A machine learning approach for feature selection traffic classification using security analysis
The Journal of Supercomputing(2018)
摘要
Class imbalance has become a big problem that leads to inaccurate traffic classification. Accurate traffic classification of traffic flows helps us in security monitoring, IP management, intrusion detection, etc. To address the traffic classification problem, in literature, machine learning (ML) approaches are widely used. Therefore, in this paper, we also proposed an ML-based hybrid feature selection algorithm named WMI_AUC that make use of two metrics: weighted mutual information (WMI) metric and area under ROC curve (AUC). These metrics select effective features from a traffic flow. However, in order to select robust features from the selected features, we proposed robust features selection algorithm. The proposed approach increases the accuracy of ML classifiers and helps in detecting malicious traffic. We evaluate our work using 11 well-known ML classifiers on the different network environment traces datasets. Experimental results showed that our algorithms achieve more than 95% flow accuracy results.
更多查看译文
关键词
Network traffic classification,Class imbalance,Feature selection,Machine learning,Security
AI 理解论文
溯源树
样例
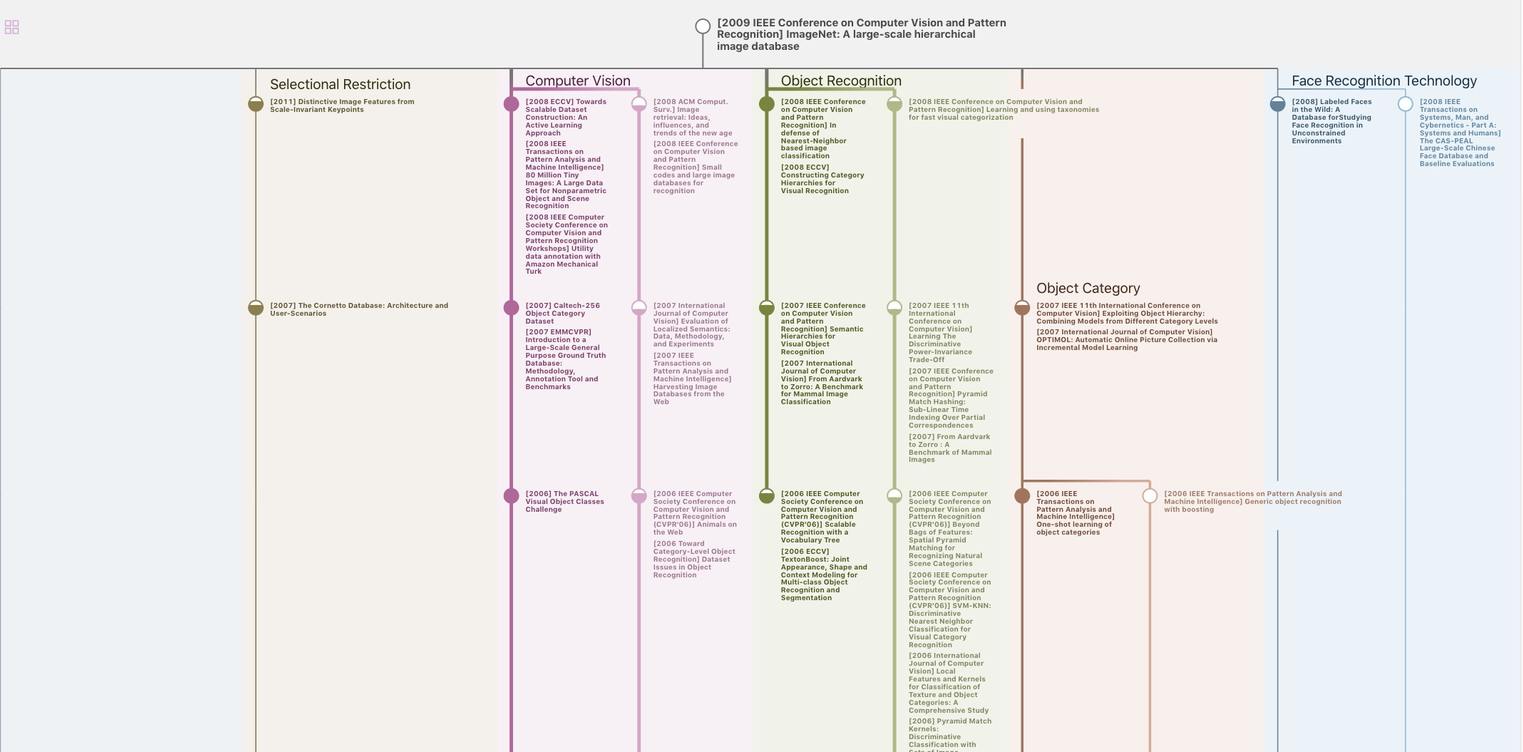
生成溯源树,研究论文发展脉络
Chat Paper
正在生成论文摘要