A Non-Euclidean Gradient Descent Framework for Non-Convex Matrix Factorization.
IEEE Transactions on Signal Processing(2018)
摘要
We study convex optimization problems that feature low-rank matrix solutions. In such scenarios, non-convex methods offer significant advantages over convex methods due to their lower space complexity, as well as practical faster convergence. Under mild assumptions, these methods feature global convergence guarantees. In this paper, we extend the results on this matter by following a different pat...
更多查看译文
关键词
Convergence,Gradient methods,Machine learning,Signal processing algorithms,Standards,Matrix decomposition
AI 理解论文
溯源树
样例
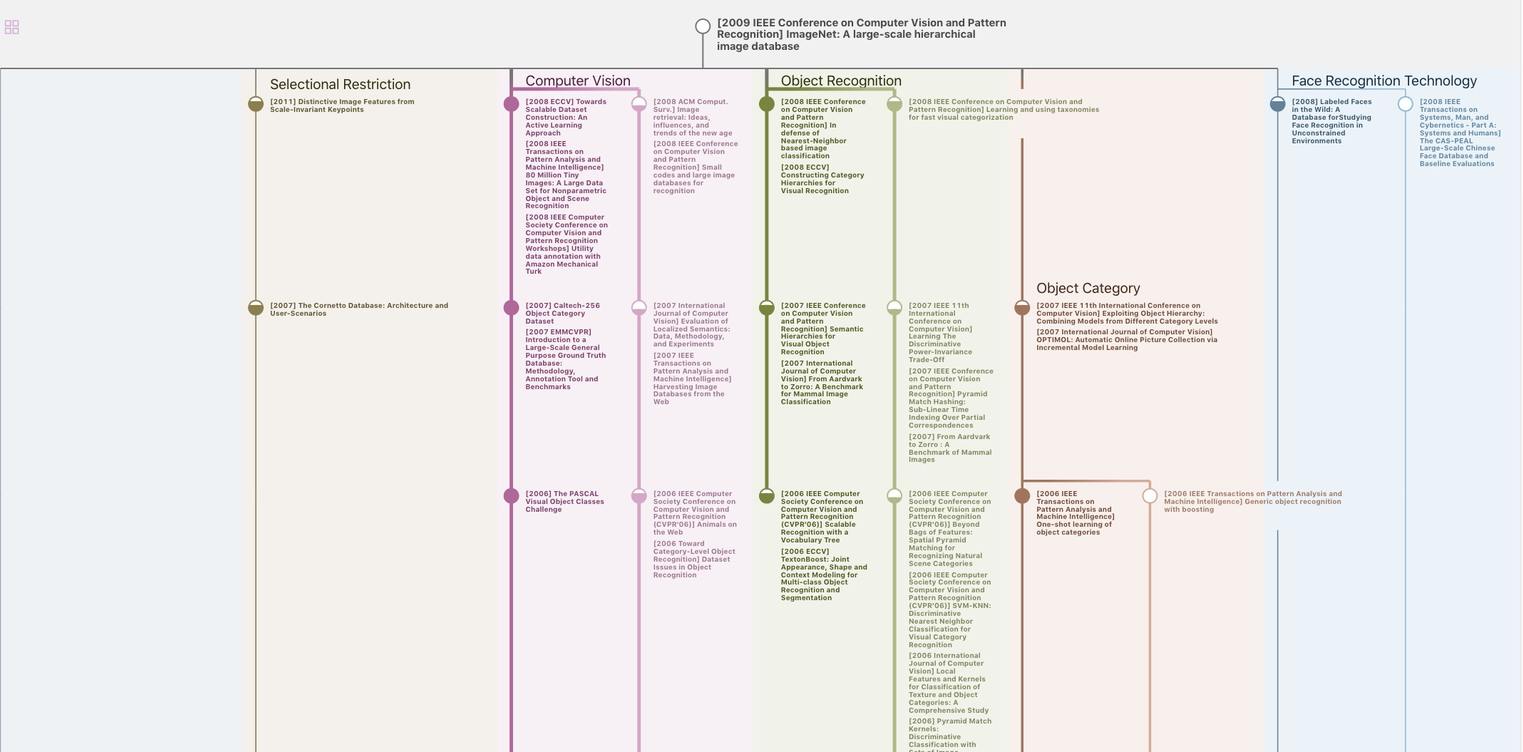
生成溯源树,研究论文发展脉络
Chat Paper
正在生成论文摘要