ChainQueen: A Real-Time Differentiable Physical Simulator for Soft Robotics
2019 International Conference on Robotics and Automation (ICRA)(2018)
摘要
Physical simulators have been widely used in robot planning and control. Among them, differentiable simulators are particularly favored, as they can be incorporated into gradient-based optimization algorithms that are efficient in solving inverse problems such as optimal control and motion planning. Simulating deformable objects is, however, more challenging compared to rigid body dynamics. The underlying physical laws of deformable objects are more complex, and the resulting systems have orders of magnitude more degrees of freedom and therefore they are significantly more computationally expensive to simulate. Computing gradients with respect to physical design or controller parameters is typically even more computationally challenging. In this paper, we propose a real-time, differentiable hybrid Lagrangian-Eulerian physical simulator for deformable objects, ChainQueen, based on the Moving Least Squares Material Point Method (MLS-MPM). MLS-MPM can simulate deformable objects including contact and can be seamlessly incorporated into inference, control and co-design systems. We demonstrate that our simulator achieves high precision in both forward simulation and backward gradient computation. We have successfully employed it in a diverse set of control tasks for soft robots, including problems with nearly 3,000 decision variables.
更多查看译文
关键词
ChainQueen,real-time differentiable physical simulator,robot planning,gradient-based optimization algorithms,inverse problems,optimal control,motion planning,rigid body simulators,deformable objects,rigid body dynamics,Lagrangian-Eulerian physical simulator,MLS-MPM,soft robotic systems,forward simulation,backward gradient computation,moving least squares material point method
AI 理解论文
溯源树
样例
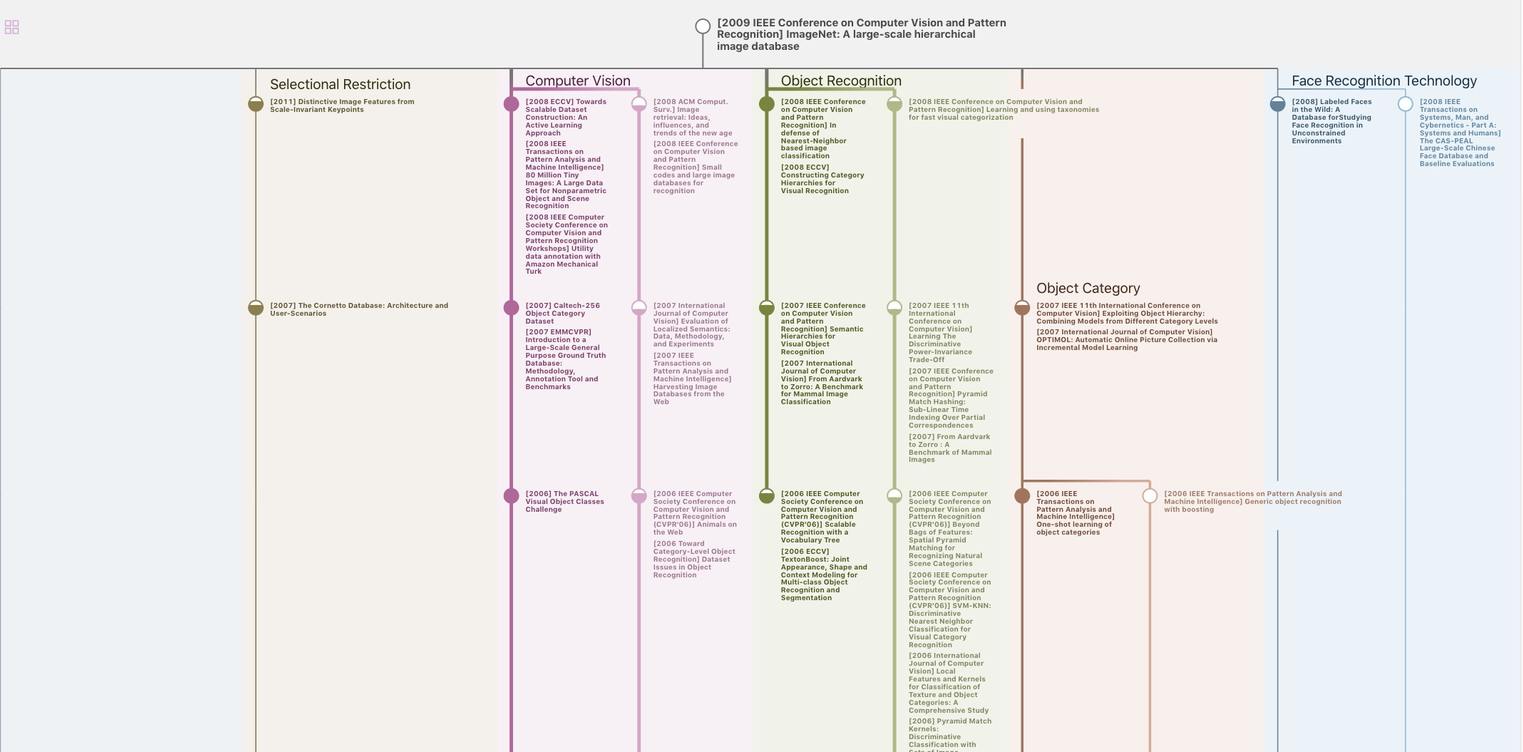
生成溯源树,研究论文发展脉络
Chat Paper
正在生成论文摘要