Geometry Guided Convolutional Neural Networks For Self-Supervised Video Representation Learning
2018 IEEE/CVF CONFERENCE ON COMPUTER VISION AND PATTERN RECOGNITION (CVPR)(2018)
摘要
It is often laborious and costly to manually annotate videos for training high-quality video recognition models, so there has been some work and interest in exploring alternative, cheap, and yet often noisy and indirect training signals for learning the video representations. However, these signals are still coarse, supplying supervision at the whole video frame level, and subtle, sometimes enforcing the learning agent to solve problems that are even hard for humans. In this paper, we instead explore geometry, a grand new type of auxiliary supervision for the self-supervised learning of video representations. In particular, we extract pixel-wise geometry information as flow fields and disparity maps from synthetic imagery and real 3D movies, respectively. Although the geometry and high-level semantics are seemingly distant topics, surprisingly, we find that the convolutional neural networks pre-trained by the geometry cues can be effectively adapted to semantic video understanding tasks. In addition, we also find that a progressive training strategy can foster a better neural network for the video recognition task than blindly pooling the distinct sources of geometry cues together. Extensive results on video dynamic scene recognition and action recognition tasks show that our geometry guided networks significantly outperform the competing methods that are trained with other types of labeling-free supervision signals.
更多查看译文
关键词
geometry guided convolutional neural networks,self-supervised video representation learning,high-quality video recognition models,noisy training signals,video representations,video frame level,learning agent,self-supervised learning,pixel-wise geometry information,geometry cues,semantic video understanding tasks,video dynamic scene recognition,action recognition tasks
AI 理解论文
溯源树
样例
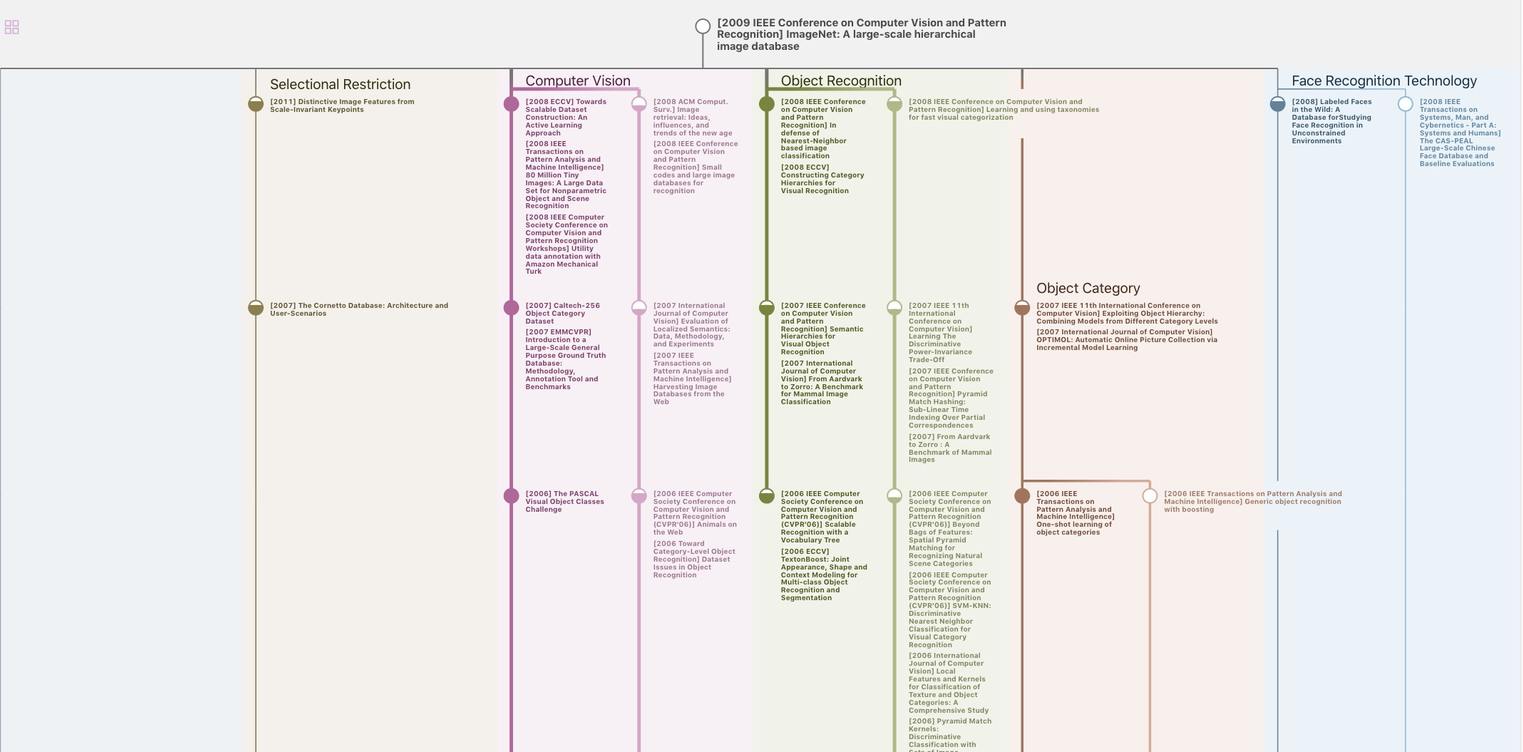
生成溯源树,研究论文发展脉络
Chat Paper
正在生成论文摘要