Attention-Based CNN-BLSTM Networks for Joint Intent Detection and Slot Filling.
CCL(2018)
摘要
Dialogue intent detection and semantic slot filling are two critical tasks in nature language understanding (NLU) for task-oriented dialog systems. In this paper, we present an attention-based encoder-decoder neural network model for joint intent detection and slot filling, which encodes sentence representation with a hybrid Convolutional Neural Networks and Bidirectional Long Short-Term Memory Networks (CNN-BLSTM), and decodes it with an attention-based recurrent neural network with aligned inputs. In the encoding process, our model firstly extracts higher-level phrase representations and local features from each utterance using convolutional neural network, and then propagates historical contextual semantic information with a bidirectional long short-term memory network layer architecture. Accordingly, we could obtain sentence representation by merging the two architectures mentioned above. In the decoding process, we introduce attention mechanism in long short-term memory networks that can provide additional sematic information. We conduct experiment on dialogue intent detection and slot filling tasks with standard data set Airline Travel Information System (ATIS). Experimental results manifest that our proposed model can achieve better overall performance.
更多查看译文
关键词
Nature language understanding, Slot filling, Intent detection, Attention model
AI 理解论文
溯源树
样例
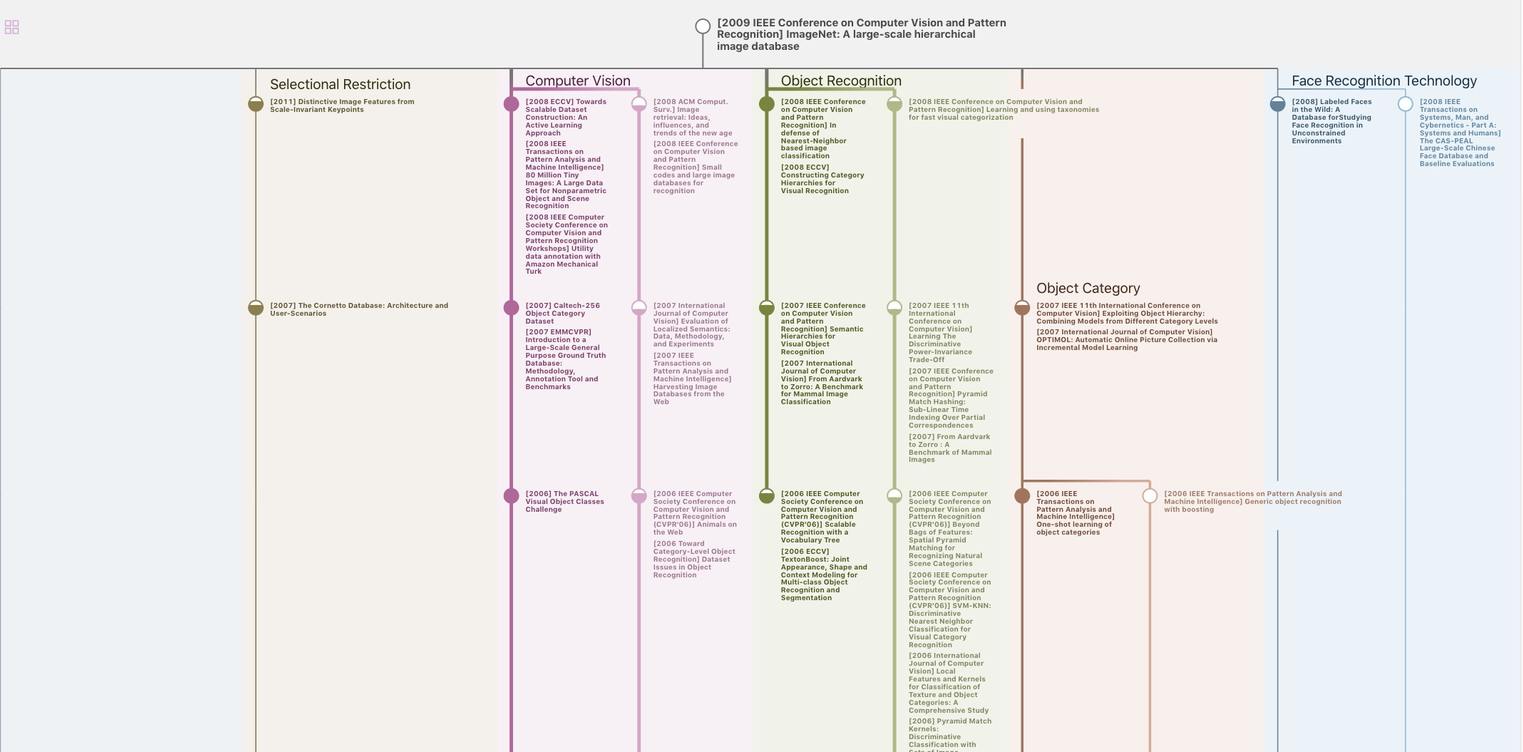
生成溯源树,研究论文发展脉络
Chat Paper
正在生成论文摘要