When Rank Order Isn't Enough: New Statistical-Significance-Aware Correlation Measures.
CIKM(2018)
摘要
Because it is expensive to construct test collections for Cranfield-based evaluation of information retrieval systems, a variety of lower-cost methods have been proposed. The reliability of these methods is often validated by measuring rank correlation (e.g., Kendall's tau) between known system rankings on the full test collection vs. observed system rankings on the lower-cost one. However, existing rank correlation measures do not consider the statistical significance of score differences between systems in the observed rankings. To address this, we propose two statistical-significance-aware rank correlation measures, one of which is a head-weighted version of the other. We first show empirical differences between our proposed measures and existing ones. We then compare the measures while benchmarking four system evaluation methods: pooling, crowdsourcing, evaluation with incomplete judgments, and automatic system ranking. We show that use of our measures can lead to different experimental conclusions regarding reliability of alternative low-cost evaluation methods.
更多查看译文
关键词
Rank Correlation, Evaluation, IR System Ranking
AI 理解论文
溯源树
样例
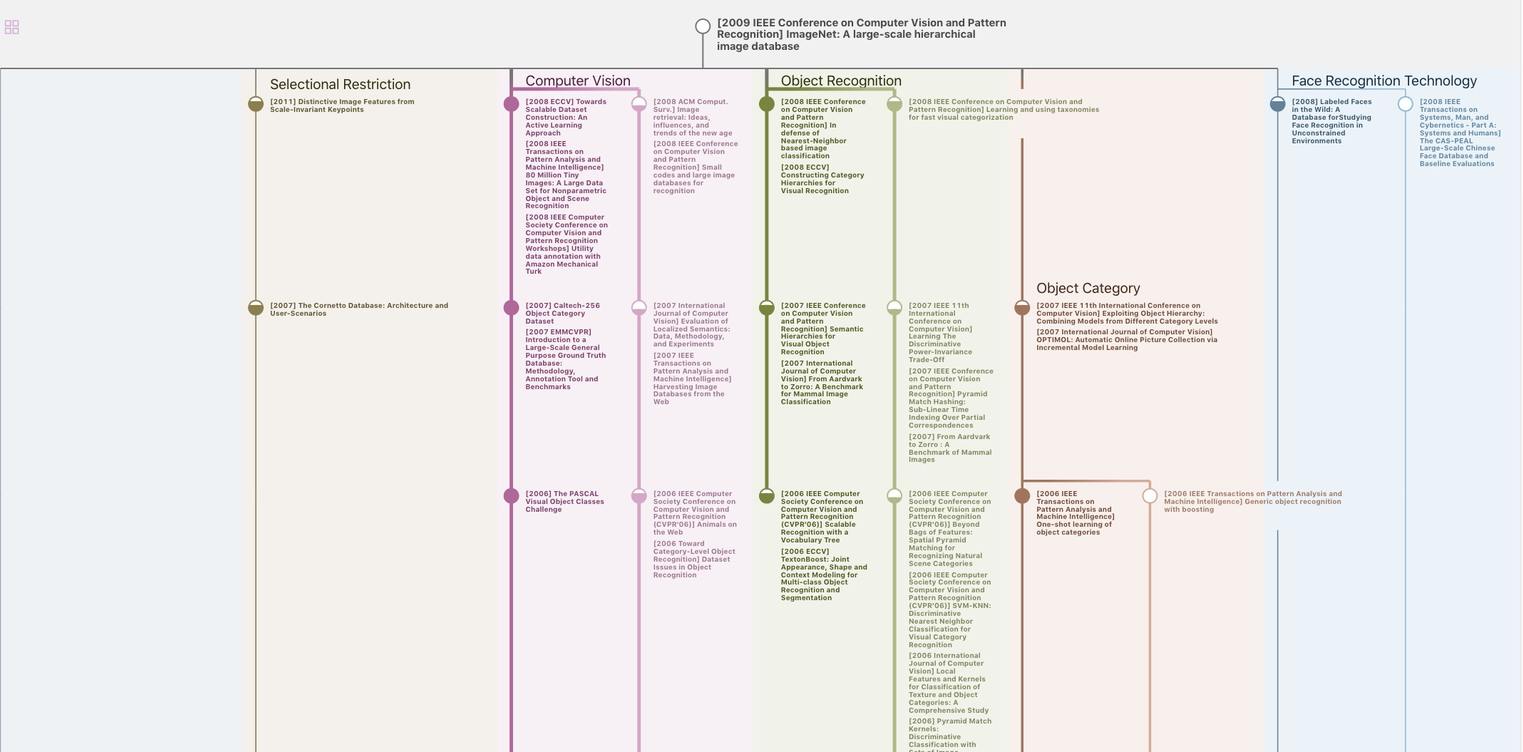
生成溯源树,研究论文发展脉络
Chat Paper
正在生成论文摘要