Global Attention for Name Tagging.
CoNLL(2018)
摘要
Many name tagging approaches use local contextual information with much success, but fail when the local context is ambiguous or limited. We present a new framework to improve name tagging by utilizing local, document-level, and corpus-level contextual information. We retrieve document-level context from other sentences within the same document and corpus-level context from sentences in other topically related documents. We propose a model that learns to incorporate document-level and corpus-level contextual information alongside local contextual information via global attentions, which dynamically weight their respective contextual information, and gating mechanisms, which determine the influence of this information. Extensive experiments on benchmark datasets show the effectiveness of our approach, which achieves state-of-the-art results for Dutch, German, and Spanish on the CoNLL-2002 and CoNLL-2003 datasets.
更多查看译文
关键词
name tagging,attention
AI 理解论文
溯源树
样例
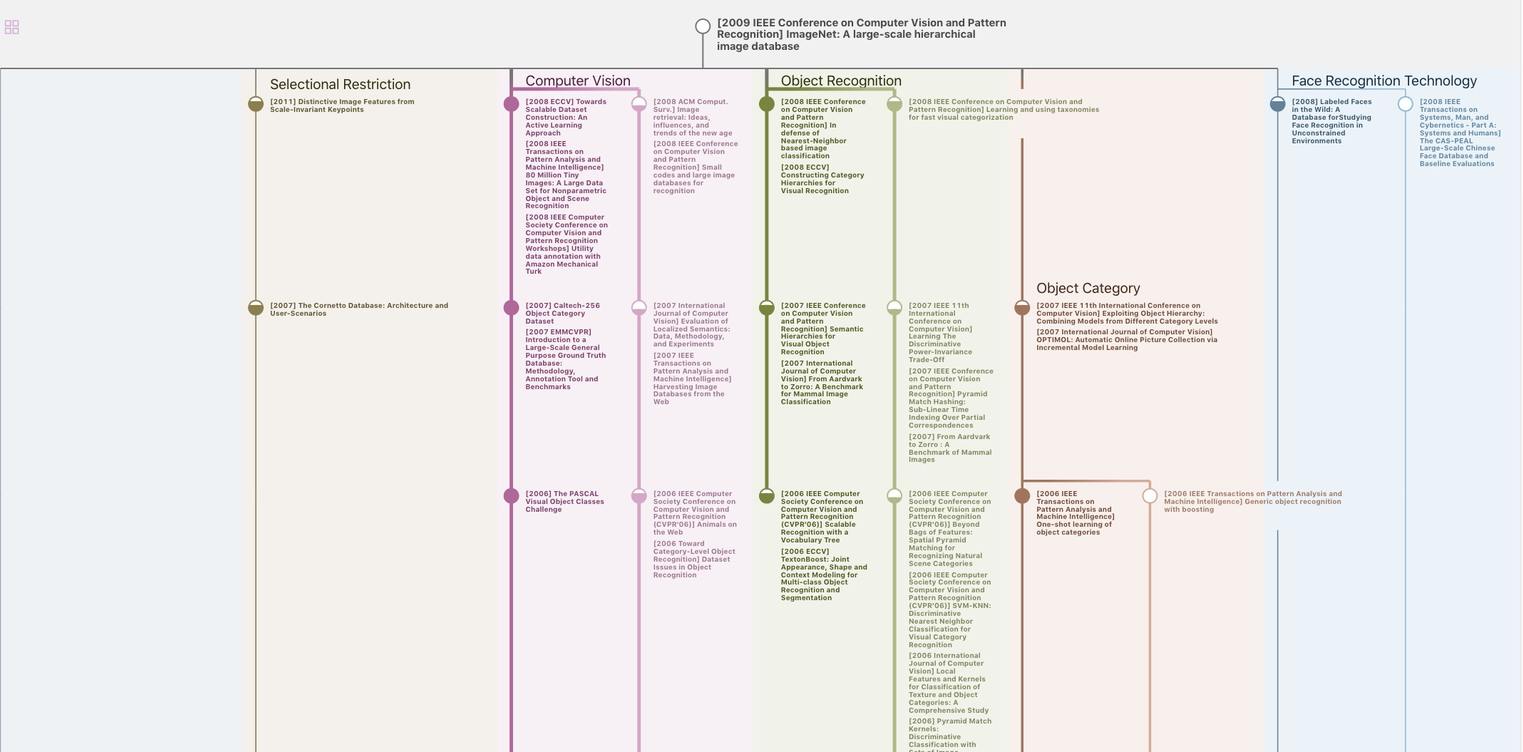
生成溯源树,研究论文发展脉络
Chat Paper
正在生成论文摘要