A Hybrid Ensemble Learning Method For Tourist Route Recommendations Based On Geo-Tagged Social Networks
INTERNATIONAL JOURNAL OF GEOGRAPHICAL INFORMATION SCIENCE(2018)
摘要
Geo-tagged travel photos on social networks often contain location data such as points of interest (POIs), and also users' travel preferences. In this paper, we propose a hybrid ensemble learning method, BAyes-Knn, that predicts personalized tourist routes for travelers by mining their geographical preferences from these location-tagged data. Our method trains two types of base classifiers to jointly predict the next travel destination: (1) The K-nearest neighbor (KNN) classifier quantifies users' location history, weather condition, temperature and seasonalityand uses a feature-weighted distance model to predict a user's personalized interests in an unvisited location. (2) A Bayes classifier introduces a smooth kernel function to estimate a-priori probabilities of features and then combines these probabilities to predict a user's latent interests in a location. All the outcomes from these subclassifiers are merged into one final prediction result by using the Borda count voting method. We evaluated our method on geo-tagged Flickr photos and Beijing weather data collected from 1 January 2005 to 1 July 2016. The results demonstrated that our ensemble approach outperformed 12 other baseline models. In addition, the results showed that our framework has better prediction accuracy than do context-aware significant travel-sequence-patterns recommendations and frequent travel-sequence patterns.
更多查看译文
关键词
Tour recommendations, spatial data mining, volunteered geographic information, location-based social networks, ensemble learning method
AI 理解论文
溯源树
样例
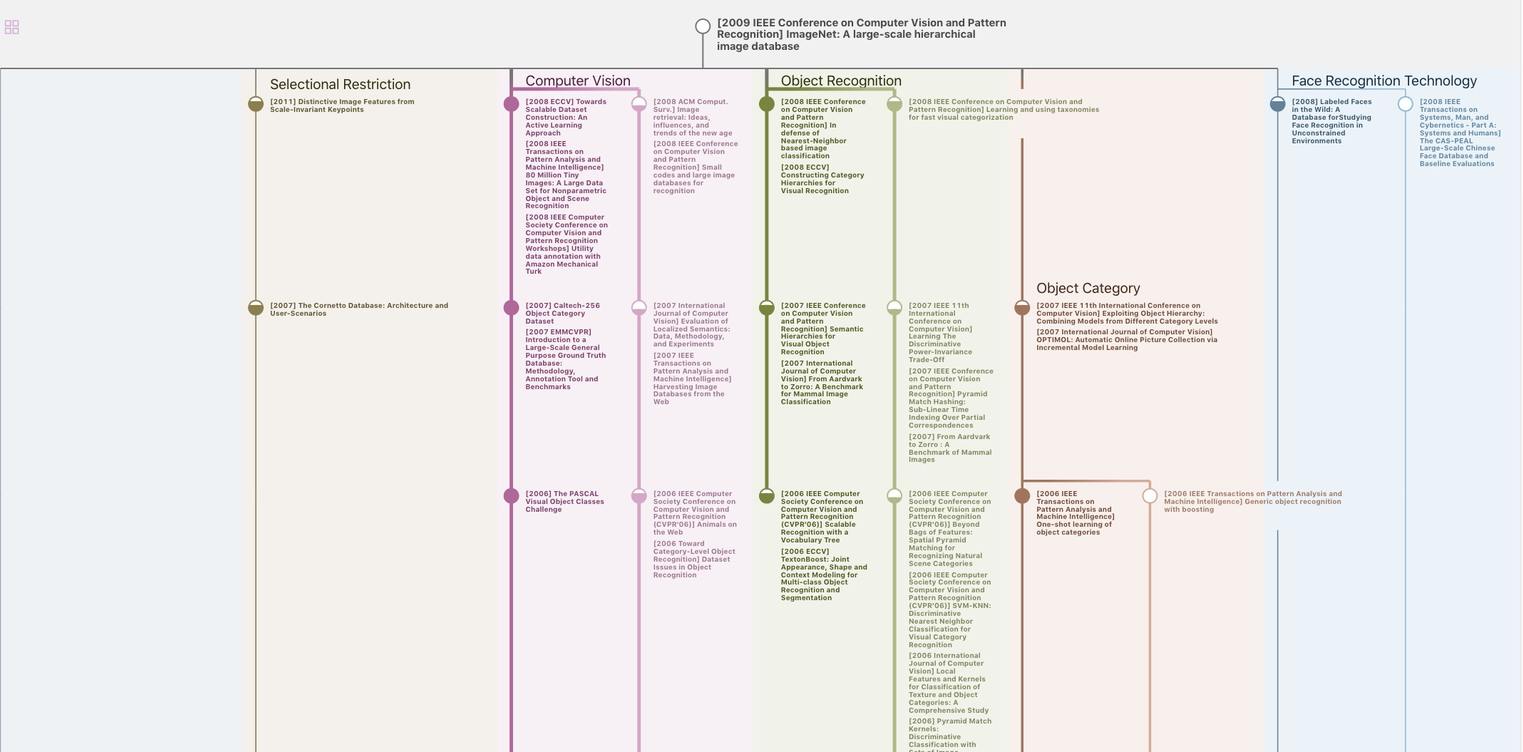
生成溯源树,研究论文发展脉络
Chat Paper
正在生成论文摘要