Low Latency Spiking ConvNets with Restricted Output Training and False Spike Inhibition
2018 International Joint Conference on Neural Networks (IJCNN)(2018)
摘要
Deep convolutional neural networks (ConvNets) have achieved the state-of-the-art performance on many real world applications. However, significant computation and storage demands are required by ConvNets. Spiking neural networks (SNNs), with sparsely activated neurons and event-driven computations, show great potential to take advantage of the ultra low power spike-based hardware architectures. Yet, training SNN with similar accuracy as ConvNets is difficult. Recent researchers have demonstrated the work of converting ConvNets to SNNs (CNN-SNN conversion) with similar accuracy. However, the energy-efficiency of the converted SNNs is impaired by the increased classification latency. In this paper, we focus on optimizing the classification latency of the converted SNNs. First, we propose a restricted output training method to normalize the converted weights dynamically in the CNN-SNN training phase. Second, false spikes are identified and the false spike inhibition theory is derived to speedup the convergence of the classification process. Third, we propose a temporal max pooling method to approximate the max pooling operation in ConvNets without accuracy loss. The evaluation shows that the converted SNNs converge in about 30 time-steps and achieve the best classification accuracy of 94% on CIFAR-10 dataset.
更多查看译文
关键词
Spiking Neural Networks,Convolutional Neural Networks,CNN-SNN Conversion
AI 理解论文
溯源树
样例
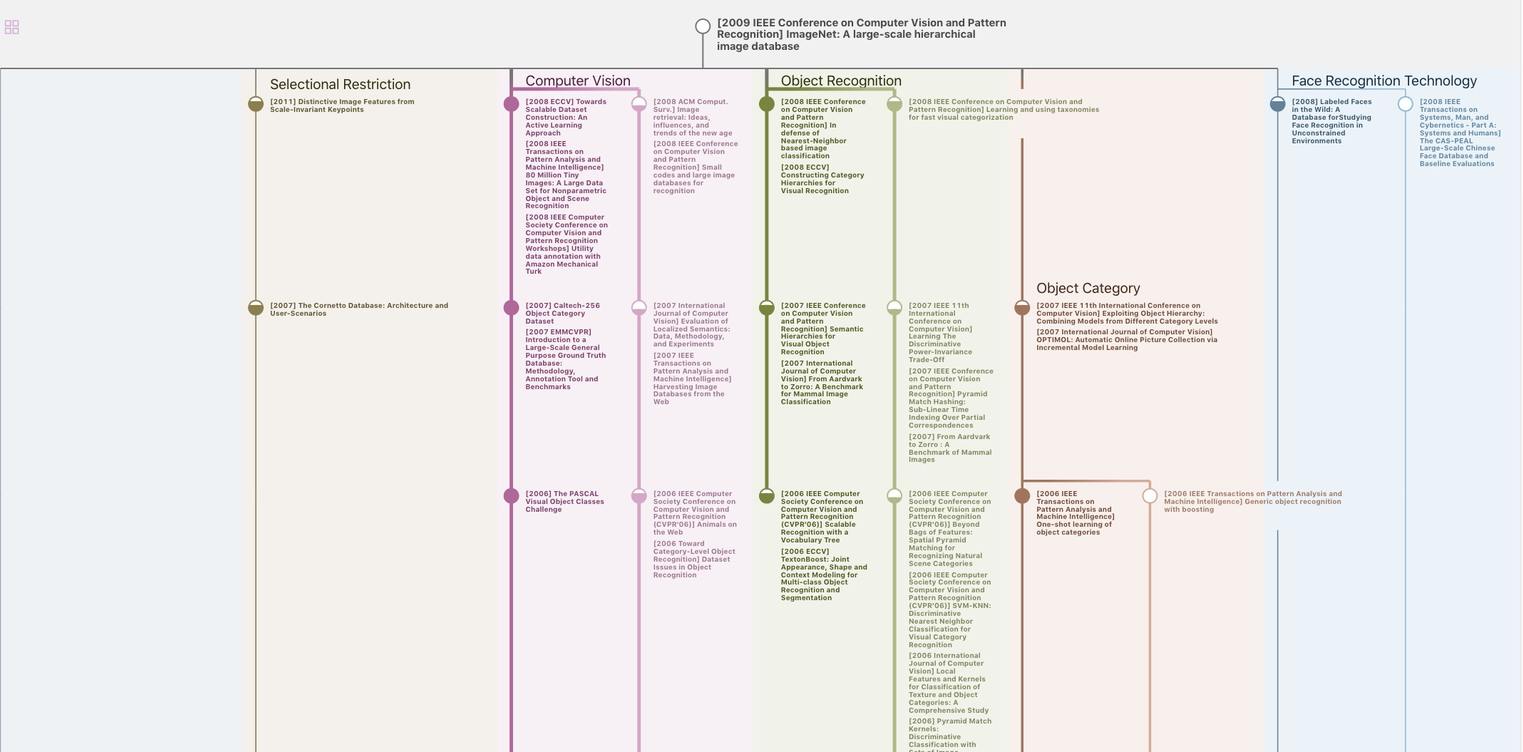
生成溯源树,研究论文发展脉络
Chat Paper
正在生成论文摘要