Fully Supervised Speaker Diarization
ICASSP 2019 - 2019 IEEE International Conference on Acoustics, Speech and Signal Processing (ICASSP)(2019)
摘要
In this paper, we propose a fully supervised speaker diarization approach, named unbounded interleaved-state recurrent neural networks (UIS-RNN). Given extracted speaker-discriminative embeddings (a.k.a. d-vectors) from input utterances, each individual speaker is modeled by a parameter-sharing RNN, while the RNN states for different speakers interleave in the time domain. This RNN is naturally integrated with a distance-dependent Chinese restaurant process (ddCRP) to accommodate an unknown number of speakers. Our system is fully supervised and is able to learn from examples where time-stamped speaker labels are annotated. We achieved a 7.6% diarization error rate on NIST SRE 2000 CALLHOME, which is better than the state-of-the-art method using spectral clustering. Moreover, our method decodes in an online fashion while most state-of-the-art systems rely on offline clustering.
更多查看译文
关键词
Speaker diarization,d-vector,clustering,recurrent neural networks,Chinese restaurant process
AI 理解论文
溯源树
样例
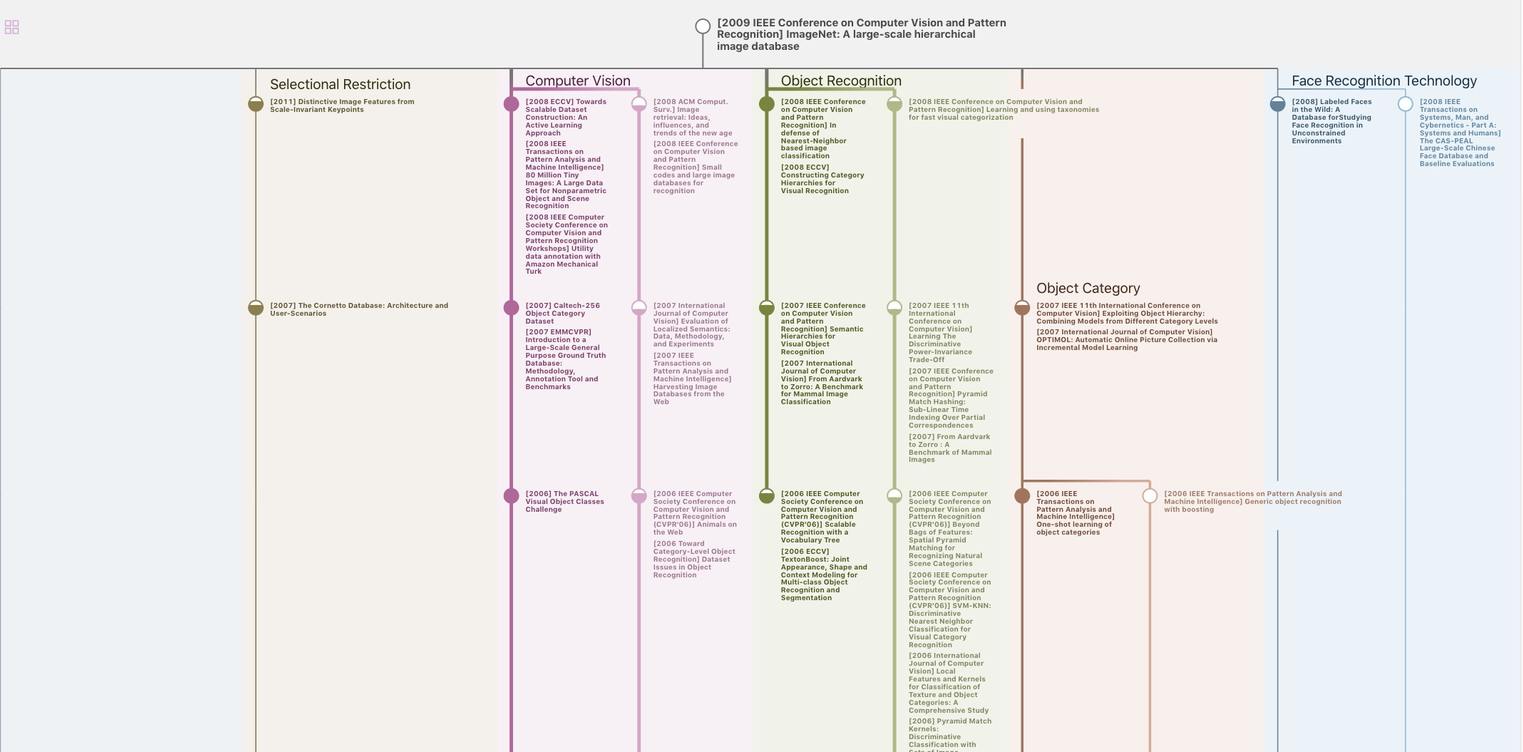
生成溯源树,研究论文发展脉络
Chat Paper
正在生成论文摘要