Variational Bayesian Inference for Robust Streaming Tensor Factorization and Completion
2018 IEEE International Conference on Data Mining (ICDM)(2018)
摘要
Streaming tensor factorization is a powerful tool for processing high-volume and multi-way temporal data in Internet networks, recommender systems and image/video data analysis. Existing streaming tensor factorization algorithms rely on least-squares data fitting and they do not possess a mechanism for tensor rank determination. This leaves them susceptible to outliers and vulnerable to over-fitting. This paper presents a Bayesian robust streaming tensor factorization model to identify sparse outliers, automatically determine the underlying tensor rank and accurately fit low-rank structure. We implement our model in Matlab and compare it with existing algorithms on tensor datasets generated from dynamic MRI and Internet traffic.
更多查看译文
关键词
streaming data,tensor factorization,tensor completion,variational bayesian
AI 理解论文
溯源树
样例
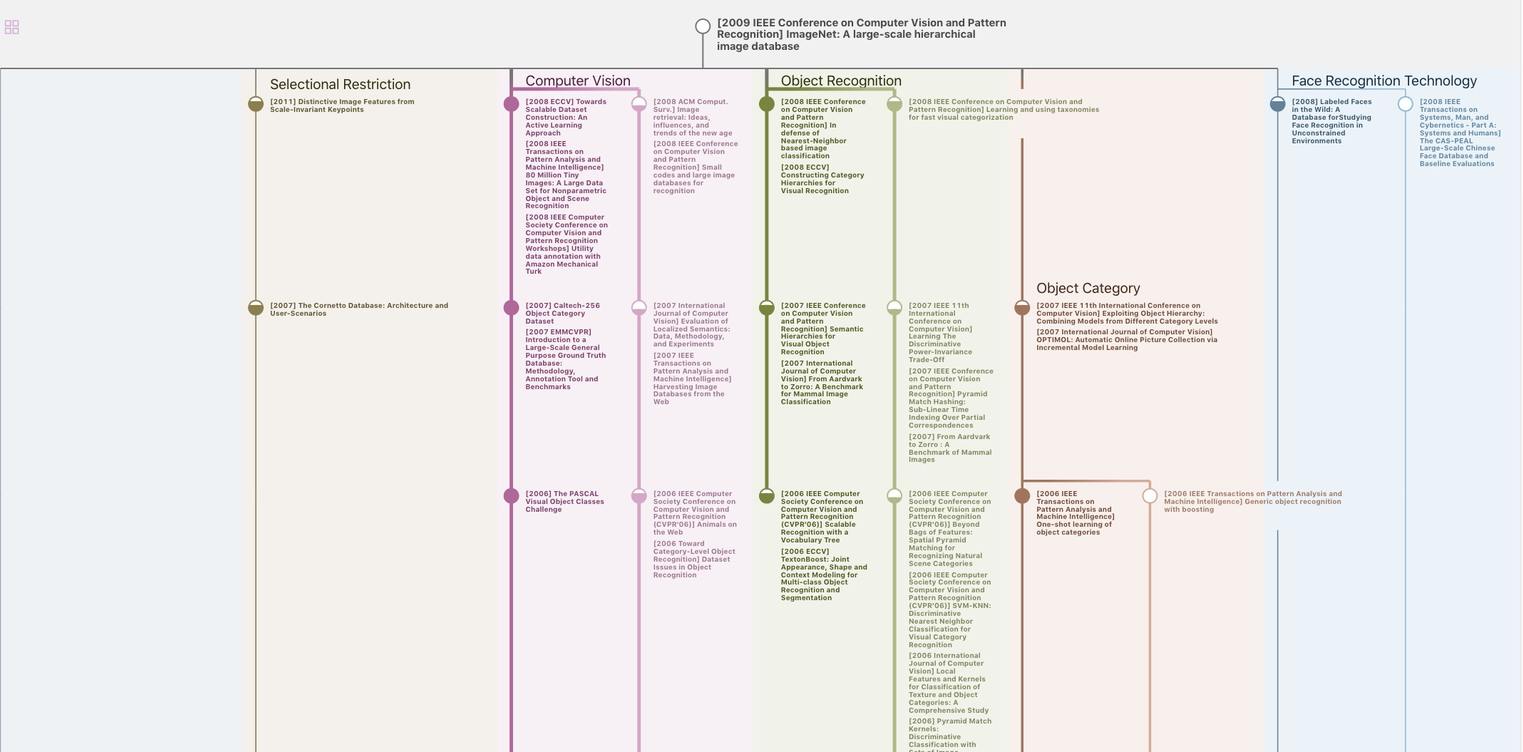
生成溯源树,研究论文发展脉络
Chat Paper
正在生成论文摘要