Reinforcement Learning Decoders For Fault-Tolerant Quantum Computation
MACHINE LEARNING-SCIENCE AND TECHNOLOGY(2021)
摘要
Topological error correcting codes, and particularly the surface code, currently provide the most feasible road-map towards large-scale fault-tolerant quantum computation. As such, obtaining fast and flexible decoding algorithms for these codes, within the experimentally realistic and challenging context of faulty syndrome measurements, without requiring any final read-out of the physical qubits, is of critical importance. In this work, we show that the problem of decoding such codes can be naturally reformulated as a process of repeated interactions between a decoding agent and a code environment, to which the machinery of reinforcement learning can be applied to obtain decoding agents. While in principle this framework can be instantiated with environments modelling circuit level noise, we take a first step towards this goal by using deepQ learning to obtain decoding agents for a variety of simplified phenomenological noise models, which yield faulty syndrome measurements without including the propagation of errors which arise in full circuit level noise models.
更多查看译文
关键词
quantum error correction, reinforcement learning, fault tolerant quantum computing
AI 理解论文
溯源树
样例
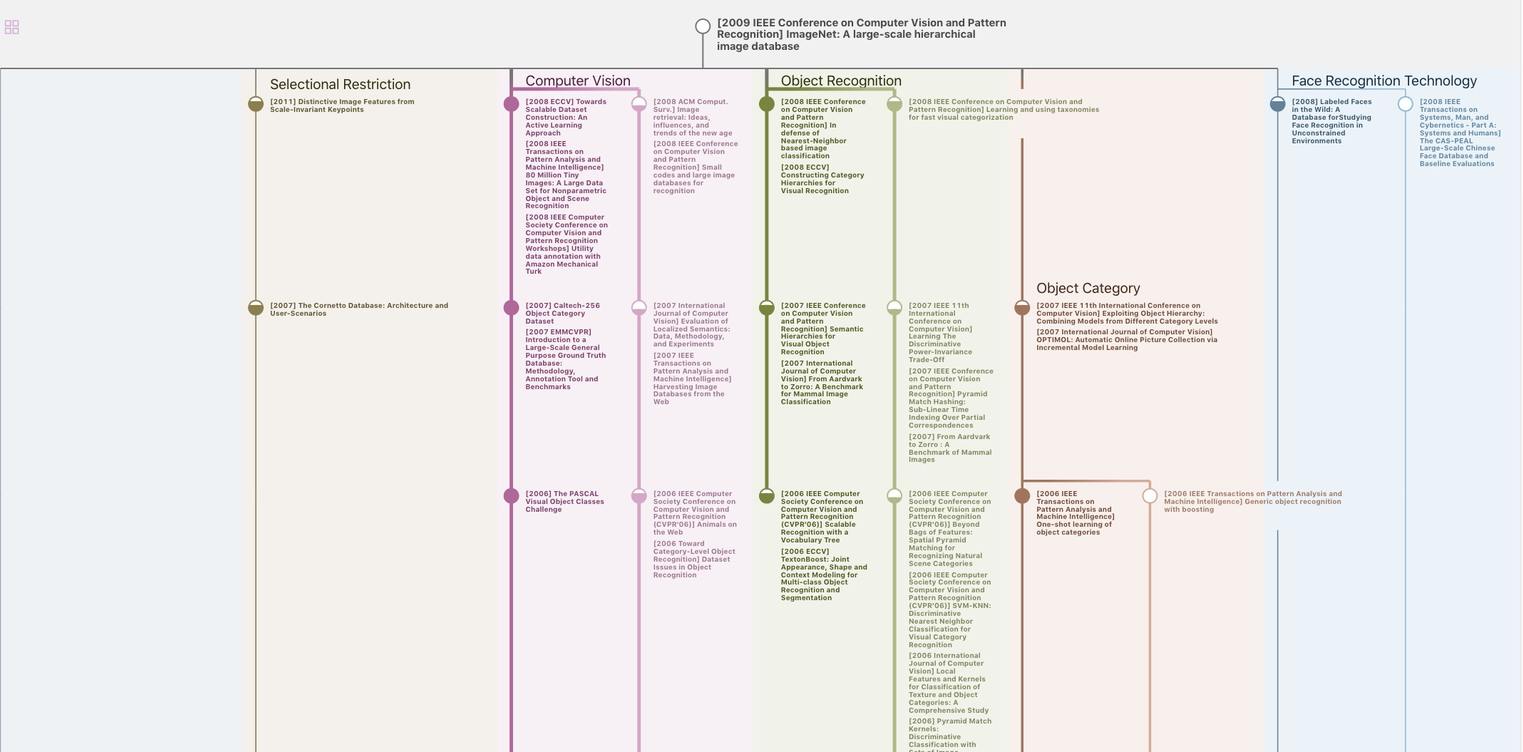
生成溯源树,研究论文发展脉络
Chat Paper
正在生成论文摘要