Shaping the collision selectivity in a looming sensitive neuron model with parallel ON and OFF pathways and spike frequency adaptation.
Neural Networks(2018)
摘要
Shaping the collision selectivity in vision-based artificial collision-detecting systems is still an open challenge. This paper presents a novel neuron model of a locust looming detector, i.e. the lobula giant movement detector (LGMD1), in order to provide effective solutions to enhance the collision selectivity of looming objects over other visual challenges. We propose an approach to model the biologically plausible mechanisms of ON and OFF pathways and a biophysical mechanism of spike frequency adaptation (SFA) in the proposed LGMD1 visual neural network. The ON and OFF pathways can separate both dark and light looming features for parallel spatiotemporal computations. This works effectively on perceiving a potential collision from dark or light objects that approach; such a bio-plausible structure can also separate LGMD1’s collision selectivity to its neighbouring looming detector — the LGMD2. The SFA mechanism can enhance the LGMD1’s collision selectivity to approaching objects rather than receding and translating stimuli, which is a significant improvement compared with similar LGMD1 neuron models. The proposed framework has been tested using off-line tests of synthetic and real-world stimuli, as well as on-line bio-robotic tests. The enhanced collision selectivity of the proposed model has been validated in systematic experiments. The computational simplicity and robustness of this work have also been verified by the bio-robotic tests, which demonstrates potential in building neuromorphic sensors for collision detection in both a fast and reliable manner.
更多查看译文
关键词
Locusts,LGMD1,Neuron model,Collision selectivity,ON and OFF pathways,Bio-robotics
AI 理解论文
溯源树
样例
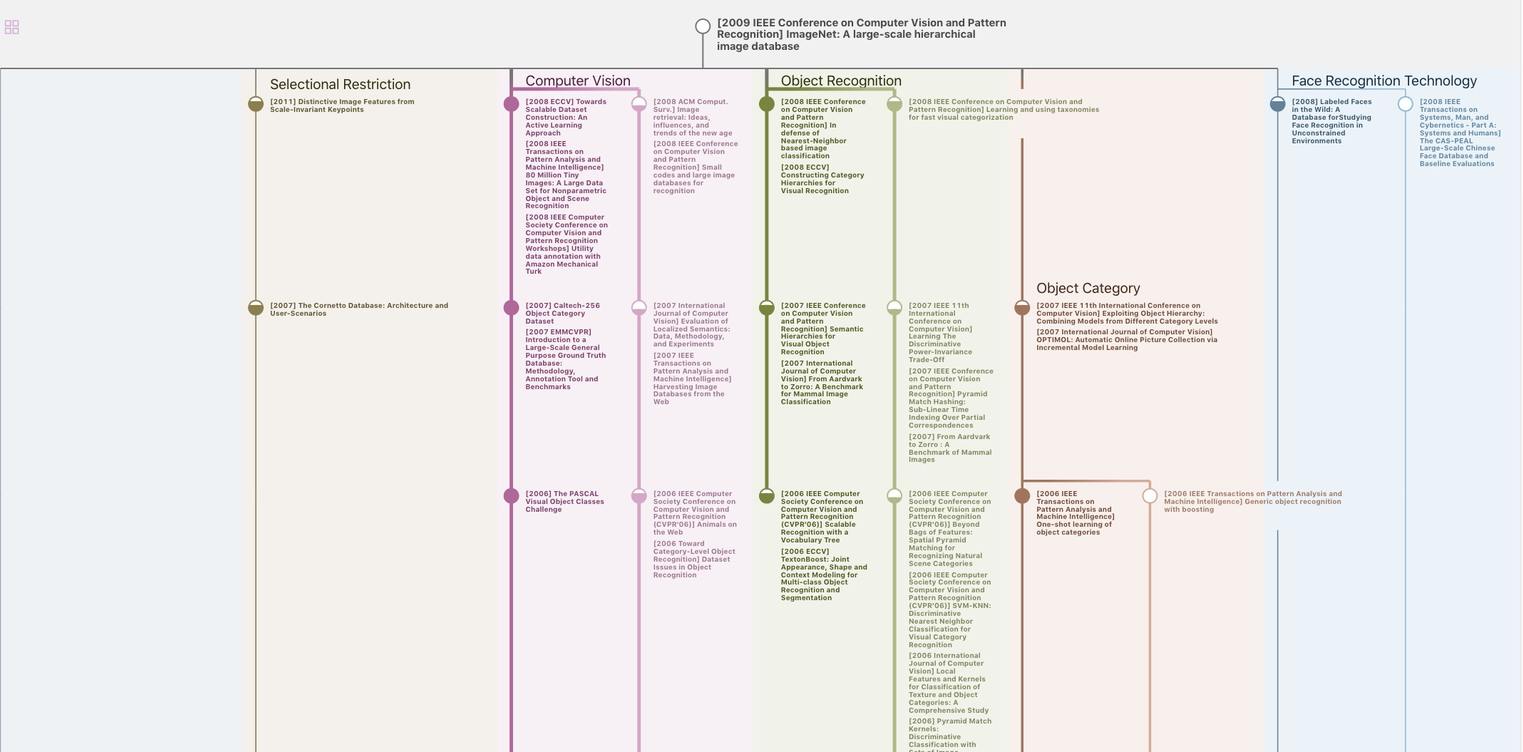
生成溯源树,研究论文发展脉络
Chat Paper
正在生成论文摘要