Meta-Learning Related Tasks With Recurrent Networks: Optimization And Generalization
2018 INTERNATIONAL JOINT CONFERENCE ON NEURAL NETWORKS (IJCNN)(2018)
摘要
There have been recent interest in meta-learning systems: i.e. networks that are trained to learn across multiple tasks. This paper focuses on optimization and generalization of a meta-learning system based on recurrent networks. The optimization investigates the influence of diverse structures and parameters on its performance. We demonstrate the generalization (robustness) of our meta-learning system to learn across multiple tasks including tasks unseen during the meta-training phase. We introduce a meta-cost function (Mean Squared Fair Error) that enhances the performance of the system by not penalizing it during transitions to learning a new task. Evaluation results are presented for Boolean and quadratic functions datasets. The best performance is obtained using a Long Short-Term Memory (LSTM) topology without a forget gate and with a clipped memory cell. The results demonstrate i) the impact of different LSTM architectures, parameters, and error functions on the meta-learning process; ii) that the mean squared fair error function does improve performance for best learning; and iii) the robustness of our meta-learning framework as it generalizes well when tested on tasks unseen during metatraining. Comparison between No-Forget-Gate LSTM and Gated Recurrent Unit also suggest that absence of a memory cell tends to degrade performance.
更多查看译文
关键词
meta-learning, recurrent networks, performance optimization, long short-term memory
AI 理解论文
溯源树
样例
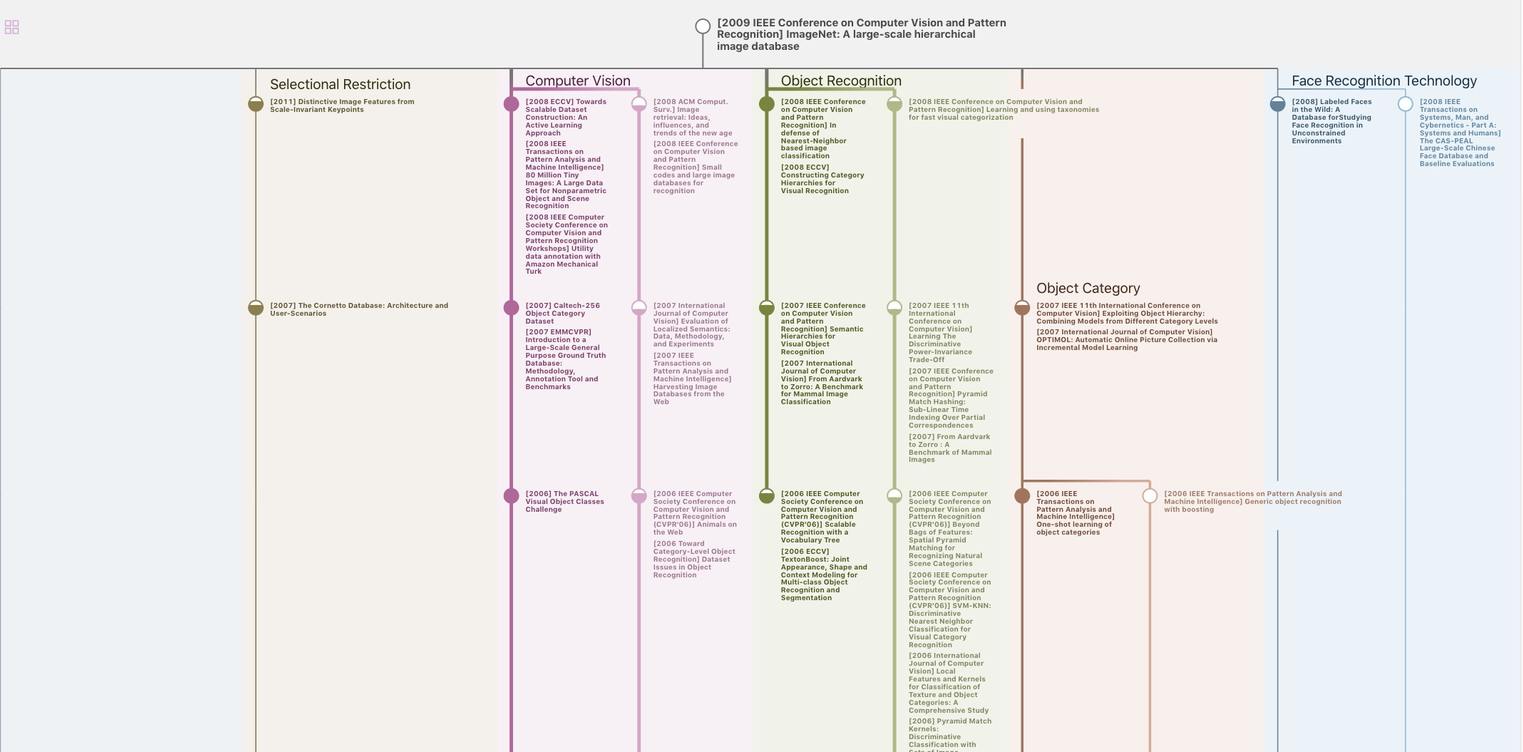
生成溯源树,研究论文发展脉络
Chat Paper
正在生成论文摘要