Learning in time-varying games.
arXiv: Computer Science and Game Theory(2018)
摘要
In this paper, we examine the long-term behavior of regret-minimizing agents in time-varying games with continuous action spaces. In its most basic form, (external) regret minimization guarantees that an agentu0027s cumulative payoff is no worse in the long run than that of the agentu0027s best fixed action in hindsight. Going beyond this worst-case guarantee, we consider a dynamic regret variant that compares the agentu0027s accrued rewards to those of any sequence of play. Specializing to a wide class of no-regret strategies based on mirror descent, we derive explicit rates of regret minimization relying only on imperfect gradient obvservations. We then leverage these results to show that players are able to stay close to Nash equilibrium in time-varying monotone games - and even converge to Nash equilibrium if the sequence of stage games admits a limit.
更多查看译文
AI 理解论文
溯源树
样例
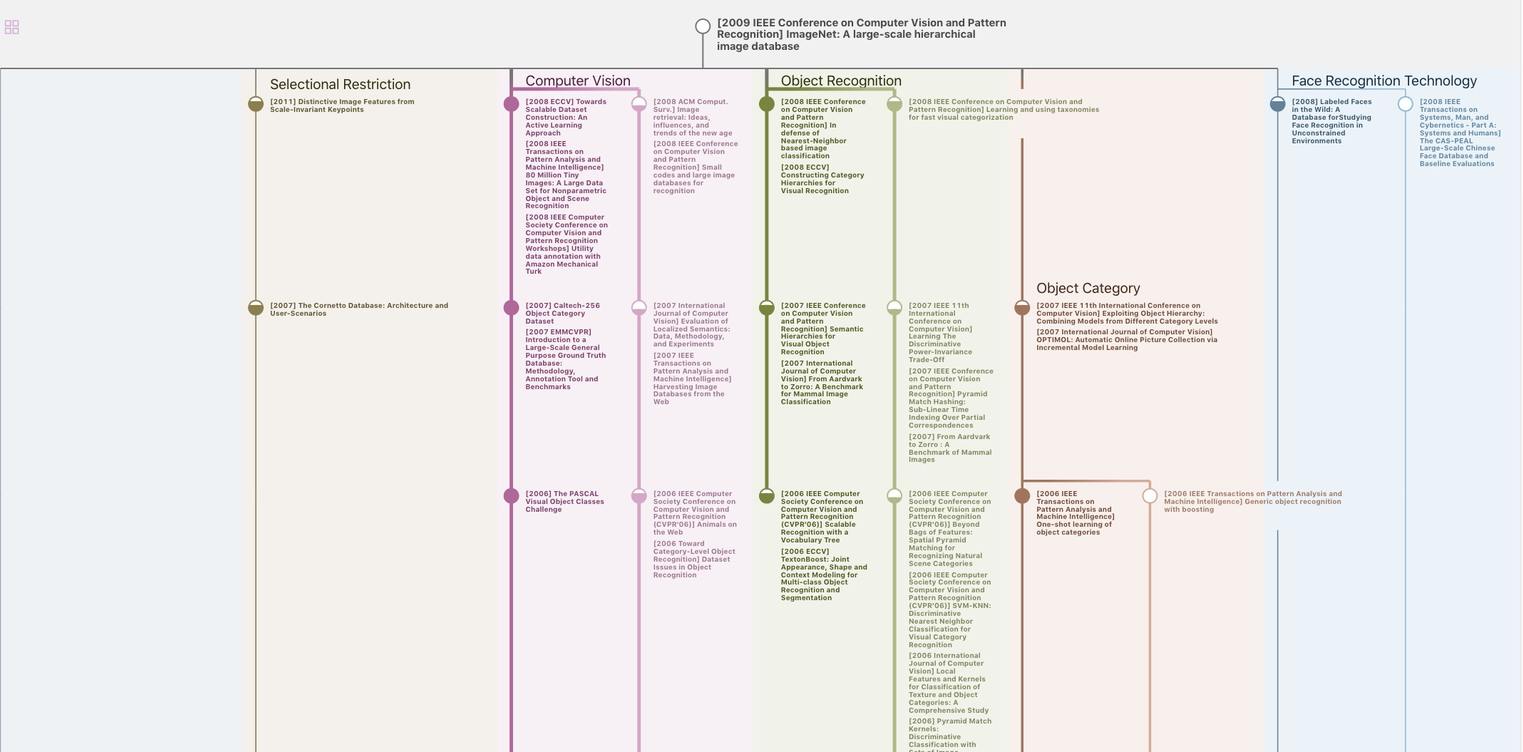
生成溯源树,研究论文发展脉络
Chat Paper
正在生成论文摘要