Temporal Link Prediction Using Cluster And Temporal Information Based Motif Feature
2018 INTERNATIONAL JOINT CONFERENCE ON NEURAL NETWORKS (IJCNN)(2018)
摘要
Predicting future link states of a temporal network from past evolution is an important task from application perspective. Different from static networks analysis, temporal link prediction considers the trends of the links as time goes by, which is proved can achieve higher performance than that only exploring the interactions at a specific moment. The key challenge of this task is to obtain effective features of the evolving network. We propose a novel supervised method CTMF based on motif feature, which can characterize nearly complete local topological characteristic of node-pairs in the temporal network. We also attach cluster information as well as temporal transition factor to capture the influence of global structure and network transition on each motif over time. An unsupervised learning strategy is used on a concatenation of the cluster and temporal information based motif features (CTMF) from the continuous sets of snapshots to capture a better feature representation. We validate the effectiveness of the CTMF by utilizing it for link prediction on a number of temporal datasets, which shows our approach outperforms state-of-art methods using other features.
更多查看译文
关键词
temporal link prediction,future link states,temporal network,static networks analysis,temporal transition factor,global structure,network transition,feature representation,temporal datasets,cluster and temporal information based motif feature,local topological characteristic,node-pairs,unsupervised learning strategy,CTMF,continuous sets
AI 理解论文
溯源树
样例
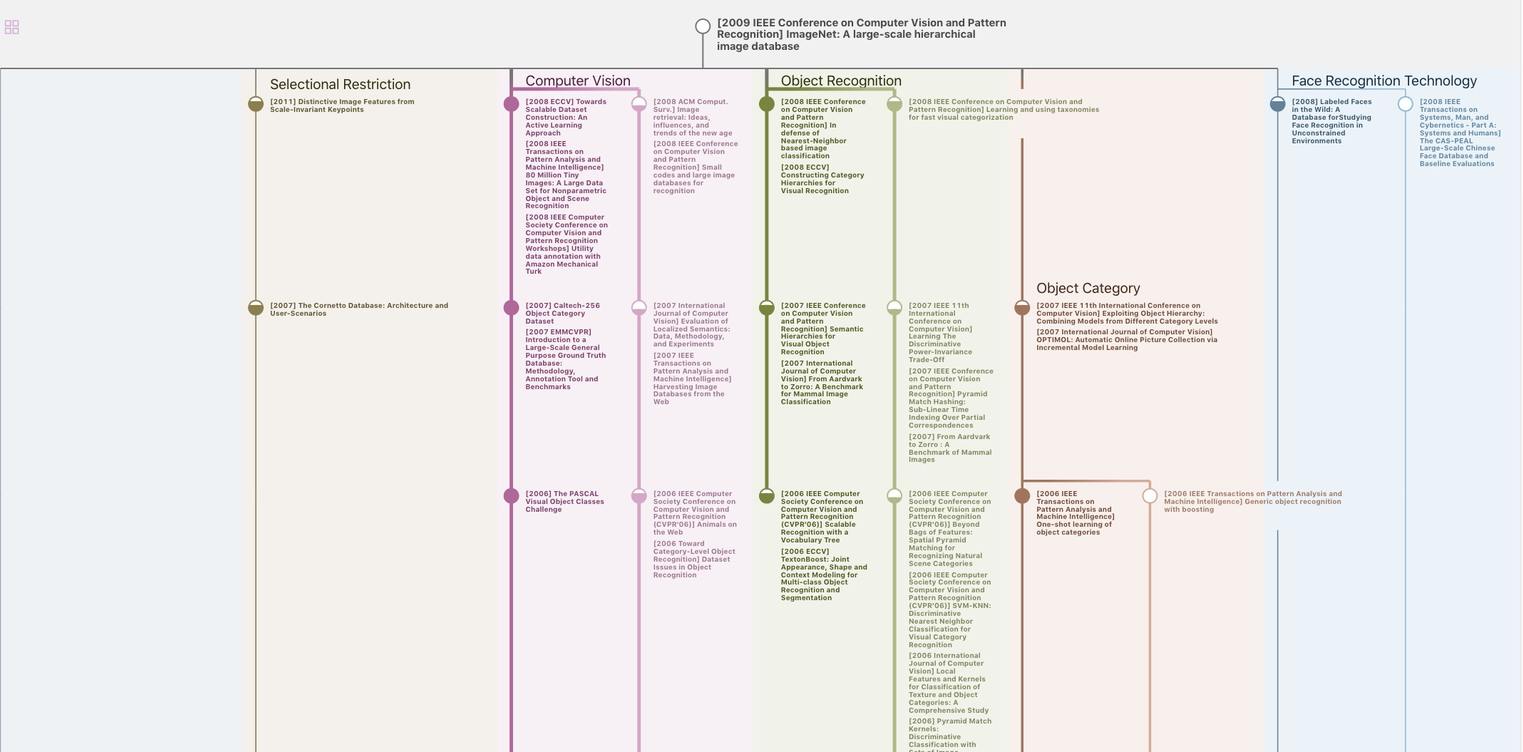
生成溯源树,研究论文发展脉络
Chat Paper
正在生成论文摘要