Approximation algorithms for stochastic clustering.
ADVANCES IN NEURAL INFORMATION PROCESSING SYSTEMS 31 (NIPS 2018)(2018)
摘要
We consider stochastic settings for clustering, and develop provably-good (approximation) algorithms for a number of these notions. These algorithms allow one to obtain better approximation ratios compared to the usual deterministic clustering setting. Additionally, they offer a number of advantages including providing fairer clustering and clustering which has better long-term behavior for each user. In particular, they ensure that every user is guaranteed to get good service (on average). We also complement some of these with impossibility results.
更多查看译文
关键词
clustering,k-center,k-median,lottery,approximation algorithms
AI 理解论文
溯源树
样例
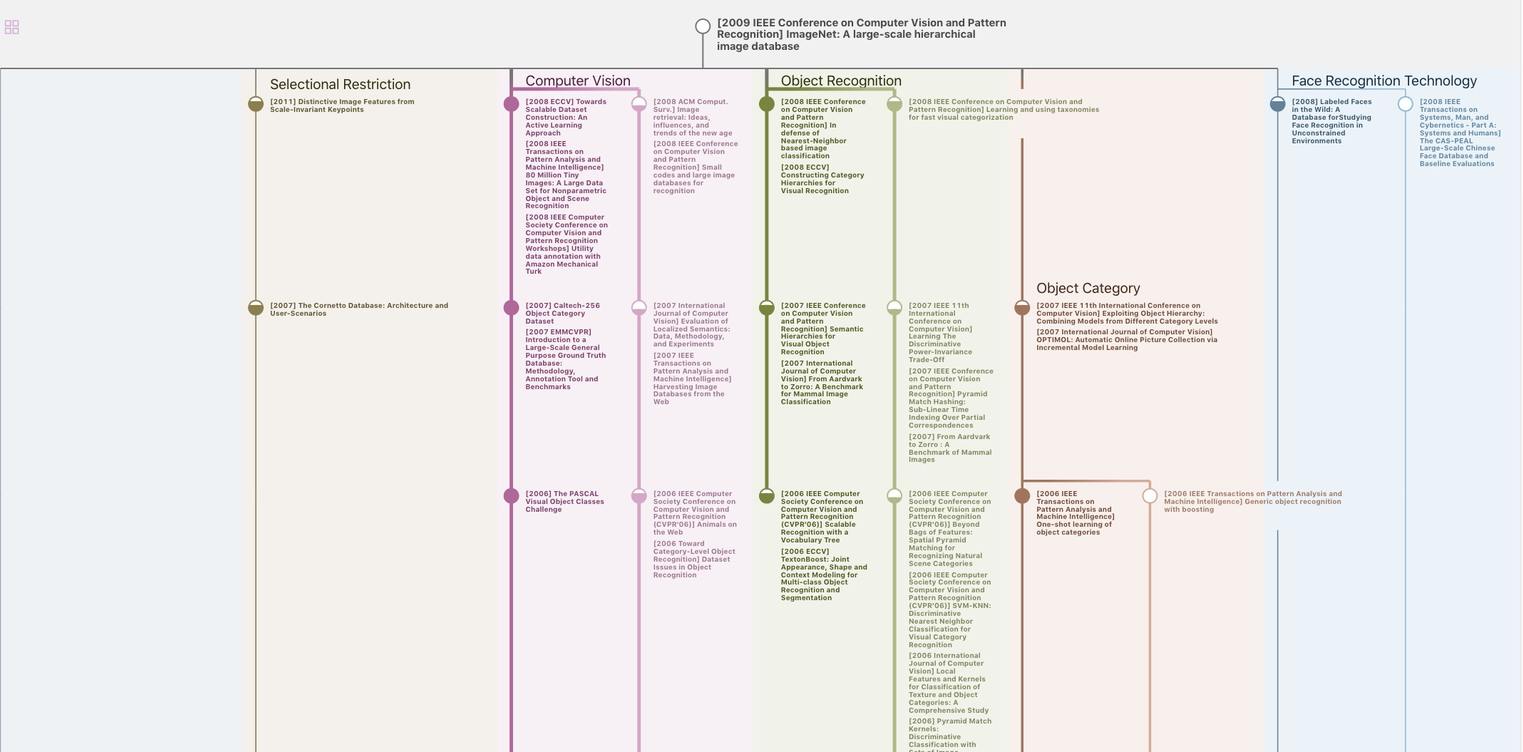
生成溯源树,研究论文发展脉络
Chat Paper
正在生成论文摘要