Towards Good Practices for Multi-modal Fusion in Large-scale Video Classification
Computer Vision – ECCV 2018 Workshops: Munich, Germany, September 8-14, 2018, Proceedings, Part IV(2018)
摘要
Leveraging both visual frames and audio has been experimentally proven effective to improve large-scale video classification. Previous research on video classification mainly focuses on the analysis of visual content among extracted video frames and their temporal feature aggregation. In contrast, multimodal data fusion is achieved by simple operators like average and concatenation. Inspired by the success of bilinear pooling in the visual and language fusion, we introduce multi-modal factorized bilinear pooling (MFB) to fuse visual and audio representations. We combine MFB with different video-level features and explore its effectiveness in video classification. Experimental results on the challenging Youtube-8M v2 dataset demonstrate that MFB significantly outperforms simple fusion methods in large-scale video classification.
更多查看译文
关键词
Video classification,Multi-modal learning,Bilinear model
AI 理解论文
溯源树
样例
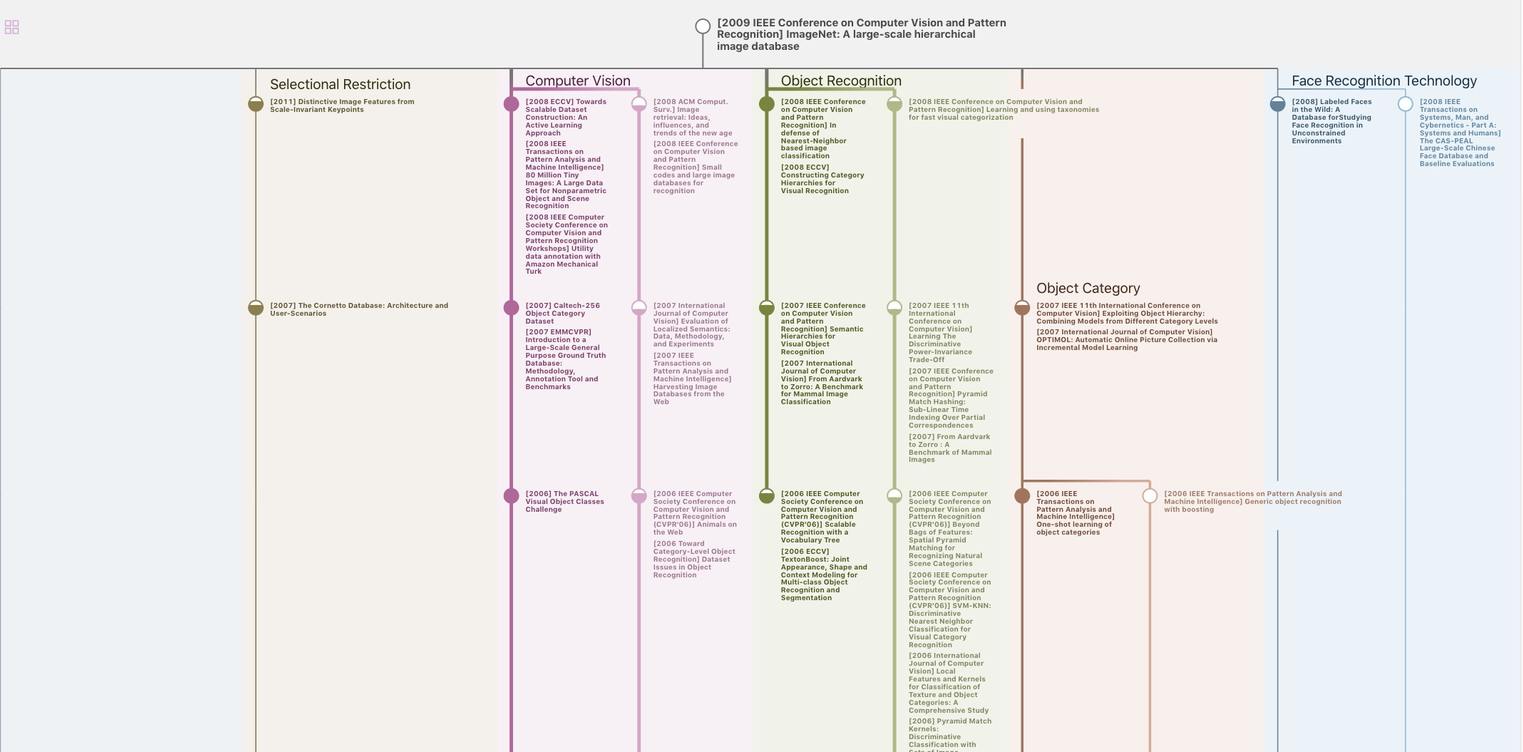
生成溯源树,研究论文发展脉络
Chat Paper
正在生成论文摘要