Analysing the Footprint of Classifiers in Overlapped and Imbalanced Contexts.
IDA(2018)
摘要
It is recognised that the imbalanced data problem is aggravated by other difficulty factors, such as class overlap. Over the years, several research works have focused on this problematic, although presenting two major hitches: the limitation of test domains and the lack of a formulation of the overlap degree, which makes results hard to generalise. This work studies the performance degradation of classifiers with distinct learning biases in overlap and imbalanced contexts, focusing on the characteristics of the test domains (shape, dimensionality and imbalance ratio) and on to what extent our proposed overlapping measure (degOver) is aligned with the performance results observed. Our results show that MLP and CART classifiers are the most robust to high levels of class overlap, even for complex domains, and that KNN and linear SVM are the most aligned with degOver. Furthermore, we found that the dimensionality of data also plays an important role in explaining performance results.
更多查看译文
关键词
Imbalanced data, Class overlap, Machine learning classifiers
AI 理解论文
溯源树
样例
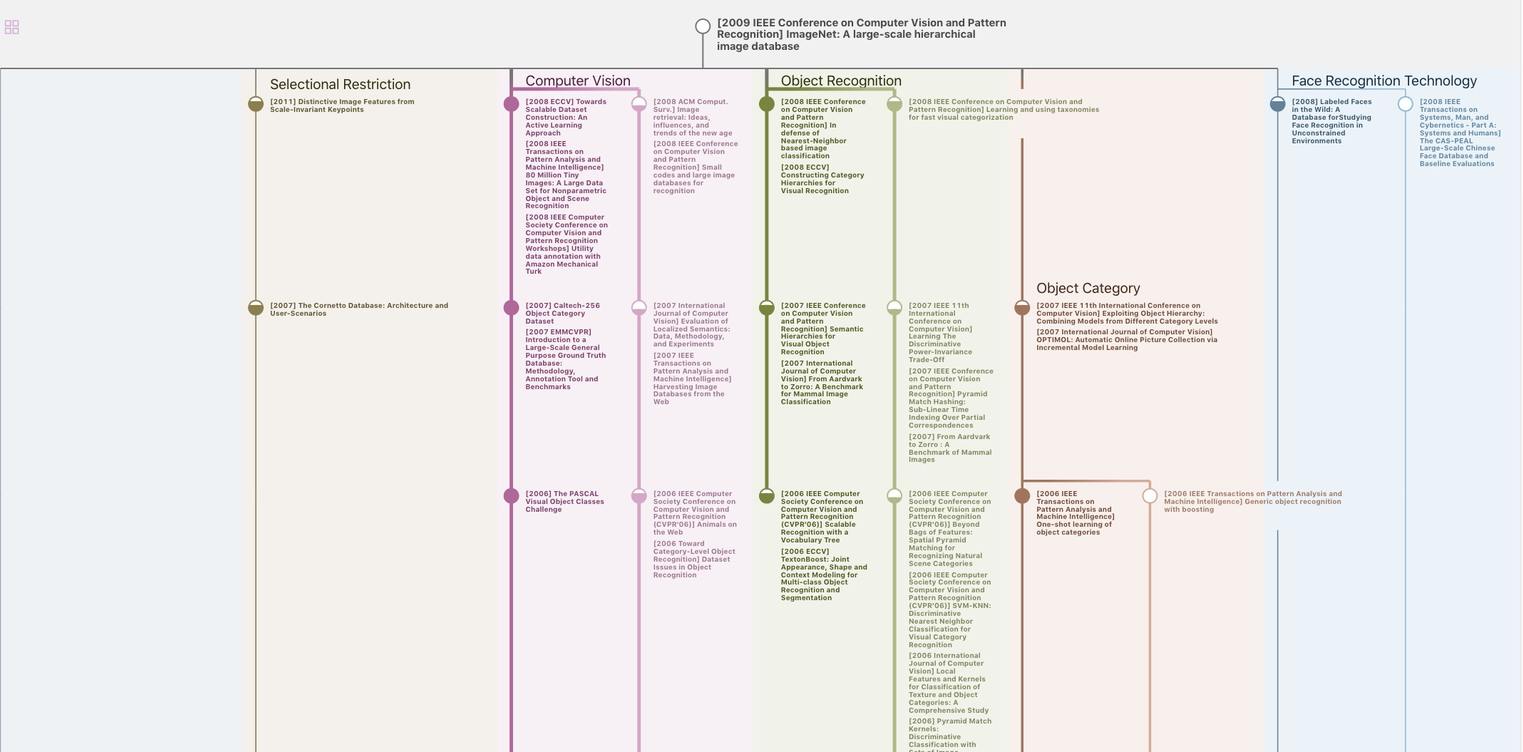
生成溯源树,研究论文发展脉络
Chat Paper
正在生成论文摘要