Image Segmentation by Deep Learning of Disjunctive Normal Shape Model Shape Representation.
IEEE Computer Society Conference on Computer Vision and Pattern Recognition Workshops(2018)
摘要
Segmenting images with low-quality, low signal to noise ratio has been a challenging task in computer vision. It has been shown that statistical prior information about the shape of the object to be segmented can be used to significantly mitigate this problem. However estimating the probability densities of the object shapes in the space of shapes can be difficult. This problem becomes more difficult when there is limited amount of training data or the testing images contain missing data. Most shape model based segmentation approaches tend to minimize an energy functional to segment the object. In this paper we propose a shape-based segmentation algorithm that utilizes convolutional neural networks to learn a posterior distribution of disjunction of conjunctions of half spaces to segment the object. This approach shows promising results on noisy and occluded data where it is able to accurately segment the objects. We show visual and quantitative results on datasets from several applications, demonstrating the effectiveness of the proposed approach. We should also note that inference with a CNN is computationally more efficient than density estimation and sampling approaches.
更多查看译文
关键词
computer vision,statistical prior information,object shapes,segmentation approaches,shape-based segmentation algorithm,density estimation,sampling approaches,image segmentation,deep learning,disjunctive normal shape model shape representation,convolutional neural network,posterior distribution
AI 理解论文
溯源树
样例
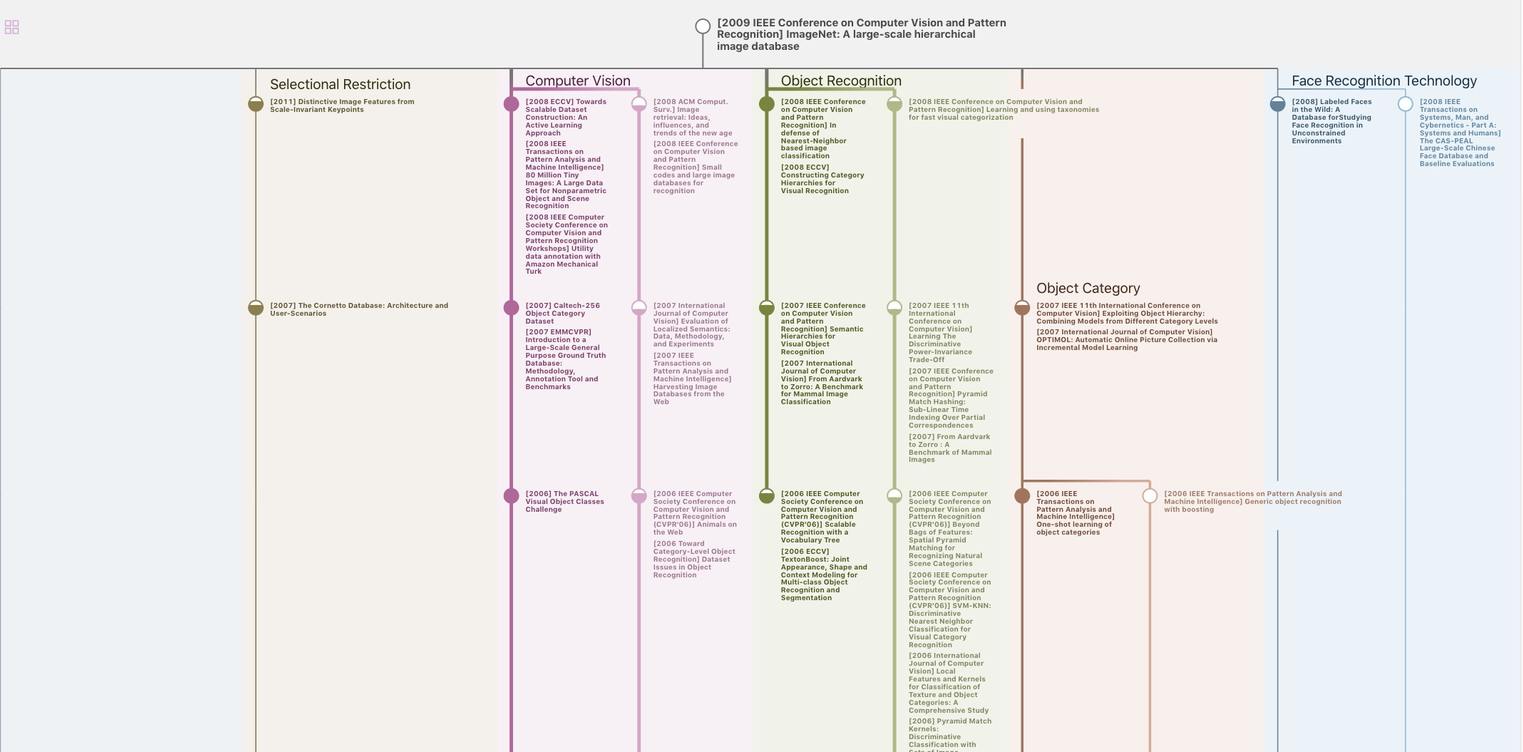
生成溯源树,研究论文发展脉络
Chat Paper
正在生成论文摘要