Investigating Multiobjective Methods In Multitask Classification
2018 INTERNATIONAL JOINT CONFERENCE ON NEURAL NETWORKS (IJCNN)(2018)
摘要
Regularized multitask learning is explicitly interpreted here as a many-objective optimization problem, dealt with a deterministic solver that properly controls the sampling of the Pareto frontier. Each objective function corresponds to the learning loss of a task, so that we have as many objectives as tasks. The obtained Pareto-optimal models are then explored to implement distinct learning sharing strategies: (1) by considering a single parameter vector for all tasks, the simplest learning model that could have been conceived in multitask learning, the distinct trade-offs along the Pareto frontier can be interpreted as efficient and diverse sharing perspectives for the multiple tasks; (2) those distinct sharing perspectives are then aggregated in an ensemble or the best model in the validation set is selected. Notice that using a single parameter vector for all tasks in our many-objective perspective should not be directly associated with that naive, and generally of low performance, procedure of taking all tasks as being equally related. Distinct trade-offs automatically promote the proposition of efficient and structurally diverse relationships among the learning tasks, which support a competitive performance when compared with consolidated multitask learning methods in classification problems.
更多查看译文
关键词
multiobjective methods,multitask classification,regularized multitask learning,many-objective optimization problem,deterministic solver,Pareto frontier,Pareto-optimal models,distinct learning sharing strategies,single parameter vector,simplest learning model,diverse sharing perspectives,multiple tasks,distinct sharing perspectives,many-objective perspective,structurally diverse relationships,learning tasks,multitask learning methods
AI 理解论文
溯源树
样例
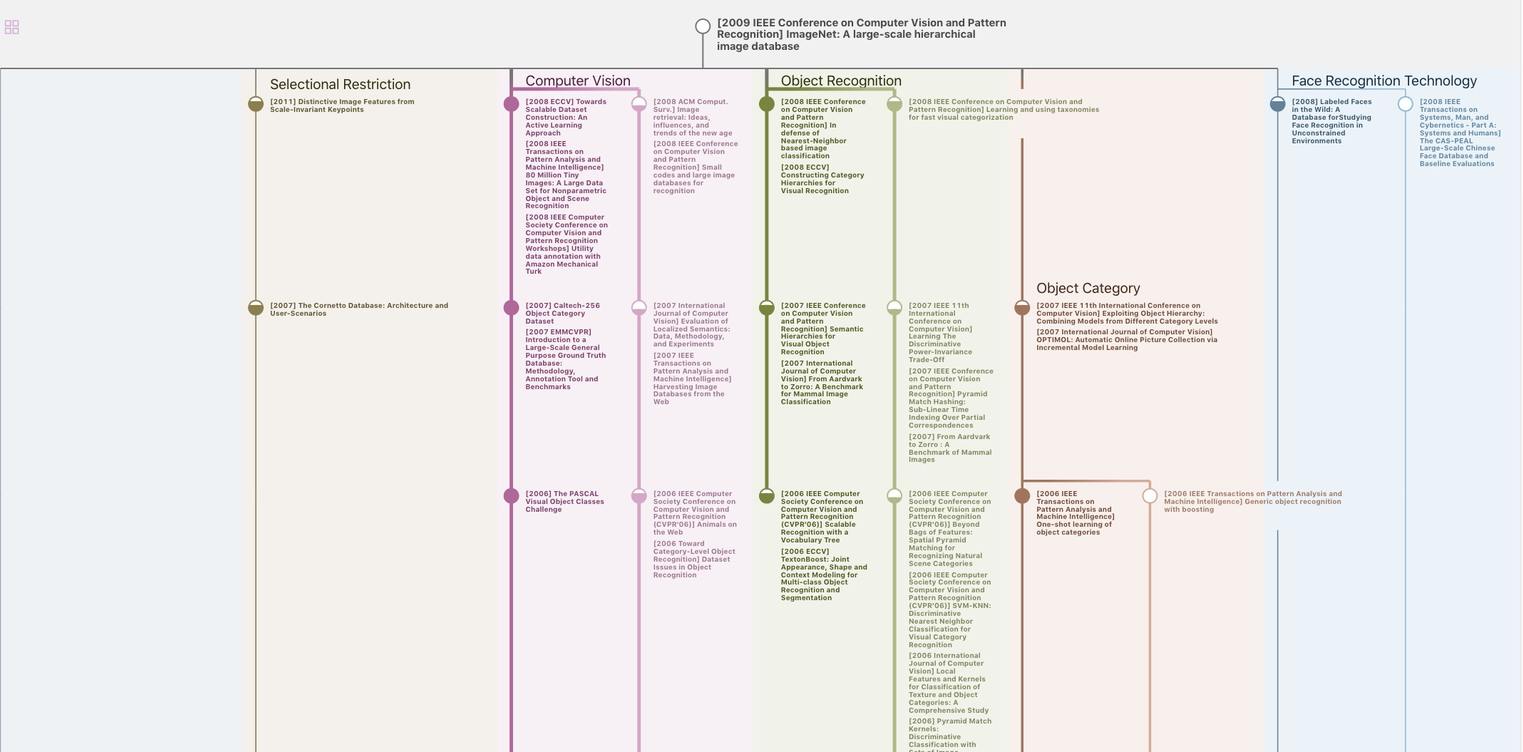
生成溯源树,研究论文发展脉络
Chat Paper
正在生成论文摘要