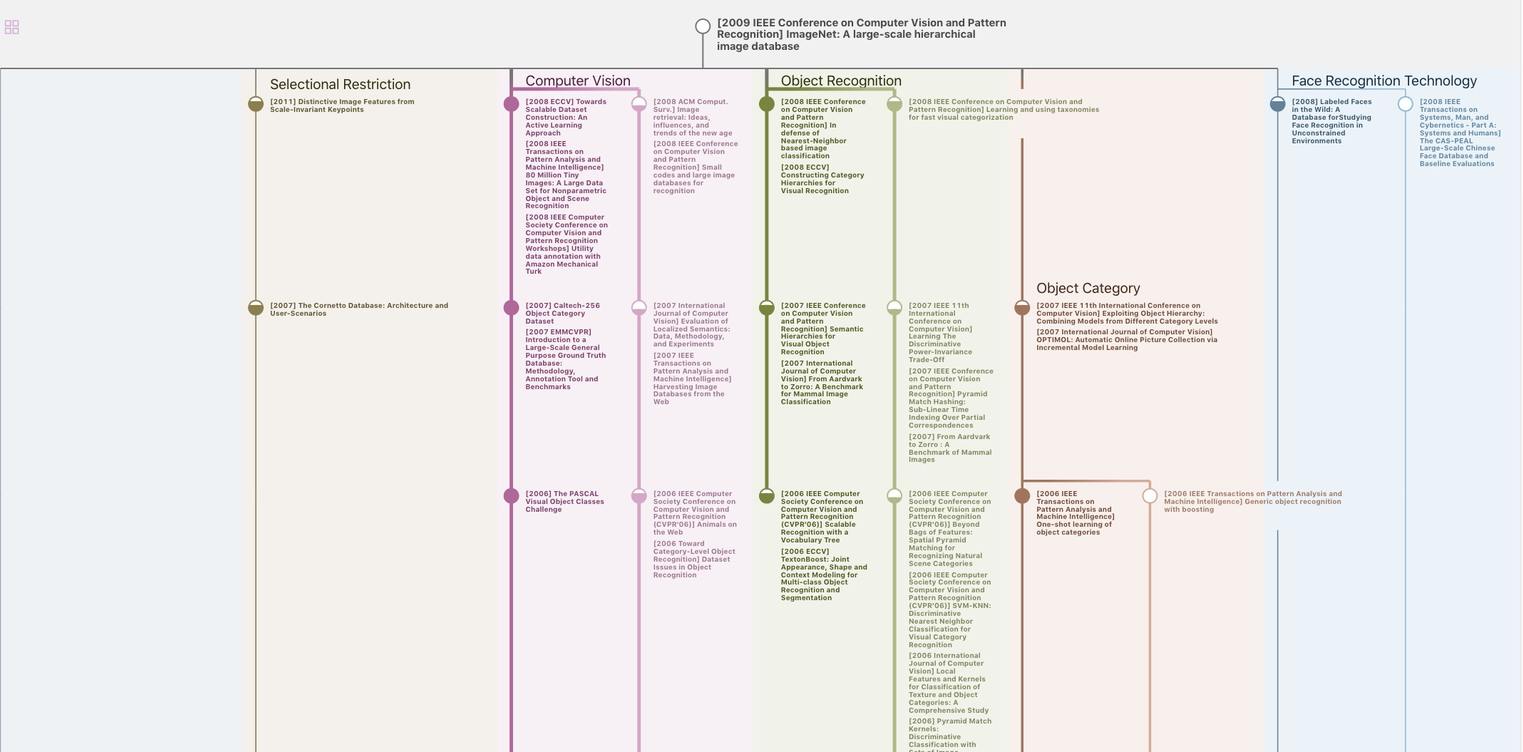
生成溯源树,研究论文发展脉络
Chat Paper
正在生成论文摘要