Exploration vs. Exploitation in Team Formation.
arXiv: Learning(2018)
摘要
An online labor platform faces an online learning problem in matching workers with jobs and using the performance on these jobs to create better future matches. This learning problem is complicated by the rise of complex tasks on these platforms, such as web development and product design, that require a team of workers to complete. The success of a job is now a function of the skills and contributions of all workers involved, which may be unknown to both the platform and the client who posted the job. These team matchings result in a structured correlation between what is known about the individuals and this information can be utilized to create better future matches. We analyze two natural settings where the performance of a team is dictated by its strongest and its weakest member, respectively. We find that both problems pose an exploration-exploitation tradeoff between learning the performance of untested teams and repeating previously tested teams that resulted in a good performance. We establish fundamental regret bounds and design near-optimal algorithms that uncover several insights into these tradeoffs.
更多查看译文
关键词
Team formation, Online learning, Online labor platforms
AI 理解论文
溯源树
样例
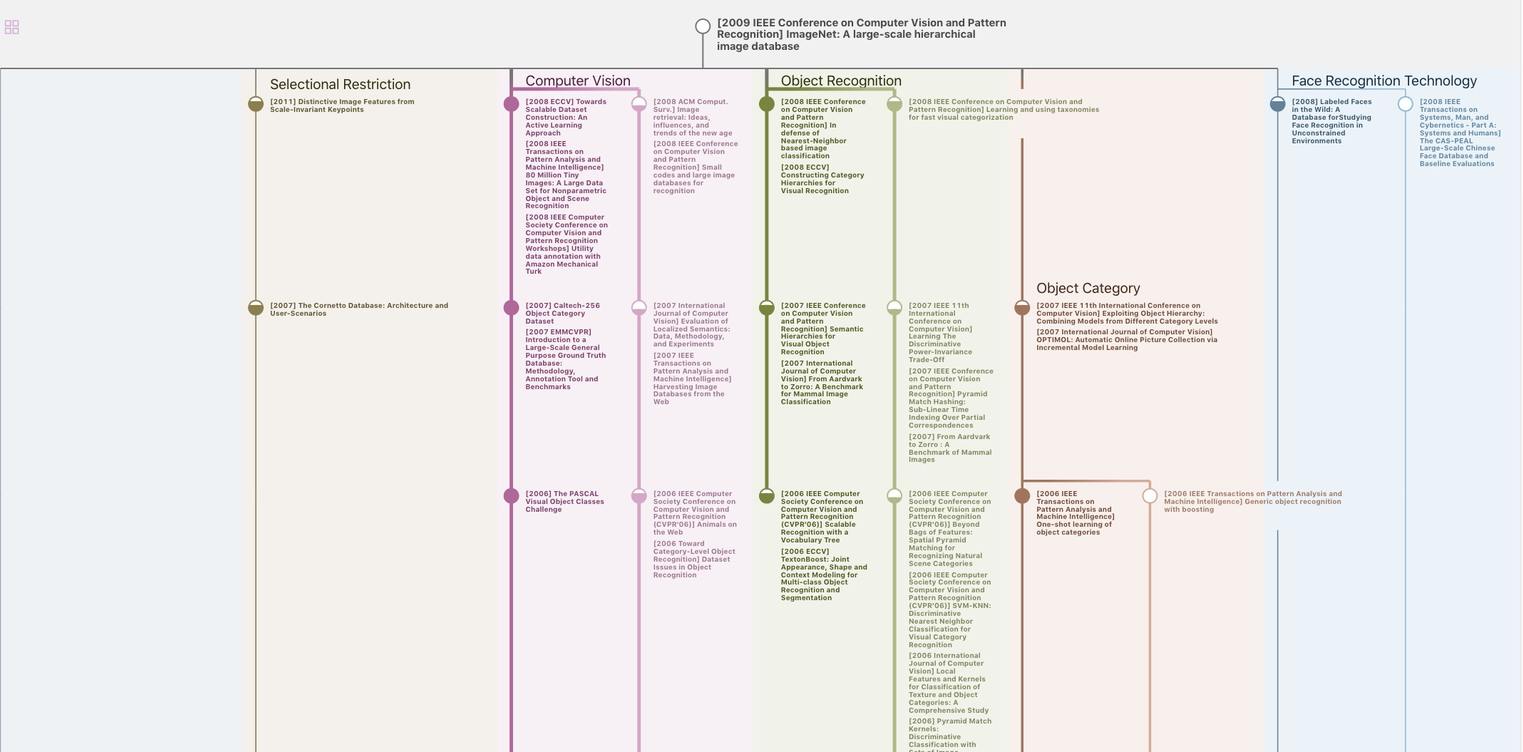
生成溯源树,研究论文发展脉络
Chat Paper
正在生成论文摘要