Unsupervised Change Detection Using Fast Fuzzy Clustering for Landslide Mapping from Very High-Resolution Images.
REMOTE SENSING(2018)
摘要
Change detection approaches based on image segmentation are often used for landslide mapping (LM) from very high-resolution (VHR) remote sensing images. However, these approaches usually have two limitations. One is that they are sensitive to thresholds used for image segmentation and require too many parameters. The other one is that the computational complexity of these approaches depends on the image size, and thus they require a long execution time for very high-resolution (VHR) remote sensing images. In this paper, an unsupervised change detection using fast fuzzy c-means clustering (CDFFCM) for LM is proposed. The proposed CDFFCM has two contributions. The first is that we employ a Gaussian pyramid-based fast fuzzy c-means (FCM) clustering algorithm to obtain candidate landslide regions that have a better visual effect due to the utilization of image spatial information. The second is that we use the difference of image structure information instead of grayscale difference to obtain more accurate landslide regions. Three comparative approaches, edge-based level-set (ELSE), region-based level-set (RLSE), and change detection-based Markov random field (CDMRF), and the proposed CDFFCM are evaluated in three true landslide cases in the Lantau area of Hong Kong. The experiments show that the proposed CDFFCM is superior to three comparative approaches in terms of higher accuracy, fewer parameters, and shorter execution time.
更多查看译文
关键词
landslide mapping (LM),change detection,image segmentation,fuzzy c-means (FCM) clustering
AI 理解论文
溯源树
样例
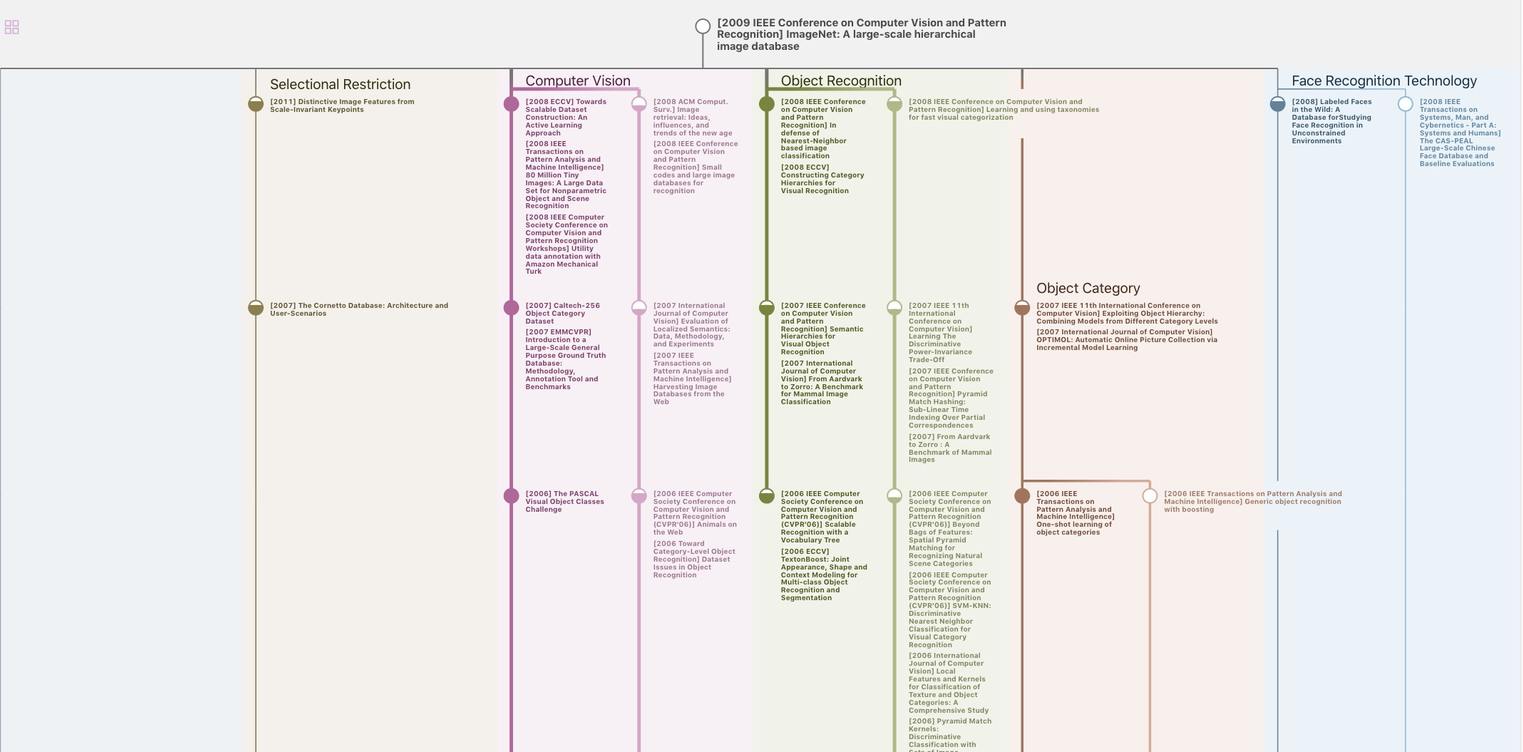
生成溯源树,研究论文发展脉络
Chat Paper
正在生成论文摘要