Drl Based Intelligent Joint Manipulator And Viewing Camera Control For Reaching Tasks And Environments With Obstacles And Occluders
2018 INTERNATIONAL JOINT CONFERENCE ON NEURAL NETWORKS (IJCNN)(2018)
摘要
This work studies joint camera and robotic manipulator control for reaching tasks in complex environments with obstacles and occluders. We obviate the conventional challenges involved in complex perception, planning, and control modules and careful calibration for sensing and actuation and seek a solution leveraging deep reinforcement learning (DRL). Our method using DRL and deep Q-learning learns a policy for robot actuation and perception control, mapping directly raw image pixels inputs into camera motion and manipulator joint control actions outputs. We show results comparing different training approaches, and demonstrating competency for increasingly complex situations and degrees of freedom. These preliminary experiments suggest the effectiveness and robustness of the proposed approach.
更多查看译文
关键词
Deep reinforcement learning, joint actuation and perception control, deep Q-learning
AI 理解论文
溯源树
样例
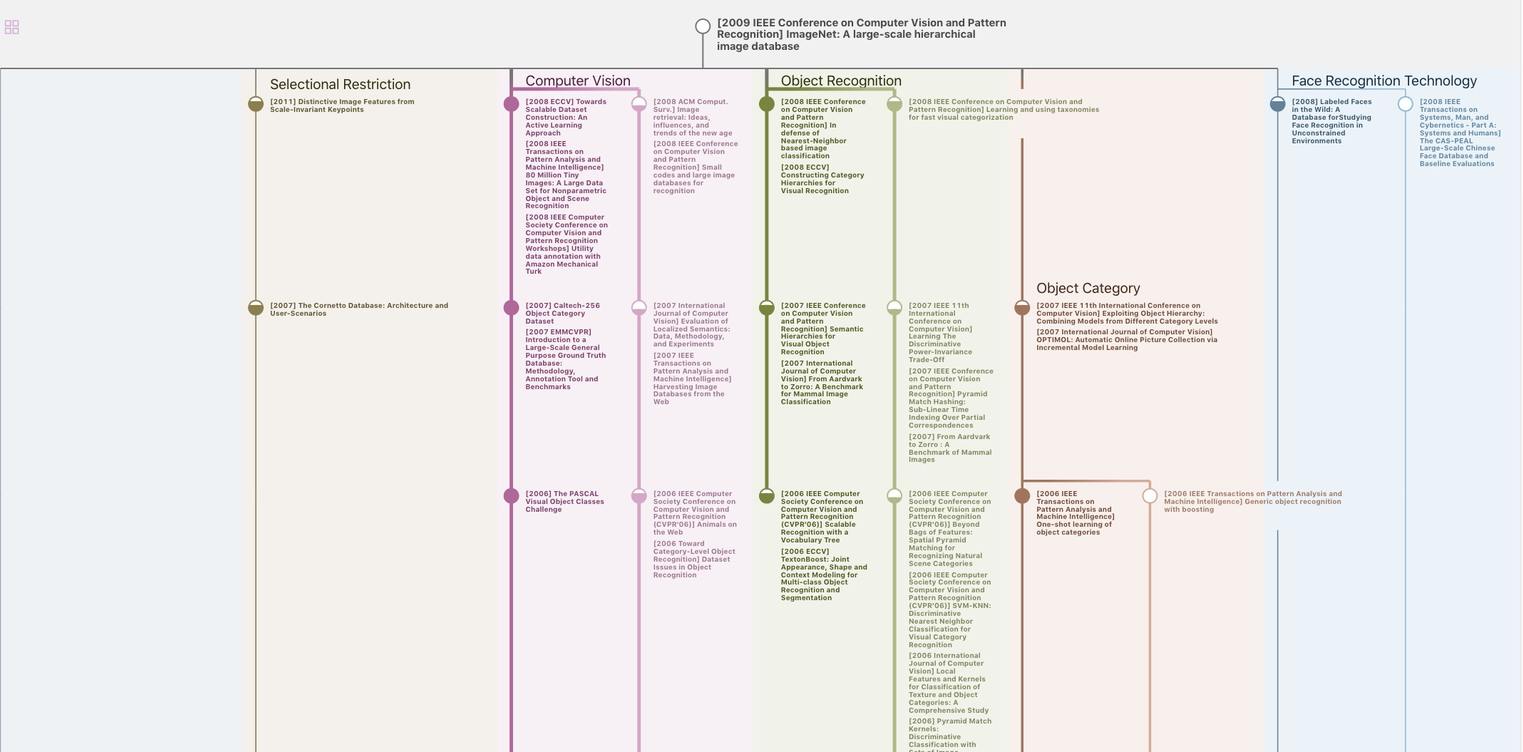
生成溯源树,研究论文发展脉络
Chat Paper
正在生成论文摘要