Cvm-Net: Cross-View Matching Network For Image-Based Ground-To-Aerial Geo-Localization
2018 IEEE/CVF CONFERENCE ON COMPUTER VISION AND PATTERN RECOGNITION (CVPR)(2018)
摘要
The problem of localization on a geo-referenced aerial/satellite map given a query ground view image remains challenging due to the drastic change in viewpoint that causes traditional image descriptors based matching to fail. We leverage on the recent success of deep learning to propose the CVM-Net for the cross-view image-based ground-to-aerial geo-localization task. Specifically, our network is based on the Siamese architecture to do metric learning for the matching task. We first use the fully convolutional layers to extract local image features, which are then encoded into global image descriptors using the powerful NetVLAD. As part of the training procedure, we also introduce a simple yet effective weighted soft margin ranking loss function that not only speeds up the training convergence but also improves the final matching accuracy. Experimental results show that our proposed network significantly outperforms the state-of-the-art approaches on two existing benchmarking datasets. Our code and models are publicly available on the project website(1).
更多查看译文
关键词
CVM-Net,cross-view matching network,geo-referenced aerial/satellite map,query ground view image,cross-view image-based ground-to-aerial geo-localization task,matching task,local image features,global image descriptors,matching accuracy,Siamese architecture
AI 理解论文
溯源树
样例
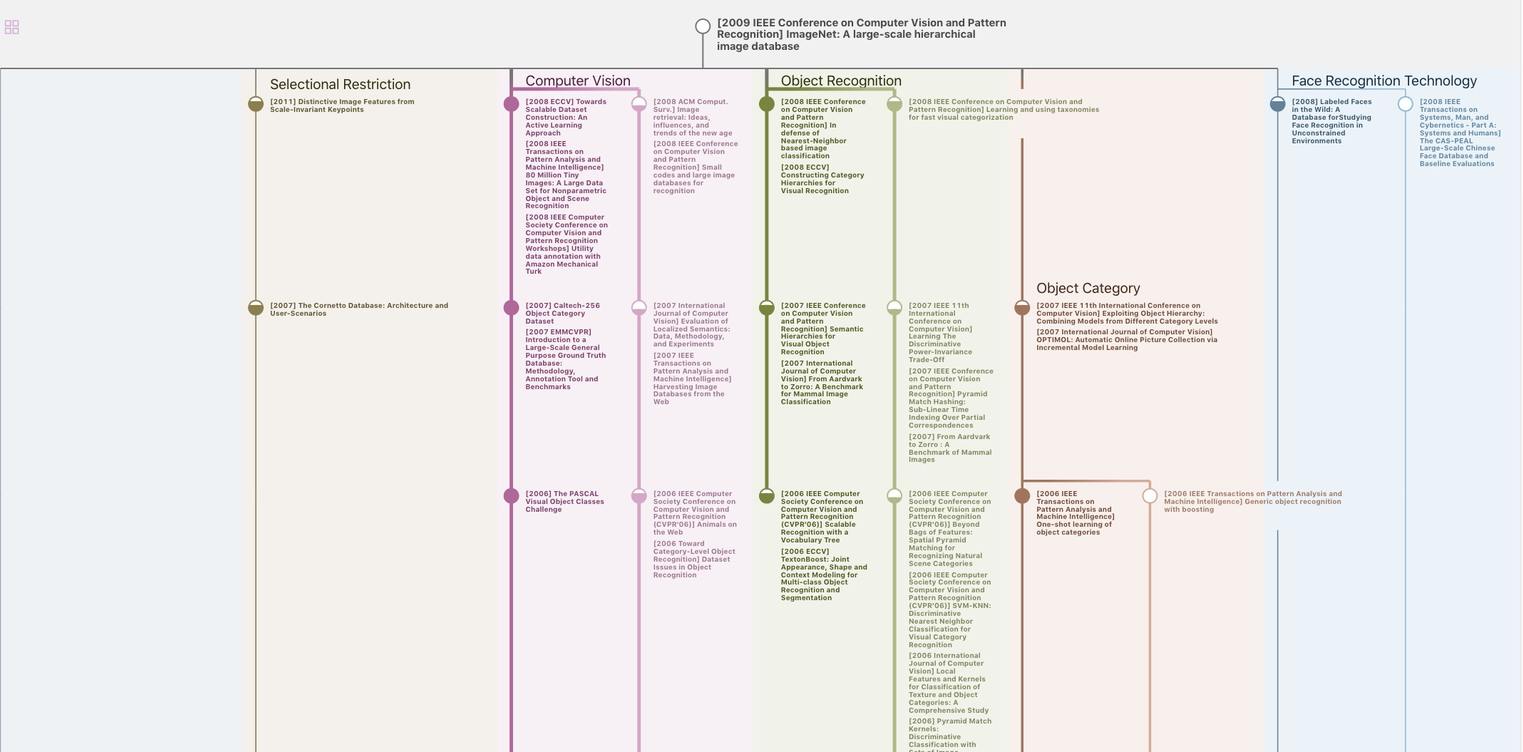
生成溯源树,研究论文发展脉络
Chat Paper
正在生成论文摘要