Jobs: Joint-Sparse Optimization From Bootstrap Samples
2019 IEEE International Symposium on Information Theory (ISIT)(2019)
摘要
Classical sparse regression based on l(1) minimization solves the least squares problem with all available measurements via sparsity-promoting regularization. In challenging practical applications with high levels of noise and missing or adversarial samples, solving the problem using all measurements simultaneously may fail. In this paper, we propose a robust global sparse recovery strategy, named JOBS, which uses bootstrap samples of measurements to improve sparse regression in difficult cases. K measurement vectors are generated from the original pool of m measurements via bootstrapping, with each bootstrap sample containing L elements, and then a joint-sparse constraint is enforced to ensure support consistency among multiple predictors. The final estimate is obtained by averaging over K estimators.The performance limits associated with finite bootstrap sampling ratio L/m and number of estimates K is analyzed theoretically. Simulation results validate the theoretical analysis of proper choice of (L, K) and show that the proposed method yields state-of-the-art recovery performance, outperforming l(1) minimization and other existing bootstrap-based techniques, especially when the number of measurements are limited. With a proper choice of bootstrap sampling ratio (0.3 - 0.5) and a reasonably large number of estimates K (>= 30), the SNR improvement over the baseline l(1)-minimization algorithm can reach up to 336%
更多查看译文
关键词
measurement vectors,least squares problem,joint-sparse optimization from bootstrap samples,JOBS,ℓ1 minimization,state-of-the-art recovery performance,SNR improvement,sparsity-promoting regularization,classical sparse regression,finite bootstrap sampling ratio,joint-sparse constraint,robust global sparse recovery strategy
AI 理解论文
溯源树
样例
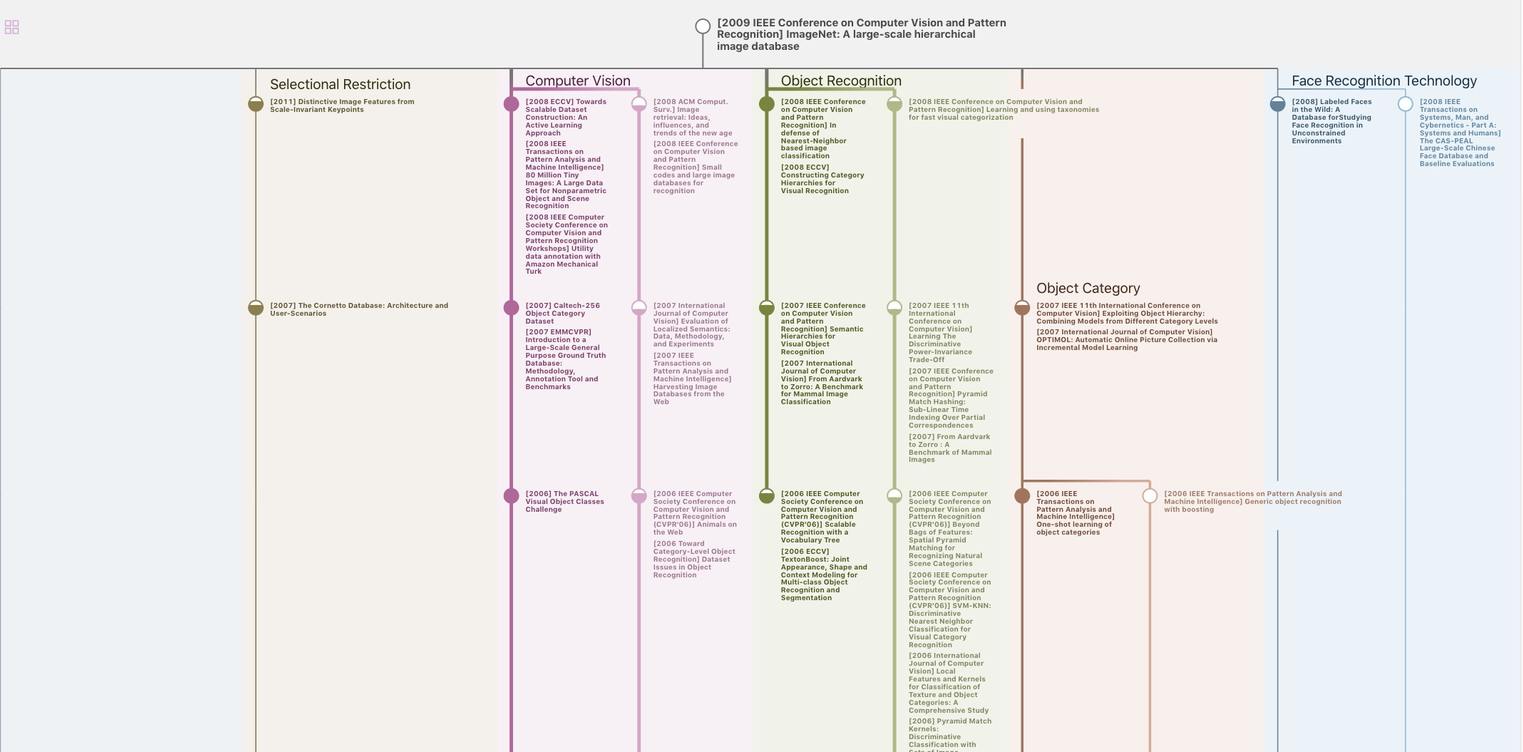
生成溯源树,研究论文发展脉络
Chat Paper
正在生成论文摘要