Snore Sounds Excitation Localization By Using Scattering Transform And Deep Neural Networks
2018 INTERNATIONAL JOINT CONFERENCE ON NEURAL NETWORKS (IJCNN)(2018)
摘要
In this paper, we propose an algorithm for snoring sounds classification based on Deep Scattering Spectrum (SCAT), Gaussian Mixture Models (GMM) Supervectors and Deep Neural Networks (DNN). The task consists in the identification of the type of snoring among four target classes representing the snore sounds' excitation location, which can be highly useful for a successful medical treatment of the habitual snorer or patient afflicted with Obstructive Sleep Apnea. The SCAT is computed from excerpt the acoustic signals, then a GMM Supervector is calculated by adapting the GMM model of the acoustic space with the Maximum a Posteriori (MAP) algorithm and concatenating the mean values of the Gaussians. Resulting supervectors are used to feed the multiclass DNN classifier. The performance of the algorithm has been assessed on the Munich-Passau Snore Sound Corpus (MPSSC), composed of recordings of Drug-Induced Sleep Endoscopy (DISE) examinations. The results are expressed in terms of Unweighted Average Recall (UAR) and a remarkable improvement with respect to the state-of-the-art performance has been registered, achieving a score up to 67.14% and 67.71% respectively on the devel and test datasets.
更多查看译文
关键词
Computational Paralinguistics, Deep Scattering Spectrum, Snoring, GMM Supervectors, Deep Neural Networks
AI 理解论文
溯源树
样例
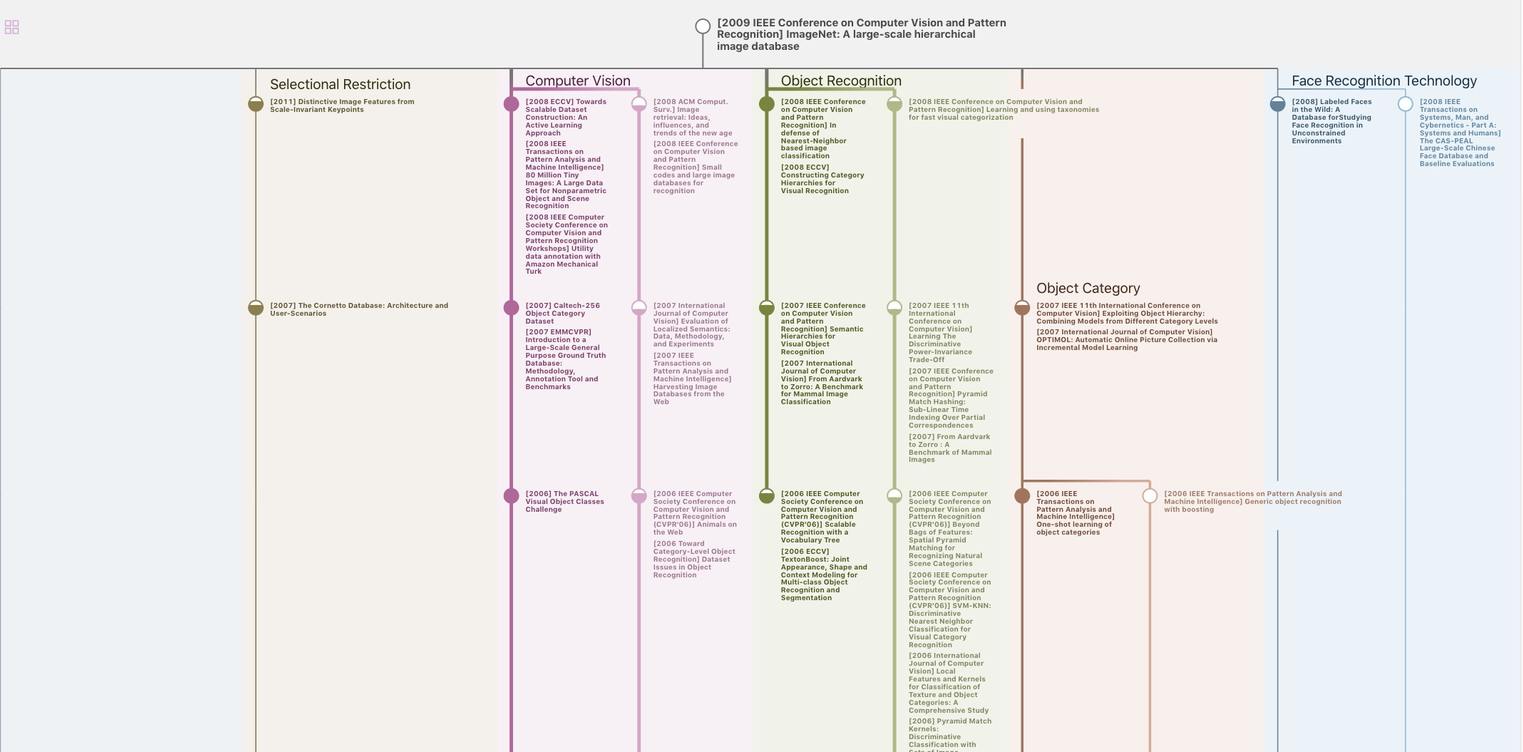
生成溯源树,研究论文发展脉络
Chat Paper
正在生成论文摘要