Constrained Probabilistic Matrix Factorization With Neural Network For Recommendation System
INTELLIGENT INFORMATION PROCESSING IX(2018)
摘要
In order to alleviate the problem of rating sparsity in recommendation system, this paper proposes a model called Constrained Probabilistic Matrix Factorization with Neural Network (CPMF-NN). In user modeling, it takes the influence of users' interaction items into consideration. In item modeling, it utilizes convolutional neural network to extract the item latent features from the corresponding documents. In the process of fusion of latent feature vectors, multi-layer perceptron is used to grasp the nonlinear structural characteristics of user-item interactions. Through extensive experiments on three real-world datasets, the results show that CPMF-NN achieves good performance on different sparse data sets.
更多查看译文
关键词
Collaborative filtering, User preference modeling, Document modeling, Nonlinear fusion
AI 理解论文
溯源树
样例
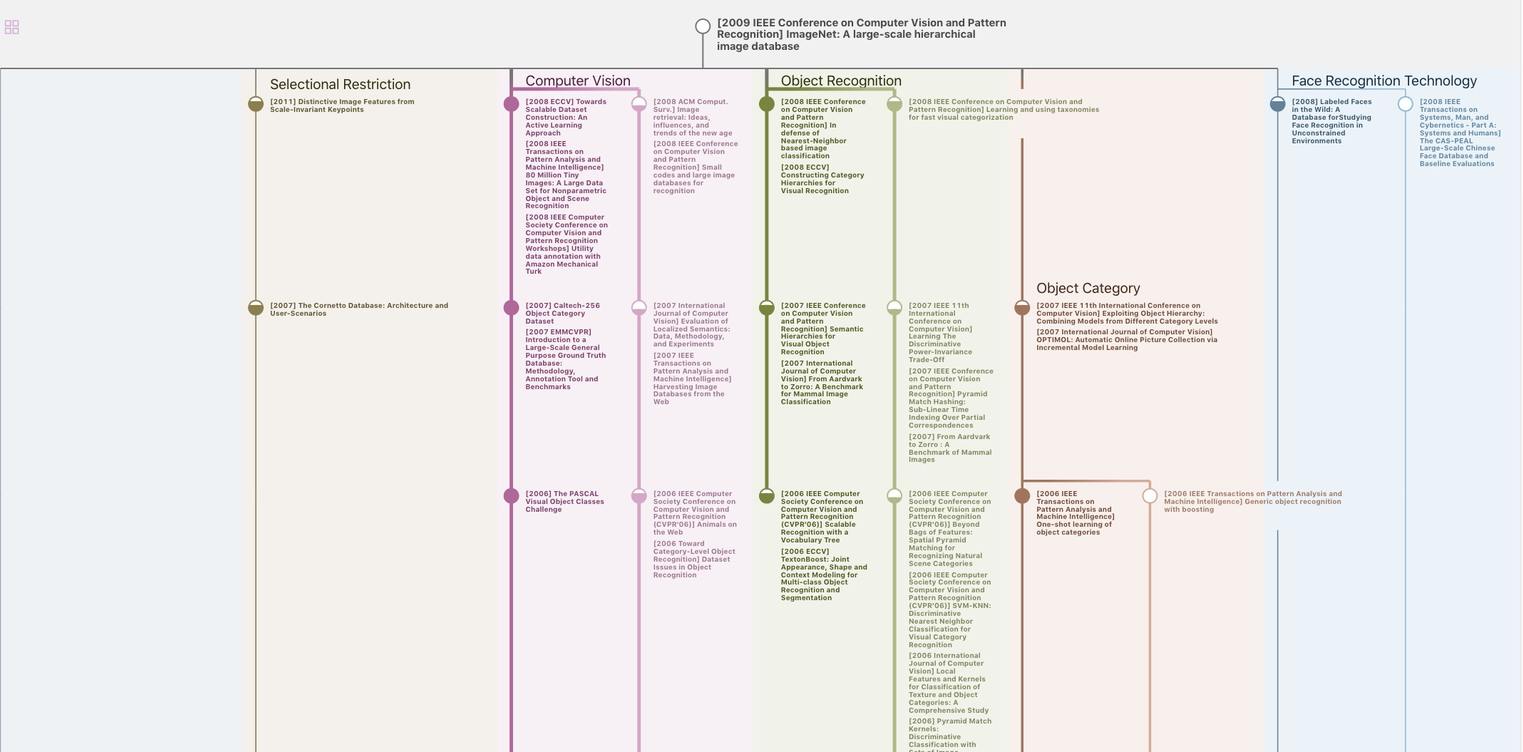
生成溯源树,研究论文发展脉络
Chat Paper
正在生成论文摘要