U-Net for MAV-based Penstock Inspection: an Investigation of Focal Loss in Multi-class Segmentation for Corrosion Identification
arXiv (Cornell University)(2018)
摘要
Periodical inspection and maintenance of critical infrastructure such as dams, penstocks, and locks are of significant importance to prevent catastrophic failures. Conventional manual inspection methods require inspectors to climb along a penstock to spot corrosion, rust and crack formation which is unsafe, labor-intensive, and requires intensive training. This work presents an alternative approach using a Micro Aerial Vehicle (MAV) that autonomously flies to collect imagery which is then fed into a pretrained deep-learning model to identify corrosion. Our simplified U-Net trained with less than 40 image samples can do inference at 12 fps on a single GPU. We analyze different loss functions to solve the class imbalance problem, followed by a discussion on choosing proper metrics and weights for object classes. Results obtained with the dataset collected from Center Hill Dam, TN show that focal loss function, combined with a proper set of class weights yield better segmentation results than the base loss, Softmax cross entropy. Our method can be used in combination with planning algorithm to offer a complete, safe and cost-efficient solution to autonomous infrastructure inspection.
更多查看译文
关键词
penstock inspection,corrosion identification,u-net,mav-based,multi-class
AI 理解论文
溯源树
样例
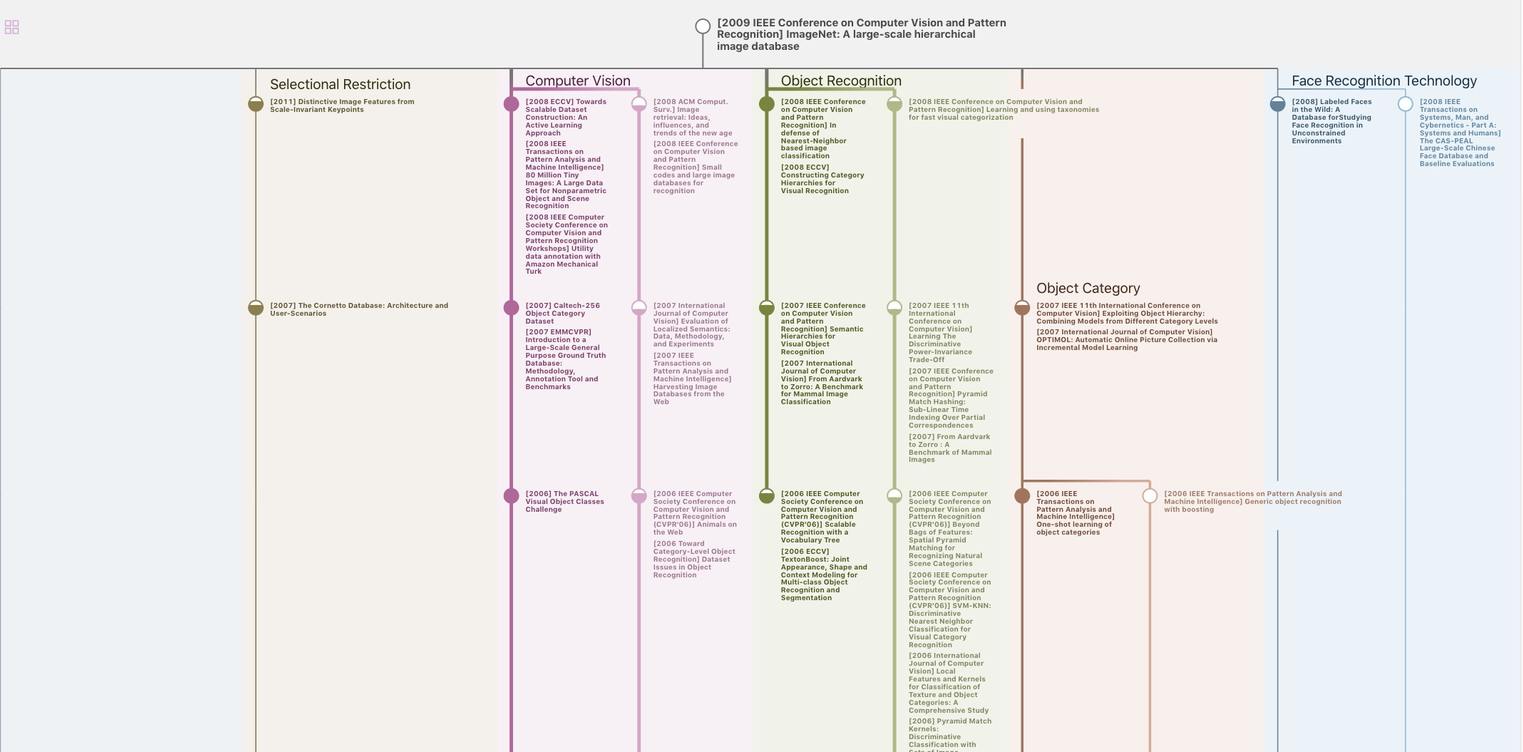
生成溯源树,研究论文发展脉络
Chat Paper
正在生成论文摘要