Realtime Traffic Speed Estimation with Sparse Crowdsourced Data
2018 IEEE 34th International Conference on Data Engineering (ICDE)(2018)
摘要
Realtime traffic speed estimation is an important issue in urban computation. Existing approaches usually focus on exploiting the periodicity properties of the traffic speed and utilize crowdsourcing techniques to facilitate real-time estimation. The quality of such estimation is limited in real world: 1) the accuracy of existing estimation over-relies on the probed data; 2) the accidental traffic variance is ignored; 3) existing strategies incur exhaustive usage of human workers to get fine-grained estimation results. Thus, a more intelligent RTSE approach is desired. In this paper, we propose the framework of CrowdRTSE (Crowdsourcing-based Real-time Traffic Speed Estimation), which adopts a hybrid offline-online process to collaboratively exploit the historical and real-time data to produce high-quality RTSE. To accomplish such a framework, we devise effective algorithms to judiciously select the best group of human workers with a constant approximation ratio, and effectively propagate the crowdsourced data with high efficiency. Comprehensive evaluations have been conducted on both synthetic and real world datasets. The experimental results verify the effectiveness and efficiency of our proposed methods.
更多查看译文
关键词
crowdsourcing,traffic speed,urban computing
AI 理解论文
溯源树
样例
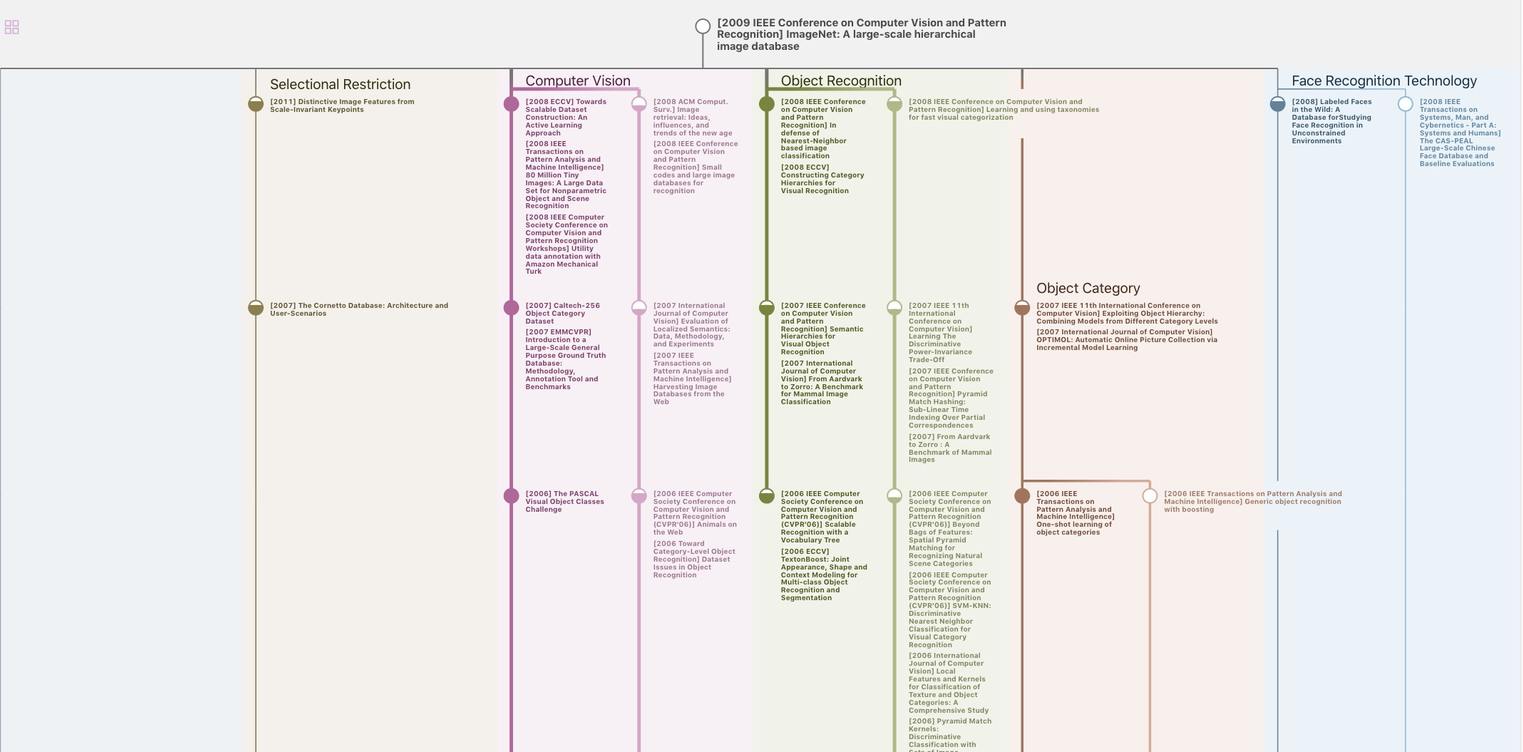
生成溯源树,研究论文发展脉络
Chat Paper
正在生成论文摘要