Methodology Based On Texture, Color And Shape Features For Traffic Light Detection And Recognition.
2018 INTERNATIONAL JOINT CONFERENCE ON NEURAL NETWORKS (IJCNN)(2018)
摘要
Urban roads are very complex. The increase in the flow of vehicles in the cities has contributed to traffic accidents. Researches for accident reduction show that the traffic lights are effective in reducing accidents. Traffic lights can minimize the occurrence of accidents at intersections and crosswalks. The implementation of traffic light signals shows significant advantages, otherwise reveals some problems such as the failure to detect road signs by drivers on urban roads. This fact is related to excessive visual information, the stress of the drivers and/or eyestrain, making the drivers lose their attention. These reasons motivated researches about intelligent vehicles. This work aims to develop a methodology to detect and recognize traffic lights, to be applied in smart vehicles. This methodology can contribute to the Advanced Driver Support Systems (ADAS), which assists drivers, especially those with partial vision impairment. Image processing techniques are used to develop the detection methodology. Back project and global thresholding are combined to find light points. Local thresholding techniques are applied to calculate the symmetry between the radius and the center of the light points to segment the traffic light body. The first step got an average rate of 99% of detection. The features of the traffic lights are extracted using Haralick texture measures, with the inclusion of color and shape information. The data generated by the feature extraction step were pre-processed using the SMOTE technique to balance the database. The recognition and identification of the traffic lights state are made by an artificial neural network using Multilayer-Perceptron (MLP). The backpropagation learning algorithm is used in the network training. The validation results show an average recognition rate of 98%.
更多查看译文
关键词
light points,traffic light body,traffic lights state,shape features,traffic light detection,urban roads,traffic accidents,traffic light signals,drivers,detection methodology,color features,texture features,advanced driver support systems,traffic light recognition,accident reduction,road signs detection,intelligent vehicles,smart vehicles,partial vision impairment,image processing techniques,back project,global thresholding,local thresholding techniques,feature extraction,Haralick texture measures,SMOTE technique,artificial neural network,multilayer-perceptron,backpropagation learning algorithm
AI 理解论文
溯源树
样例
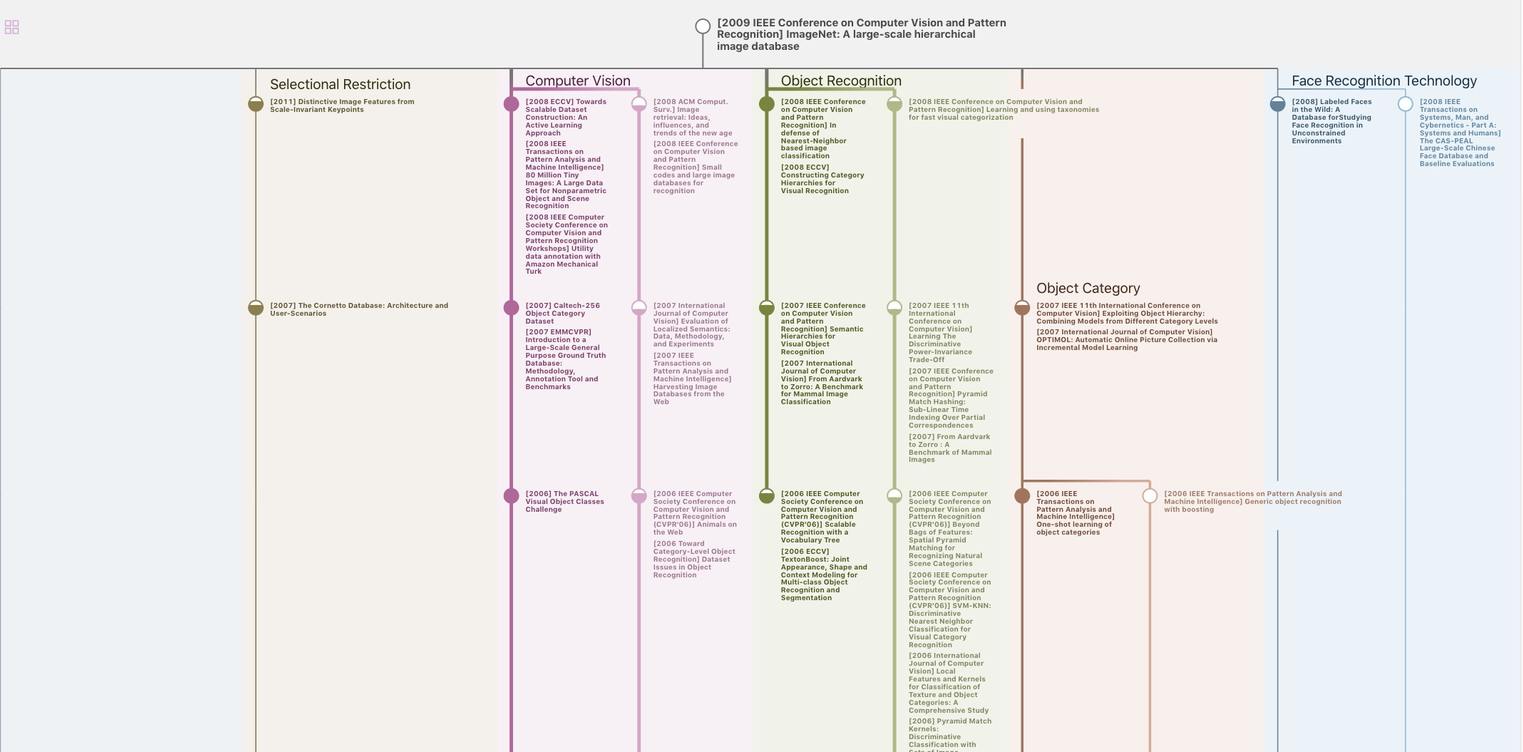
生成溯源树,研究论文发展脉络
Chat Paper
正在生成论文摘要