CrowdTravel: scenic spot profiling by using heterogeneous crowdsourced data
J. Ambient Intelligence and Humanized Computing(2017)
摘要
Traveling is one of the most important entertainments in the modern society. In general, before traveling to an unfamiliar city, one of the possible ways of travel planning is to search travel information from travel-related websites. With the advances of mobile social networks, increasingly more people are willing to record and share their travel experience via social media, which provides abundant information for people who are going to make travel plans. Textual reviews and travelogues with large scale of photos are two kinds of popular social travel sharing. They are complementary to each other in terms of structure and content, forming a large amount of fragmented travel knowledge. Moreover, the ever-increasing reviews and travelogues may impose a huge burden on gaining and reorganizing travel knowledge. To address these issues, this paper proposes CrowdTravel, a multi-source social media data fusion approach for multi-aspect tourism information perception, which can provide travelling assistance for tourists by crowd intelligence mining. We first study the problem of discovering popular scenic spots over crowd contributed data. Second, we propose a cross-media multi-aspect correlation method to connect fragmented travel information. Then we mine popular travel routes from travelogues based on Sequential Pattern Mining Algorithm. Finally, we achieve cross-media information relevance based on the similarity between the reviews and image contexts. We conduct experiments over a dataset of several popular scenic spots in Beijing and Xi’an, which is collected from two major online travel websites, namely Dazhongdianping and Mafengwo. The results indicate that our approach attains fine-grained characterization for the scenic spots and delivers excellent performance.
更多查看译文
关键词
Crowd intelligence, Scenic spot profiling, Social media data fusion, Multi-aspect characterization
AI 理解论文
溯源树
样例
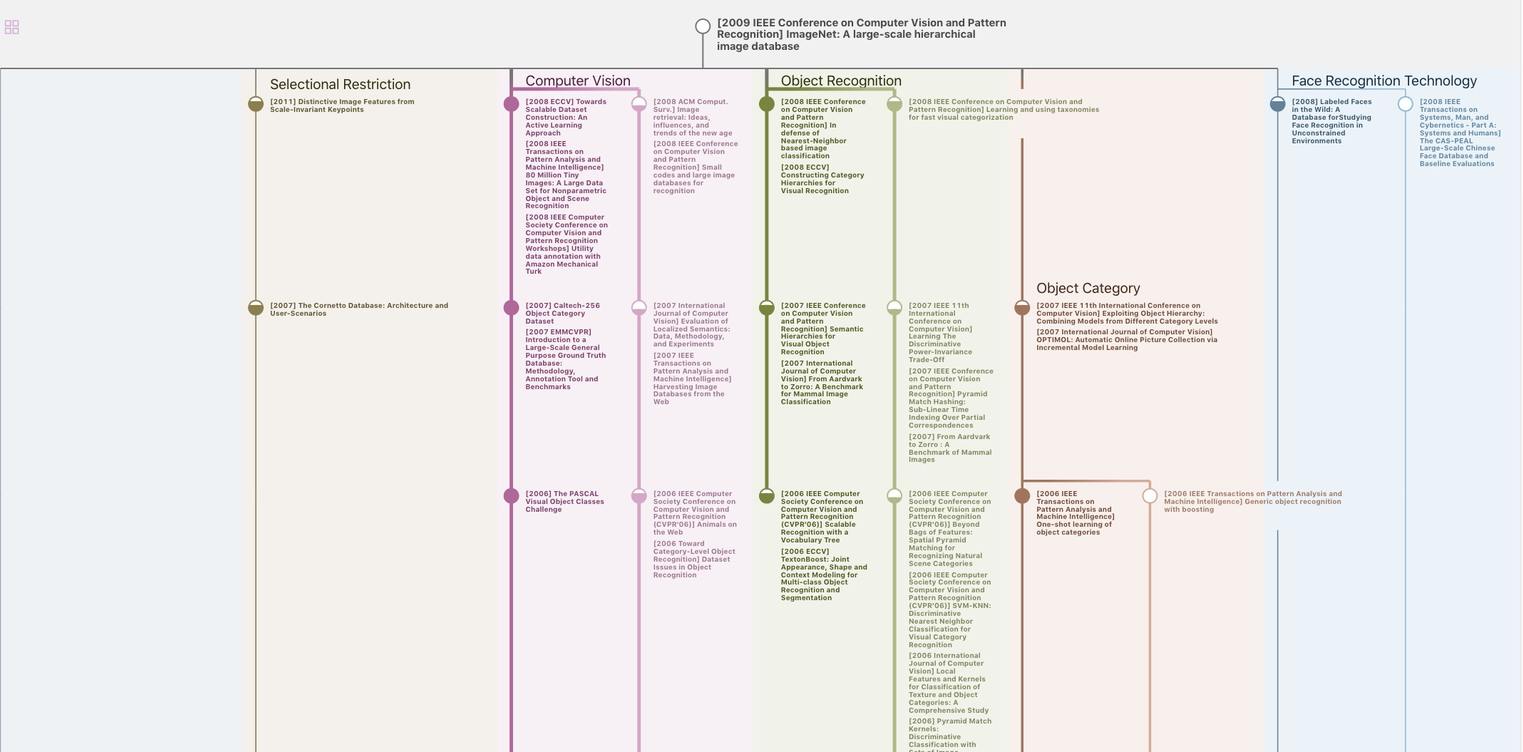
生成溯源树,研究论文发展脉络
Chat Paper
正在生成论文摘要