Multi-instance Transfer Metric Learning by Weighted Distribution and Consistent Maximum Likelihood Estimation
Neurocomputing(2018)
摘要
Multi-Instance learning (MIL) aims to predict labels of unlabeled bags by training a model with labeled bags. The usual assumption of existing MIL methods is that the underlying distribution of training data is the same as that of the testing data. However, this assumption may not be valid in practice, especially when training data from a source domain and testing data from a target domain are drawn from different distributions. In this paper, we put forward a novel algorithm Multi-Instance Transfer Metric Learning (MITML). Specially, MITML first attempts to bridge the distributions of different domains by using the bag weighting method. Then a consistent maximum likelihood estimation method is learned to construct an optimal distance metric and exploited to classify testing bags. Comprehensive experimental results on benchmark datasets have demonstrated that the learning performance of the proposed MITML algorithm is better than those of other state-of-the-art MIL algorithms.
更多查看译文
关键词
Multi-instance learning,Transfer learning,Metric learning,Bag weights estimation,Consistent maximum likelihood estimation
AI 理解论文
溯源树
样例
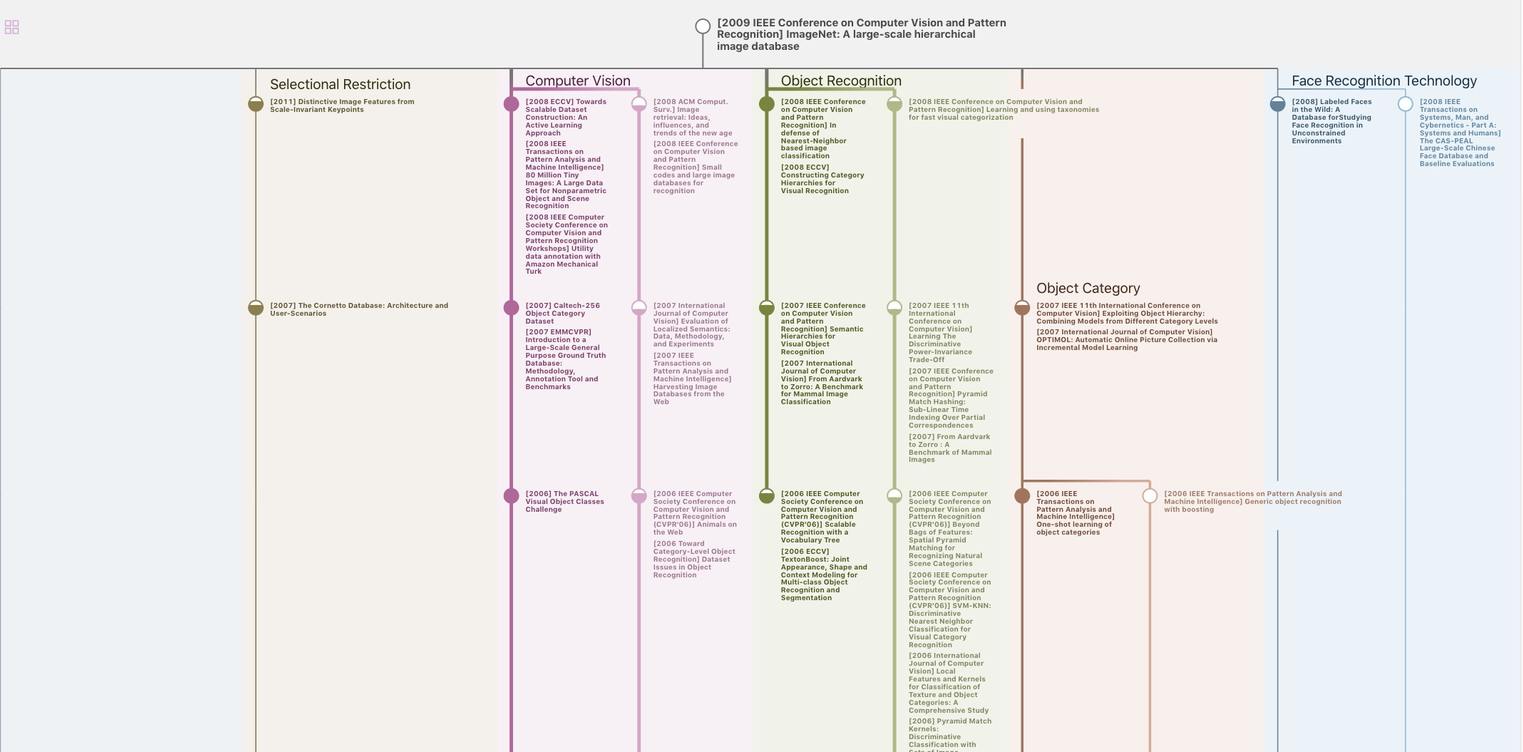
生成溯源树,研究论文发展脉络
Chat Paper
正在生成论文摘要