Action unit detection and key frame selection for human activity prediction.
Neurocomputing(2018)
摘要
Human activity prediction aims to recognize an unfinished activity with limited appearance and motion information. In this paper, we propose to predict an incomplete activity by combining the mid-level action units and the discriminative key frames exploited from each activity class. Specifically, we extract a great deal of action-related volumes from activity videos. Based on a set of low-level powerful features, similar volumes are aggregated into a mid-level feature, named action unit. Then, we detect these action units in each activity video and generate the frame feature by computing the distribution of concurrent action units in a single frame. Notice that human can easily recognize an incomplete activity using scanty key frames composed of representative interrelated action units together. The key frames in each activity class are selected by computing the entropy of each single frame feature. Finally, a structured SVM is trained to recognize activities with different observation ratios. The proposed approach is evaluated on several publicly available datasets in comparison with state-of-the-art approaches. The experimental results and analysis clearly demonstrate the effectiveness of the proposed approach.
更多查看译文
关键词
Activity prediction,Key frame selection,Action unit detection,Structured SVM
AI 理解论文
溯源树
样例
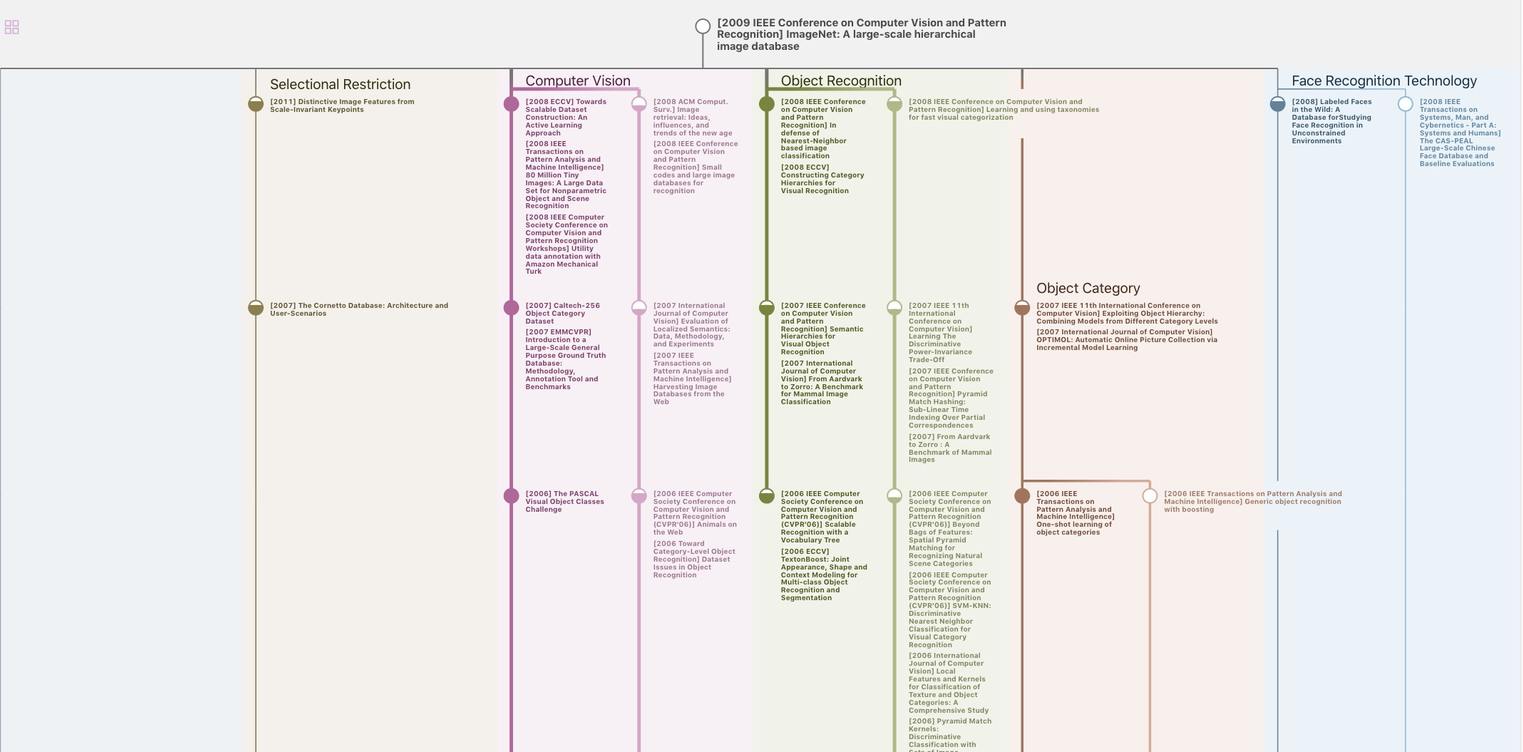
生成溯源树,研究论文发展脉络
Chat Paper
正在生成论文摘要