Attention-Based Bilstm Network With Lexical Feature For Emotion Classification
2018 INTERNATIONAL JOINT CONFERENCE ON NEURAL NETWORKS (IJCNN)(2018)
摘要
Emotion classification is an important task for identifying users' emotional expressions in text. Though a variety of neural models have been proposed nowadays, these models mainly focus on modeling the content of words or characters without fully employing the emotional features in lexical features, especially the features of part-of-speech (POS). In this paper, we reveal that the information of POS as well as that of words is important for identifying the type of emotion in a given text. We propose two simple models to fully learn the emotional features of the POS of words. Every model consists of the long short-term memory (LSTM) network as input encoders and the component of attention mechanism. One model is to concatenate the POS tags of vectors into the hidden states of representations generated by LSTM as raw feature representations and put them into the component of attention mechanism to generate the text representation toward a special emotion. The other is to use both LSTM and attention mechanism to model the context representation of words and those of POS tags respectively and concatenate these context representations as the text representation toward a special emotion. We conduct some experiments on datasets for evaluation and demonstrate the effectiveness of our model, where the datasets consist of the open-source dataset from NLPCC&2014 and the dataset of manual annotation. Experimental results show that our models can achieve outstanding performance for emotion classification in Chinese Weibo texts and outperform classical baselines.
更多查看译文
关键词
LSTM, Attention Mechanism, Part-of-Speech, Emotion Classification, Chinese Weibo Texts
AI 理解论文
溯源树
样例
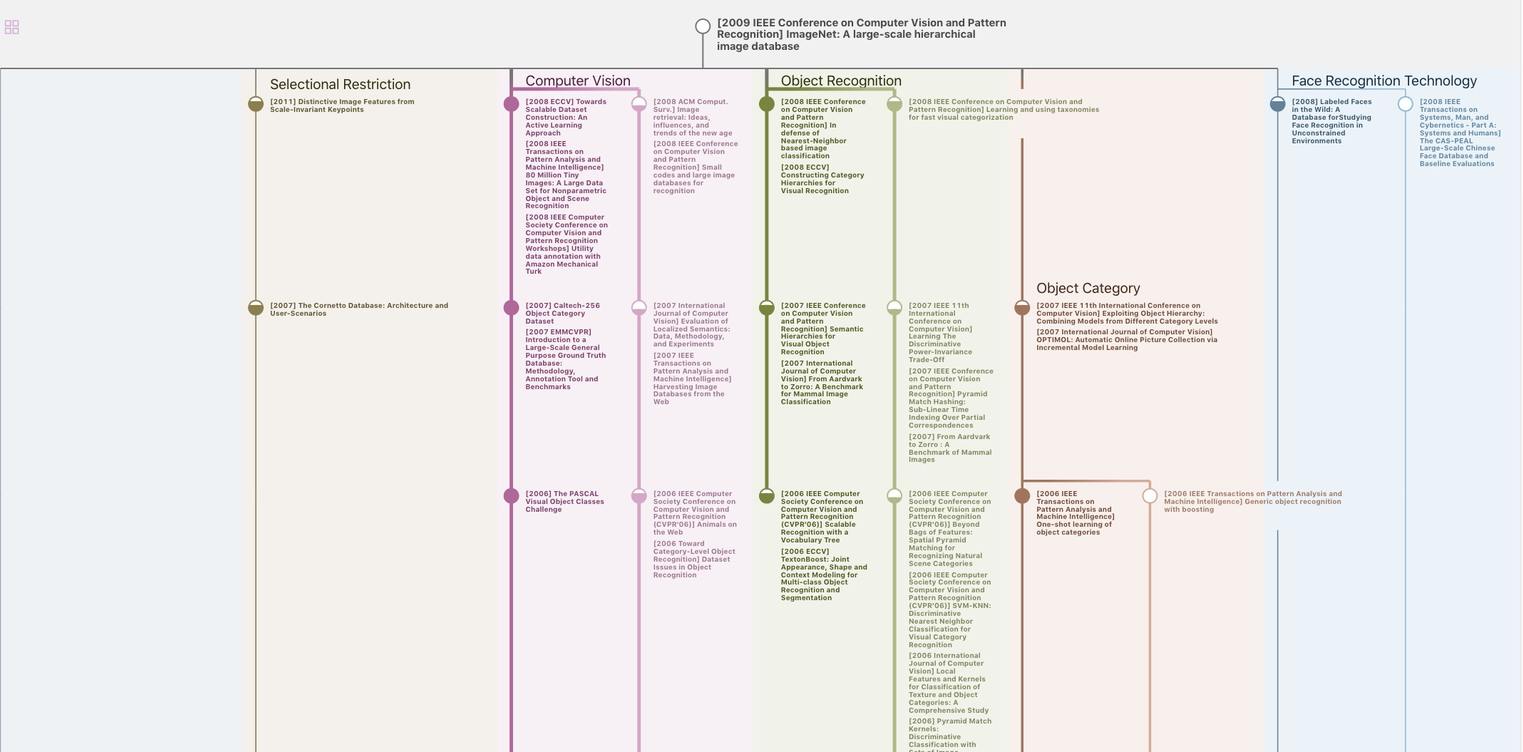
生成溯源树,研究论文发展脉络
Chat Paper
正在生成论文摘要