Flow Motifs in Interaction Networks
EDBT(2018)
摘要
Many real-world phenomena are best represented as interaction networks with dynamic structures (e.g., transaction networks, social networks, traffic networks). Interaction networks capture flow of data which is transferred between their vertices along a timeline. Analyzing such networks is crucial toward comprehend- ing processes in them. A typical analysis task is the finding of motifs, which are small subgraph patterns that repeat themselves in the network. In this paper, we introduce network flow motifs, a novel type of motifs that model significant flow transfer among a set of vertices within a constrained time window. We design an algorithm for identifying flow motif instances in a large graph. Our algorithm can be easily adapted to find the top-k instances of maximal flow. In addition, we design a dynamic programming module that finds the instance with the maximum flow. We evaluate the performance of the algorithm on three real datasets and identify flow motifs which are significant for these graphs. Our results show that our algorithm is scalable and that the real networks indeed include interesting motifs, which appear much more frequently than in randomly generated networks having similar characteristics.
更多查看译文
AI 理解论文
溯源树
样例
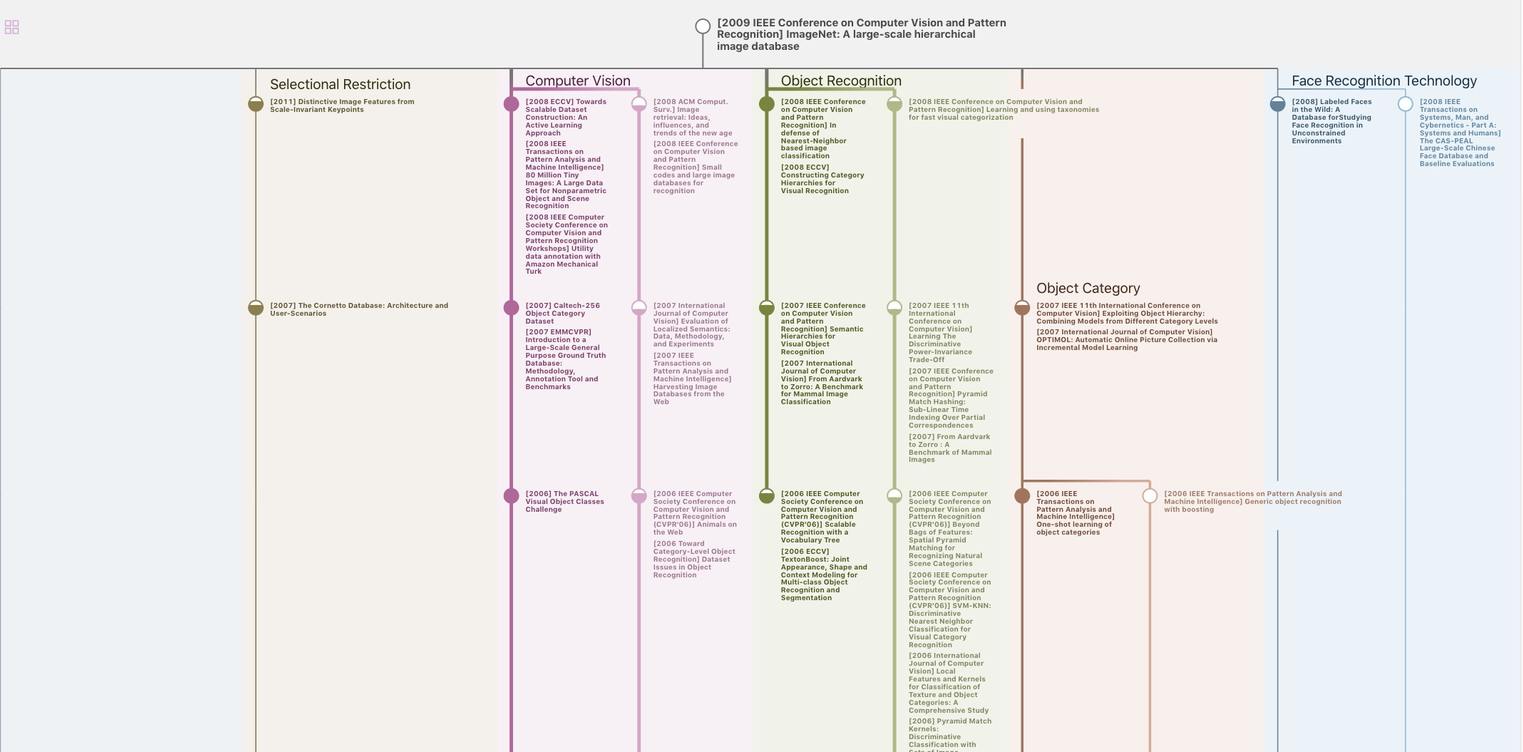
生成溯源树,研究论文发展脉络
Chat Paper
正在生成论文摘要