Vector minimax concave penalty for sparse representation.
Digital Signal Processing(2018)
摘要
This paper proposes vector minimax concave (VMC) penalty for sparse representation using tools of Moreau envelope. The VMC penalty is a weighted MC function; by fine tuning the weight of the VMC penalty with given strategy, the VMC regularized least squares problem shares the same global minimizers with the L0 regularization problem but has fewer local minima. Facilitated by the alternating direction method of multipliers (ADMM), the VMC regularization problem can be tackled as a sequence of convex sub-problems, each of which can be solved fast. Theoretical analysis of ADMM shows that the convergence of solving the VMC regularization problem is guaranteed. We present a series of numerical experiments demonstrating the superior performance of the VMC penalty and the ADMM algorithm in broad applications for sparse representation, including sparse denoising, sparse deconvolution, and missing data estimation.
更多查看译文
关键词
Nonconvex sparsity regularization,Minimax-concave penalty,CEL0,Sparse representation
AI 理解论文
溯源树
样例
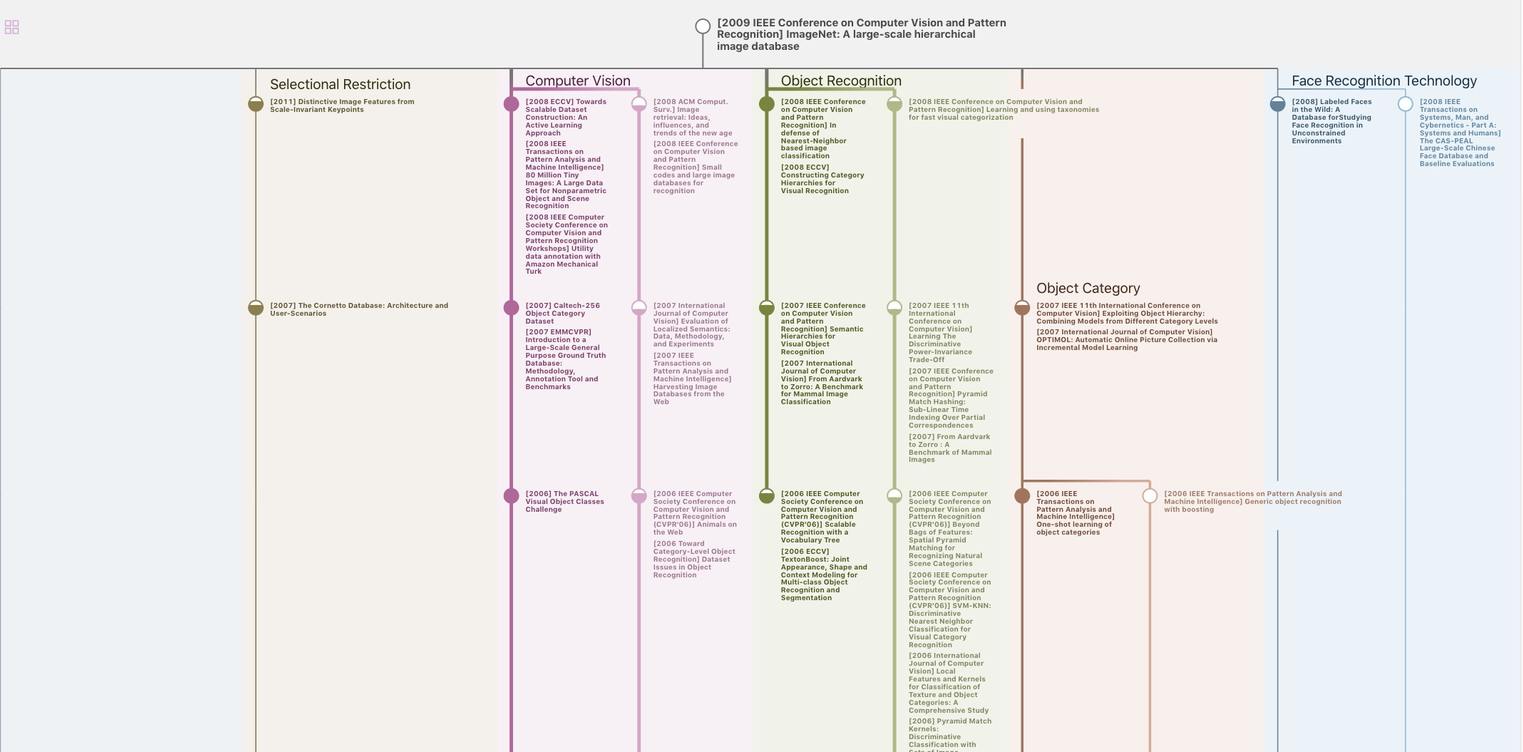
生成溯源树,研究论文发展脉络
Chat Paper
正在生成论文摘要