Fast construction of correcting ensembles for legacy Artificial Intelligence systems
Information Sciences: an International Journal(2019)
摘要
This paper presents a new approach for constructing simple and computationally efficient improvements of generic Artificial Intelligence (AI) systems, including Multilayer and Deep Learning neural networks. The improvements are small network ensembles added to the existing AI architectures. Theoretical foundations of the approach are based on stochastic separation theorems and the ideas of the concentration of measure. We show that, subject to mild technical assumptions on statistical properties of internal signals in the original AI system, the approach enables fast removal of the AI’s errors with probability close to one on the datasets which may be exponentially large in dimension. The approach is illustrated with numerical examples and a case study of digits recognition in American Sign Language.
更多查看译文
关键词
Neural ensembles,Stochastic separation theorems,Perceptron,Artificial intelligence,Measure concentration
AI 理解论文
溯源树
样例
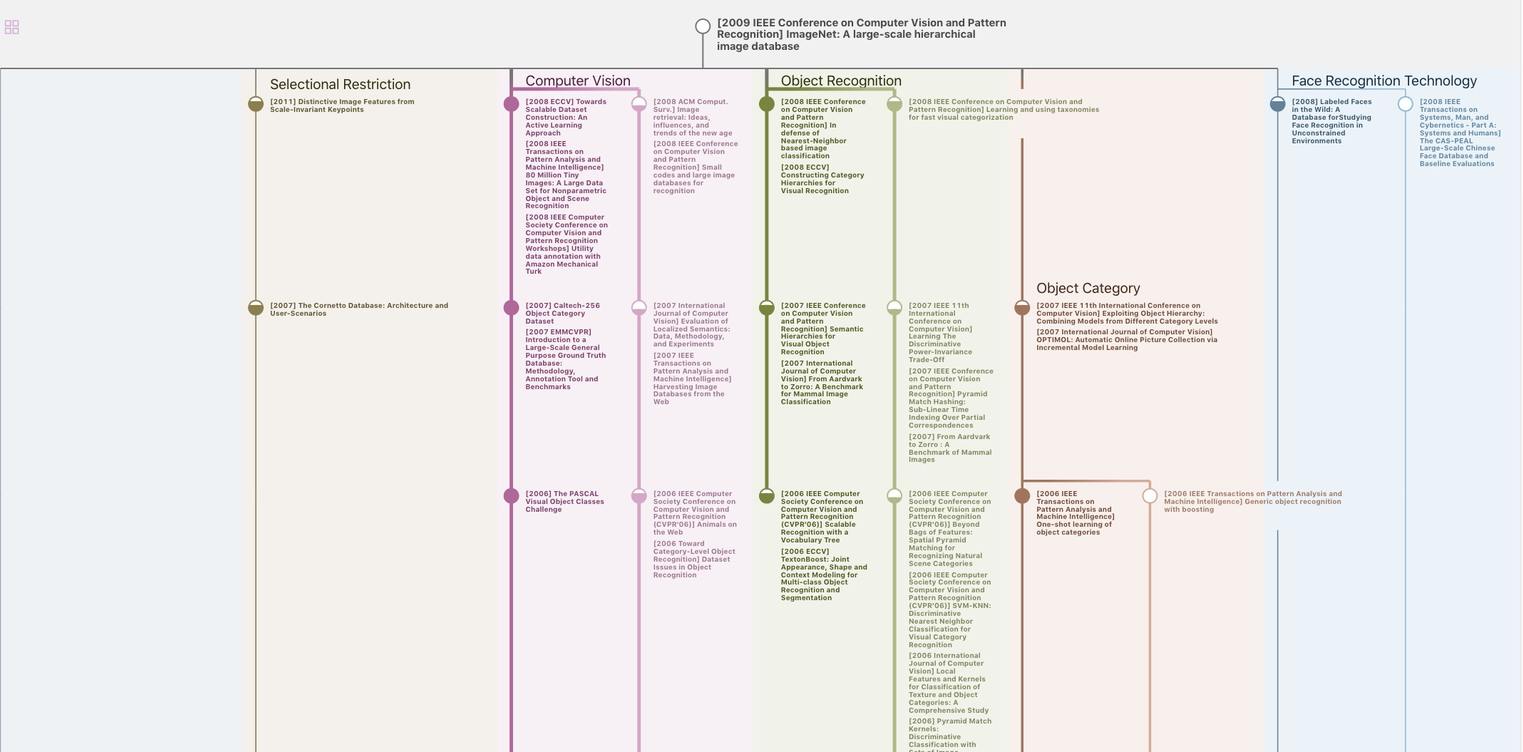
生成溯源树,研究论文发展脉络
Chat Paper
正在生成论文摘要