Efficient Statistics, in High Dimensions, from Truncated Samples
2018 IEEE 59th Annual Symposium on Foundations of Computer Science (FOCS)(2018)
摘要
We provide an efficient algorithm for the classical problem, going back to Galton, Pearson, and Fisher, of estimating, with arbitrary accuracy the parameters of a multivariate normal distribution from truncated samples. Truncated samples from a d-variate normal N(mu, Sigma) means a samples is only revealed if it falls in some subset S of the d-dimensional Euclidean space; otherwise the samples are hidden and their count in proportion to the revealed samples is also hidden. We show that the mean mu and covariance matrix Sigma can be estimated with arbitrary accuracy in polynomial-time, as long as we have oracle access to S, and S has non-trivial measure under the unknown d-variate normal distribution. Additionally we show that without oracle access to S, any non-trivial estimation is impossible.
更多查看译文
关键词
efficient statistics,truncated samples,normal distribution,high dimensions,stochastic gradient descent
AI 理解论文
溯源树
样例
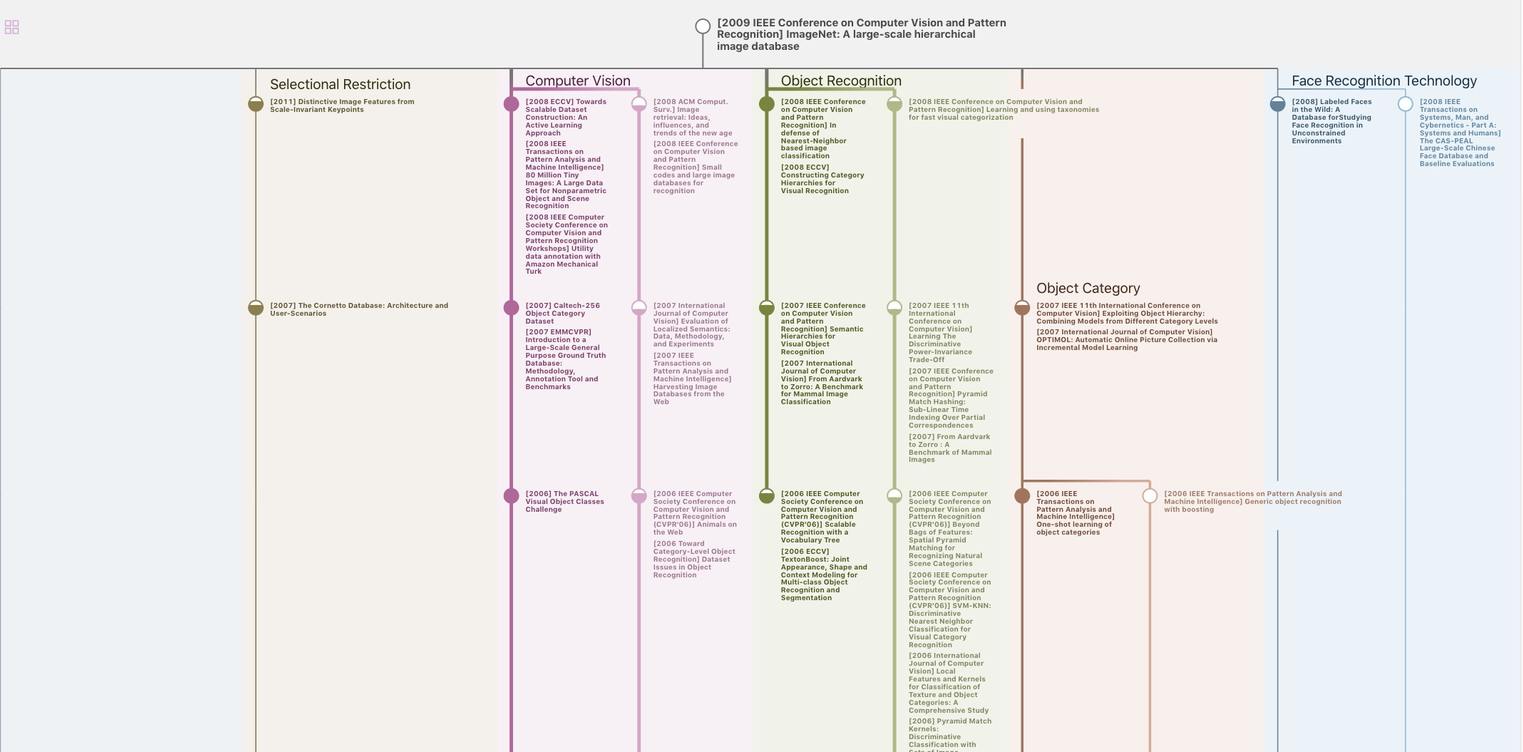
生成溯源树,研究论文发展脉络
Chat Paper
正在生成论文摘要